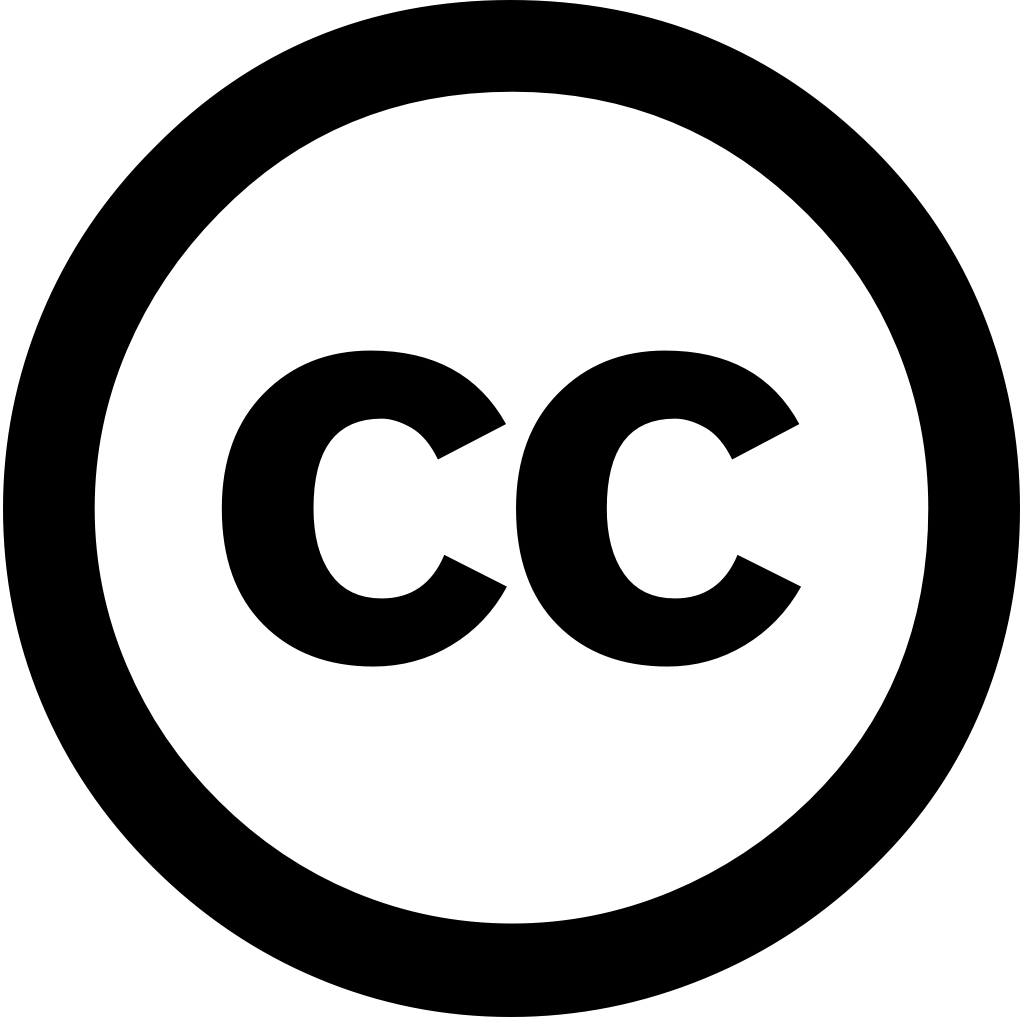
arXiv (Cornell University), Journal Year: 2023, Volume and Issue: unknown
Published: Jan. 1, 2023
Detecting travel modes from global navigation satellite system (GNSS) trajectories is essential for understanding individual behavior and a prerequisite achieving sustainable transport systems. While studies have acknowledged the benefits of incorporating geospatial context information into mode detection models, few summarized modeling approaches analyzed significance these features, hindering development an efficient model. Here, we identify representations related work propose analytical pipeline to assess contribution based on random forest model SHapley Additive exPlanation (SHAP) method. Through experiments large-scale GNSS tracking dataset, report that features describing relationships with infrastructure networks, such as distance railway or road network, significantly contribute model's prediction. Moreover, point entities help public travel, but most land-use land-cover barely task. We finally reveal contexts distinct contributions in identifying different modes, providing insights selecting appropriate approaches. The results this study enhance our relationship between movement guide implementation effective models.
Language: Английский