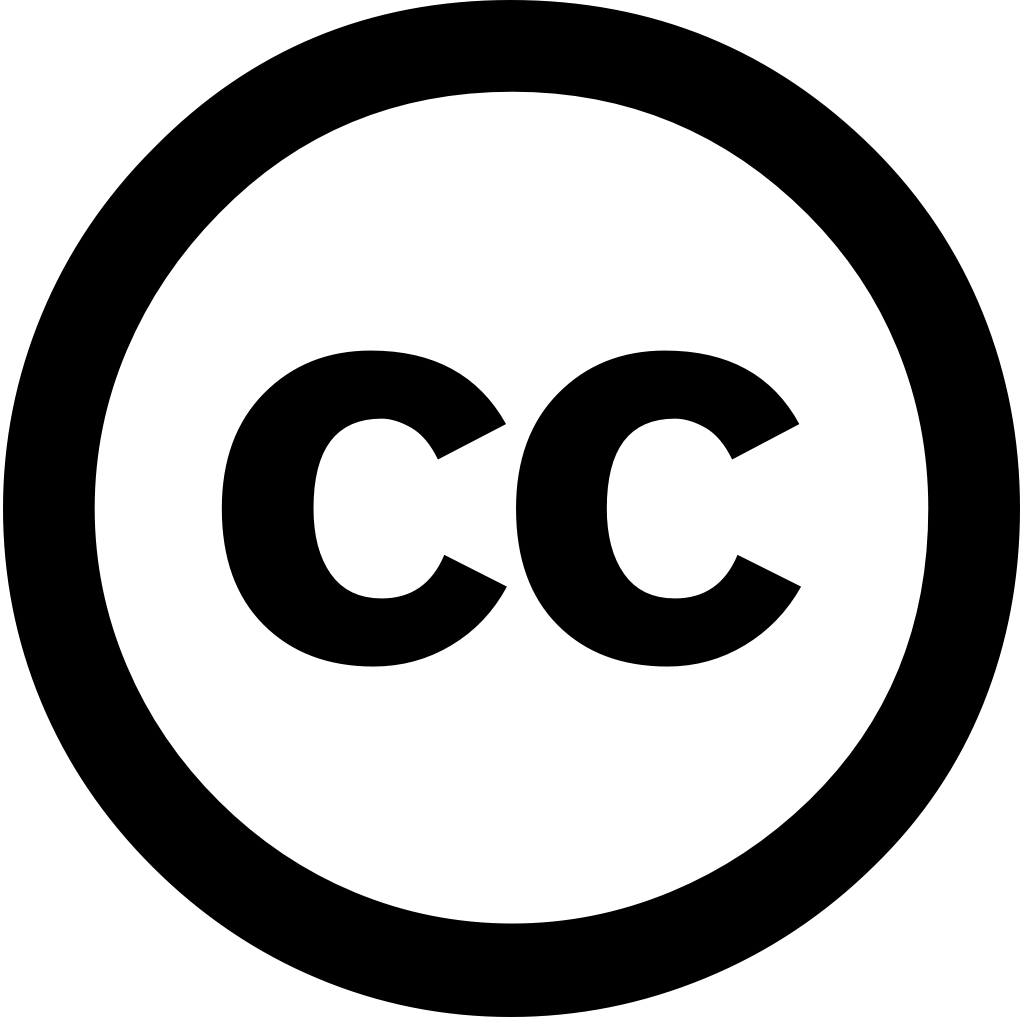
Journal of Energy Storage, Journal Year: 2024, Volume and Issue: 106, P. 114909 - 114909
Published: Dec. 3, 2024
Language: Английский
Journal of Energy Storage, Journal Year: 2024, Volume and Issue: 106, P. 114909 - 114909
Published: Dec. 3, 2024
Language: Английский
Transport Policy, Journal Year: 2025, Volume and Issue: unknown
Published: Jan. 1, 2025
Language: Английский
Citations
1Machines, Journal Year: 2025, Volume and Issue: 13(1), P. 54 - 54
Published: Jan. 13, 2025
In this article, the results of an experimental investigation and a 1D modeling activity on steady-state performance wastegated turbocharger turbine for spark ignition engines are presented. An campaign to analyze different waste-gate valve openings was conducted at test bench components propulsion systems University Genoa. Thanks activity, model is developed assess interaction between flow through impeller by-pass port. Advanced techniques crucial improving assessment turbines and, consequently, enhancing engine–turbocharger matching calculation. The initial tuning based characteristic maps obtained with port kept closed. study then highlights behavior considering its openings. It found that more refined necessary accurately define mass rate valve. After independently models valve, their analyzed in parallel-flow conditions. highlight significant interactions two must be taken into account reduce inaccuracies engine-turbocharger These lead reduced swallowing capacity impeller. This reduction has impact power delivered compressor, boost pressure, engine backpressure. suggest methods generally adopted consider as nozzles working parallel under same thermodynamic condition could insufficient behavior.
