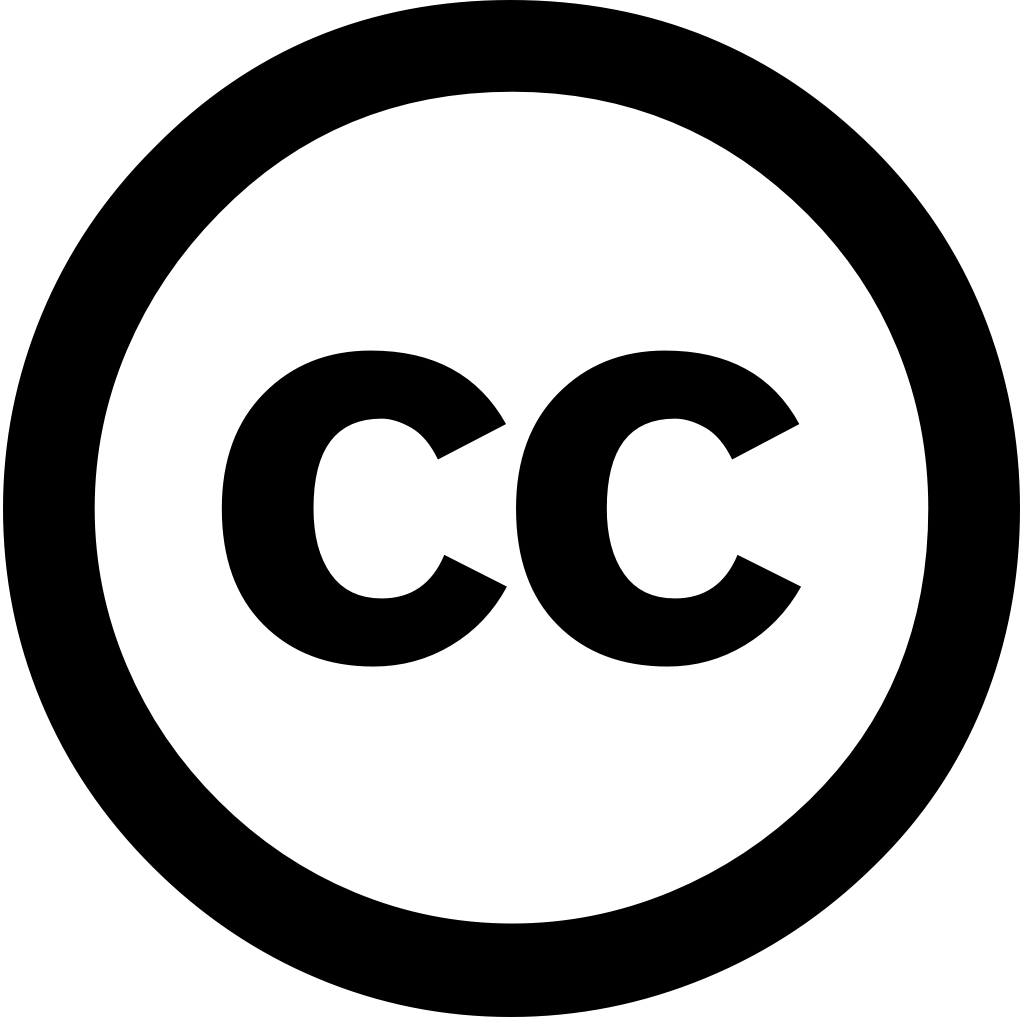
International Journal of Energy Research, Journal Year: 2025, Volume and Issue: 2025(1)
Published: Jan. 1, 2025
Specific capacitance plays a critical role when assessing the performance of supercapacitor. Hence, its prediction is crucial for evaluating electrochemical electric double‐layer capacitors (EDLCs). Machine learning (ML) offers prospect predicting with nominal investment in synthesis and testing electrode materials. Herein, six ML models: random forest (RF), artificial neural network (ANN), tree (RT), committee (RC), subspace (RS), support vector machine (SVM) regressor are used to analyze effect four hetero atom doping (nitrogen, boron, sulfur, phosphorous) on EDLCs. Amongst all, RF, ANN, RS showed highest correlation values 0.9996, 0.9993 0.9867, respectively, lowest root mean square 0.93, 1.19, 2.31, through selection 12 key input descriptors basis physical, structural, test, operational, parameters. Furthermore, attribute prioritization was introduced identify rank important features within dataset. It highlights that specific surface area, total pore volume, nitrogen most significant among selected features. With fewer iterations, developed models’ estimation accuracy surpassed other state‐of‐art models literature. In perspective, this study considers an extensive dataset extracted from more than 250 research articles heteroatom‐doped carbon electrodes. also provides insights into significance modeling technology.
Language: Английский