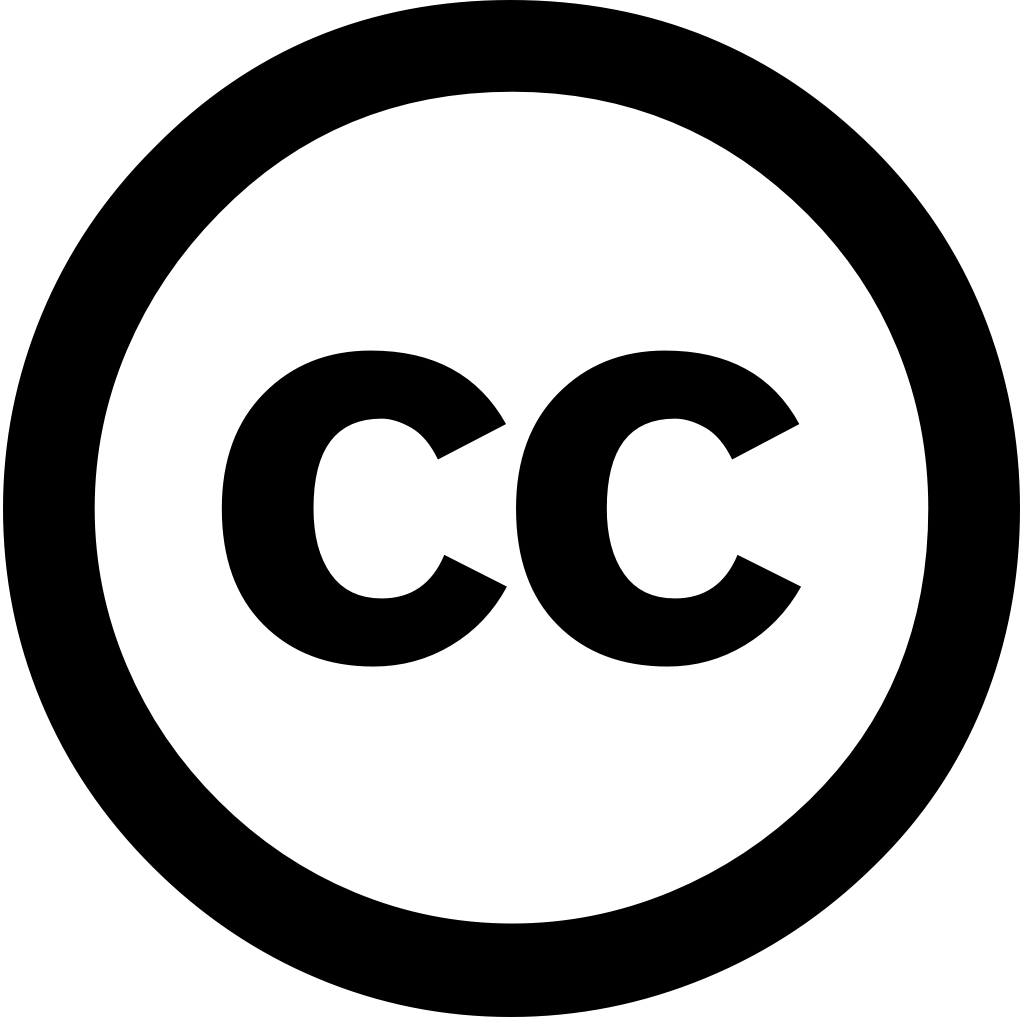
Journal of Environmental Management, Journal Year: 2024, Volume and Issue: 373, P. 123570 - 123570
Published: Dec. 6, 2024
Language: Английский
Journal of Environmental Management, Journal Year: 2024, Volume and Issue: 373, P. 123570 - 123570
Published: Dec. 6, 2024
Language: Английский
Sustainability, Journal Year: 2024, Volume and Issue: 16(17), P. 7489 - 7489
Published: Aug. 29, 2024
Floods, caused by intense rainfall or typhoons, overwhelming urban drainage systems, pose significant threats to areas, leading substantial economic losses and endangering human lives. This study proposes a methodology for flood assessment in areas using multiclass classification approach with Deep Neural Network (DNN) optimized through hyperparameter tuning genetic algorithms (GAs) leveraging remote sensing data of dataset the Ibadan metropolis, Nigeria Metro Manila, Philippines. The results show that DNN model significantly improves risk accuracy (Ibadan-0.98) compared datasets containing only location precipitation (Manila-0.38). By incorporating soil into model, as well reducing number classes, it is able predict risks more accurately, providing insights proactive mitigation strategies planning.