Language: Английский
Citations
0Transportation Research Part D Transport and Environment, Journal Year: 2025, Volume and Issue: 141, P. 104652 - 104652
Published: Feb. 19, 2025
Language: Английский
Citations
0Sustainable Cities and Society, Journal Year: 2025, Volume and Issue: unknown, P. 106289 - 106289
Published: March 1, 2025
Language: Английский
Citations
0Batteries, Journal Year: 2025, Volume and Issue: 11(3), P. 107 - 107
Published: March 13, 2025
Artificial Neural Networks (ANNs) improve battery management in electric vehicles (EVs) by enhancing the safety, durability, and reliability of electrochemical batteries, particularly through improvements State Charge (SOC) estimation. EV batteries operate under demanding conditions, which can affect performance and, extreme cases, lead to critical failures such as thermal runaway—an exothermic chain reaction that may result overheating, fires, even explosions. Addressing these risks requires advanced diagnostic strategies, machine learning presents a powerful solution due its ability adapt across multiple facets management. The versatility ML enables application material discovery, model development, quality control, real-time monitoring, charge optimization, fault detection, positioning it an essential technology for modern systems. Specifically, ANN models excel at detecting subtle, complex patterns reflect health performance, crucial accurate SOC effectiveness applications this domain, however, is highly dependent on selection datasets, relevant features, suitable algorithms. Advanced techniques active are being explored enhance improving models’ responsiveness diverse nuanced behavior. This compact survey consolidates recent advances estimation, analyzing current state field highlighting challenges opportunities remain. By structuring insights from extensive literature, paper aims establish ANNs foundational tool next-generation systems, ultimately supporting safer more efficient EVs robust safety protocols. Future research directions include refining dataset quality, optimizing algorithm selection, precision, thereby broadening ANNs’ role ensuring reliable vehicles.
Language: Английский
Citations
0Journal of Industrial Ecology, Journal Year: 2025, Volume and Issue: unknown
Published: April 9, 2025
Abstract Transitioning to electric vehicles has become a global carbon neutrality policy goal. However, vehicle electrification may have different outcomes depending on the energy mix scenario, average driving distance, lifetime, and manufacturing supply chain in country. Hence, this study, we applied life cycle optimization approach identify optimal car replacement periods, which would yield lowest cumulative CO 2 emissions for 2020–2050 six countries: China, Germany, Finland, India, Japan, United States. The results showed that discrepancy is evident between existing policies of To optimize emission reduction, these necessitate revision. Additionally, given profound impact region‐specific parameters, such as distance preferences, States should endeavor develop state‐specific predicated upon detailed state‐level data.
Language: Английский
Citations
0International Journal of Hydrogen Energy, Journal Year: 2025, Volume and Issue: 130, P. 605 - 614
Published: May 1, 2025
Language: Английский
Citations
0Energy, Journal Year: 2025, Volume and Issue: unknown, P. 136770 - 136770
Published: May 1, 2025
Language: Английский
Citations
0Energy Reports, Journal Year: 2024, Volume and Issue: 12, P. 5834 - 5845
Published: Nov. 27, 2024
Language: Английский
Citations
0Journal of Energy Storage, Journal Year: 2024, Volume and Issue: 106, P. 114909 - 114909
Published: Dec. 3, 2024
Language: Английский
Citations
0