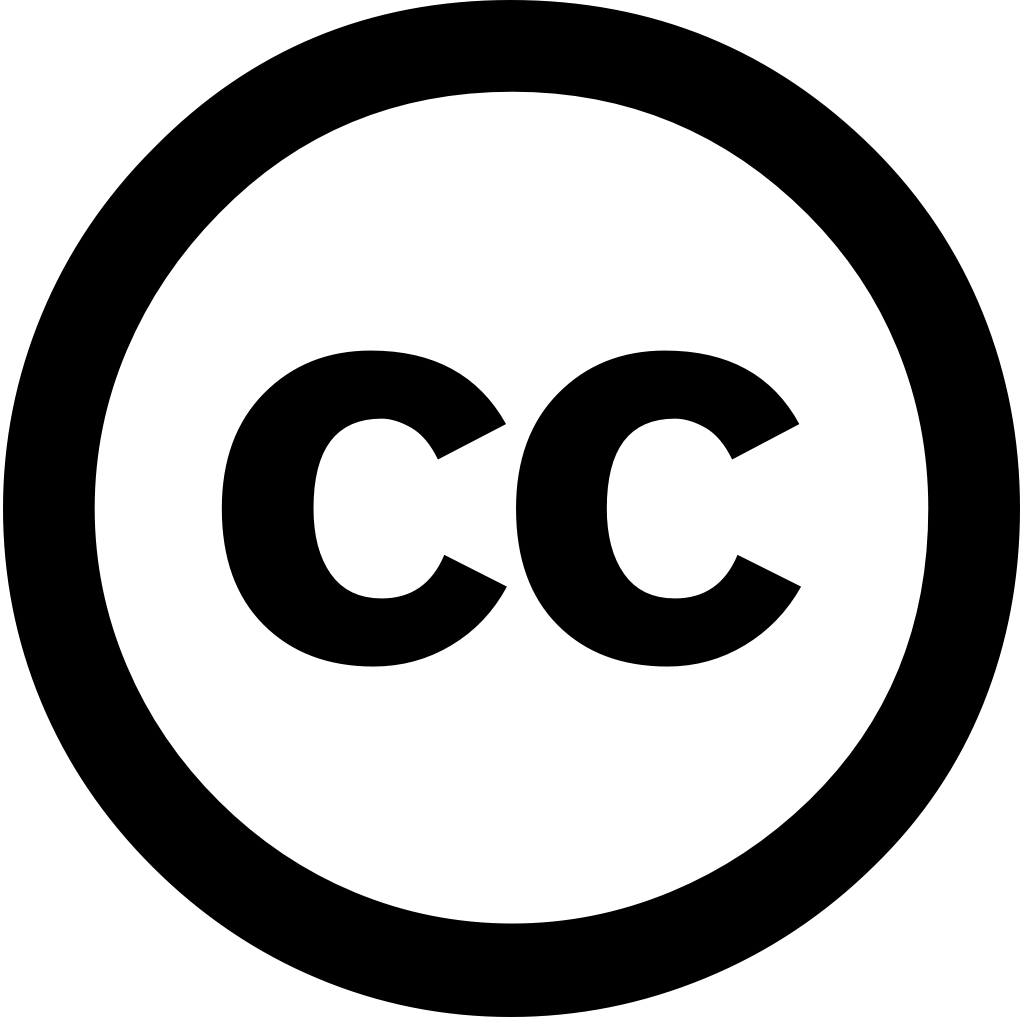
Journal of Marine Science and Engineering, Journal Year: 2025, Volume and Issue: 13(4), P. 746 - 746
Published: April 8, 2025
Autonomous vessels are becoming paramount to ocean transportation, while they also face complex risks in dynamic marine environments. Machine learning plays a crucial role enhancing maritime safety by leveraging its data analysis and predictive capabilities. However, there has been no review grounded bibliometric this field. To explore the research evolution knowledge frontier field of for autonomous shipping, was conducted using 719 publications from Web Science database, covering period 2000 up May 2024. This study utilized VOSviewer, alongside traditional literature methods, construct network map perform cluster analysis, thereby identifying hotspots, trends, emerging frontiers. The findings reveal robust cooperative among journals, researchers, institutions, countries or regions, underscoring interdisciplinary nature domain. Through review, we found that machine methods evolving toward systematic comprehensive direction, integration with AI human interaction may be next bellwether. Future will concentrate on three main areas: objectives towards proactive management coordination, developing advanced technologies, such as bio-inspired sensors, quantum learning, self-healing systems, decision-making algorithms generative adversarial networks (GANs), hierarchical reinforcement (HRL), federated learning. By visualizing collaborative networks, analyzing evolutionary lays groundwork pioneering advancements sets visionary angle future shipping. Moreover, it facilitates partnerships between industry academia, making concerted efforts domain USVs.
Language: Английский