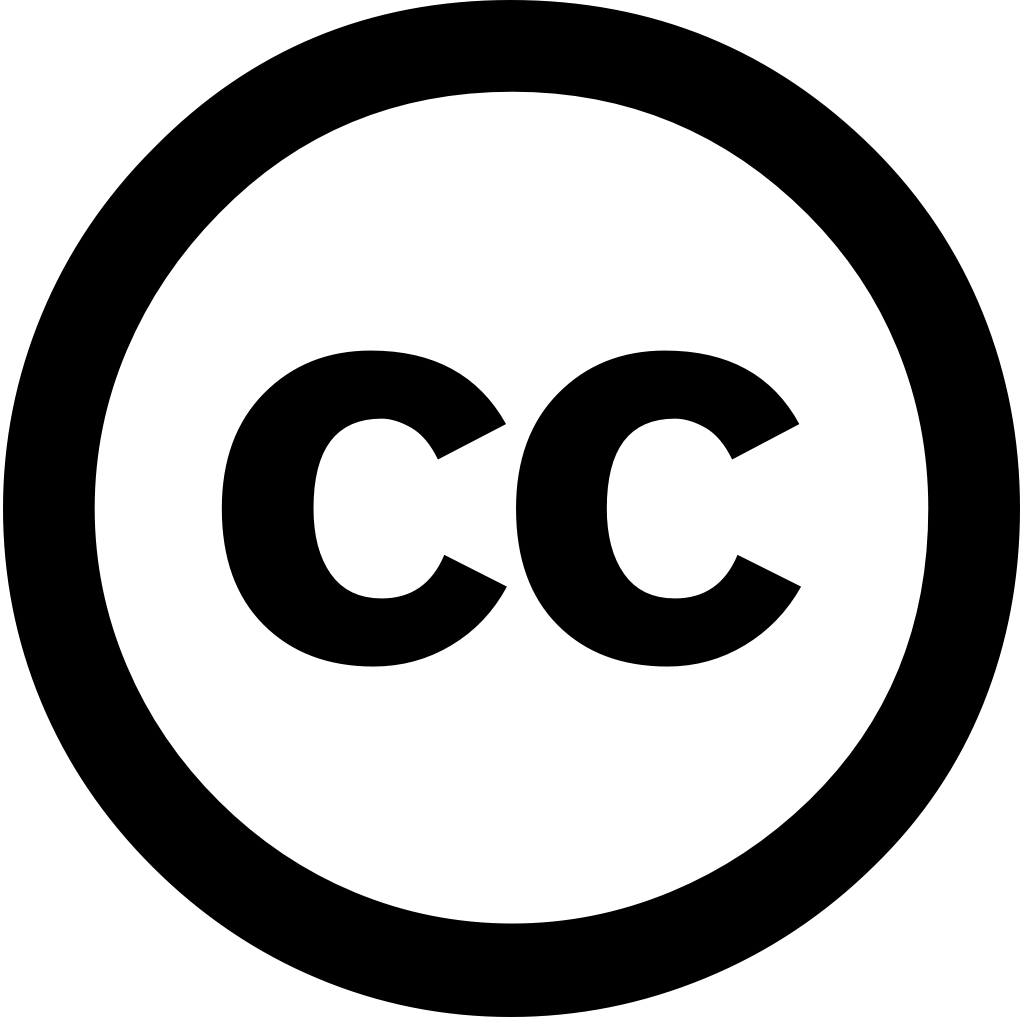
Scientific Reports, Journal Year: 2024, Volume and Issue: 14(1)
Published: Dec. 30, 2024
Accurate estimation of the soil resilient modulus (M
Language: Английский
Scientific Reports, Journal Year: 2024, Volume and Issue: 14(1)
Published: Dec. 30, 2024
Accurate estimation of the soil resilient modulus (M
Language: Английский
Structures, Journal Year: 2024, Volume and Issue: 68, P. 107159 - 107159
Published: Sept. 1, 2024
Language: Английский
Citations
14Modeling Earth Systems and Environment, Journal Year: 2024, Volume and Issue: 10(4), P. 5079 - 5102
Published: May 31, 2024
Language: Английский
Citations
10Scientific Reports, Journal Year: 2024, Volume and Issue: 14(1)
Published: Aug. 6, 2024
Accurately predicting the Modulus of Resilience (MR) subgrade soils, which exhibit non-linear stress–strain behaviors, is crucial for effective soil assessment. Traditional laboratory techniques determining MR are often costly and time-consuming. This study explores efficacy Genetic Programming (GEP), Multi-Expression (MEP), Artificial Neural Networks (ANN) in forecasting using 2813 data records while considering six key parameters. Several Statistical assessments were utilized to evaluate model accuracy. The results indicate that GEP consistently outperforms MEP ANN models, demonstrating lowest error metrics highest correlation indices (R2). During training, achieved an R2 value 0.996, surpassing (R2 = 0.97) 0.95) models. Sensitivity SHAP (SHapley Additive exPlanations) analysis also performed gain insights into input parameter significance. revealed confining stress (21.6%) dry density (26.89%) most influential parameters MR. corroborated these findings, highlighting critical impact on predictions. underscores reliability as a robust tool precise prediction applications, providing valuable performance significance across various machine-learning (ML) approaches.
Language: Английский
Citations
9Soil Dynamics and Earthquake Engineering, Journal Year: 2024, Volume and Issue: 186, P. 108943 - 108943
Published: Sept. 5, 2024
Language: Английский
Citations
9Rock Mechanics and Rock Engineering, Journal Year: 2025, Volume and Issue: unknown
Published: Feb. 5, 2025
Language: Английский
Citations
1Expert Systems with Applications, Journal Year: 2024, Volume and Issue: 256, P. 124900 - 124900
Published: July 30, 2024
Language: Английский
Citations
4Journal of environmental chemical engineering, Journal Year: 2024, Volume and Issue: 12(6), P. 114330 - 114330
Published: Oct. 6, 2024
Language: Английский
Citations
4Transportation Geotechnics, Journal Year: 2025, Volume and Issue: unknown, P. 101516 - 101516
Published: Feb. 1, 2025
Language: Английский
Citations
0Bulletin of Engineering Geology and the Environment, Journal Year: 2025, Volume and Issue: 84(4)
Published: March 19, 2025
Language: Английский
Citations
0Materials Today Communications, Journal Year: 2025, Volume and Issue: unknown, P. 112379 - 112379
Published: March 1, 2025
Language: Английский
Citations
0