Language: Английский
Citations
12Journal of Hydrology, Journal Year: 2025, Volume and Issue: unknown, P. 132852 - 132852
Published: Feb. 1, 2025
Language: Английский
Citations
1Sustainable Cities and Society, Journal Year: 2025, Volume and Issue: 123, P. 106277 - 106277
Published: March 11, 2025
Language: Английский
Citations
1Journal of Hydrology, Journal Year: 2025, Volume and Issue: unknown, P. 133117 - 133117
Published: April 1, 2025
Language: Английский
Citations
1Water, Journal Year: 2025, Volume and Issue: 17(9), P. 1276 - 1276
Published: April 25, 2025
Flash floods rank among the most devastating natural hazards, causing widespread socio-economic, environmental, and infrastructural damage globally. Hence, innovative management approaches are required to mitigate their increasing frequency intensity, driven by factors such as climate change urbanization. Accordingly, this study introduced an integrated flood assessment approach (IFAA) for sustainable of risks integrating analytical hierarchy process-weighted linear combination (AHP-WLC) fuzzy-ordered weighted averaging (FOWA) methods. The IFAA was applied in South Khorasan Province, Iran, arid flood-prone region. Fifteen controlling factors, including rainfall (RF), slope (SL), land use/land cover (LU/LC), distance rivers (DTR), were processed using collected data. AHP-WLC method classified region into susceptibility zones: very low (10.23%), (23.14%), moderate (29.61%), high (17.54%), (19.48%). FOWA technique ensured these findings introducing optimistic pessimistic fuzzy scenarios risk. extreme scenario indicated that 98.79% area highly sensitive flooding, while less than 5% deemed low-risk under conservative scenarios. Validation demonstrated its reliability, with achieving curve (AUC) 0.83 average accuracy ~75% across all Findings revealed elevated dangers densely populated industrialized areas, particularly northern southern regions, which influenced proximity rivers. Therefore, also addressed challenges linked development goals (SDGs), SDG 13 (climate action), proposing adaptive strategies meet 60% targets. This research can offer a scalable framework risk management, providing actionable insights hydrologically vulnerable regions worldwide.
Language: Английский
Citations
1Journal of Hydrology, Journal Year: 2024, Volume and Issue: 635, P. 131169 - 131169
Published: April 5, 2024
This paper presents a purely data-driven deep-learning approach for flood maps forecasting. For the first time in this context Transformer-based algorithm is employed to address one of main issues early-warning systems propagation, i.e., long computational times required forecast inundation evolution real time. The proposed model, named "FloodSformer", trained extract spatiotemporal information from short sequence water depth and predict map at subsequent instant. Then, future maps, we employ an autoregressive procedure based on surrogate model. method was applied both synthetic dam-break scenarios case study, specifically ideal failure Parma River dam (Italy). training testing datasets were generated numerically two-dimensional hydraulic simulations. In test case, average Root Mean Square Error found be equal 10.4 cm. (e.g., 90 representing lead 3 h, takes less than 1 min) makes FloodSformer model suitable tool real-time emergency applications.
Language: Английский
Citations
7Journal of Hydrology, Journal Year: 2024, Volume and Issue: 636, P. 131263 - 131263
Published: May 3, 2024
This paper investigates the application of physics-informed neural networks (PINNs) to solve free-surface flow problems governed by 2D shallow water equations (SWEs). Two types PINNs are developed and analyzed: a fully connected network (PIFCN) convolutional (PICN). The eliminate need for labeled data training employing SWEs, initial boundary conditions as components loss function be minimized. Results from set idealized real-world tests showed that prediction accuracy computation time (i.e., time) both may less affected resolution domain discretization when compared against solutions Finite Volume (FV) model. Overall, PICN shows better trade-off between computational speed than PIFCN. Also, our results indicated can provide more 5 times higher FV model, while simulation with coarse (e.g., 10 m) sub-centimeter accurate (RMSE) at least one order magnitude faster PINNs.Results river flood delivered speed-accuracy model in terms predicting depth, models outperformed predictions total discharge.
Language: Английский
Citations
7Water Research, Journal Year: 2024, Volume and Issue: 270, P. 122816 - 122816
Published: Nov. 19, 2024
Language: Английский
Citations
5Journal of Hydrology, Journal Year: 2025, Volume and Issue: unknown, P. 132785 - 132785
Published: Feb. 1, 2025
Language: Английский
Citations
0Journal of Water and Climate Change, Journal Year: 2025, Volume and Issue: unknown
Published: Jan. 18, 2025
ABSTRACT In this study, a physics-informed machine learning-based surrogate model (SM) for the variable infiltration capacity (VIC) was developed to improve simulation efficiency in Yarlung Tsangpo River basin. The approach combines empirical orthogonal function decomposition of low-fidelity VIC models extract spatial and temporal features, with learning techniques applied refine feature series. This allows accurate reconstruction high-fidelity simulations from results model. Using SM built 1.0°-resolution as an example, study highlights challenges solutions associated simulations. significantly improves accuracy, achieving Kling–Gupta 0.88, Nash–Sutcliffe 0.97, PBIAS value −6.21% reduced computational demands. Additionally, different methods impact performance SM, support vector regression performing best these methods. SMs varying resolutions maintain similar but higher notably enhance efficiency, reducing time by 86.31% when compared These findings demonstrate potential while requirements.
Language: Английский
Citations
0