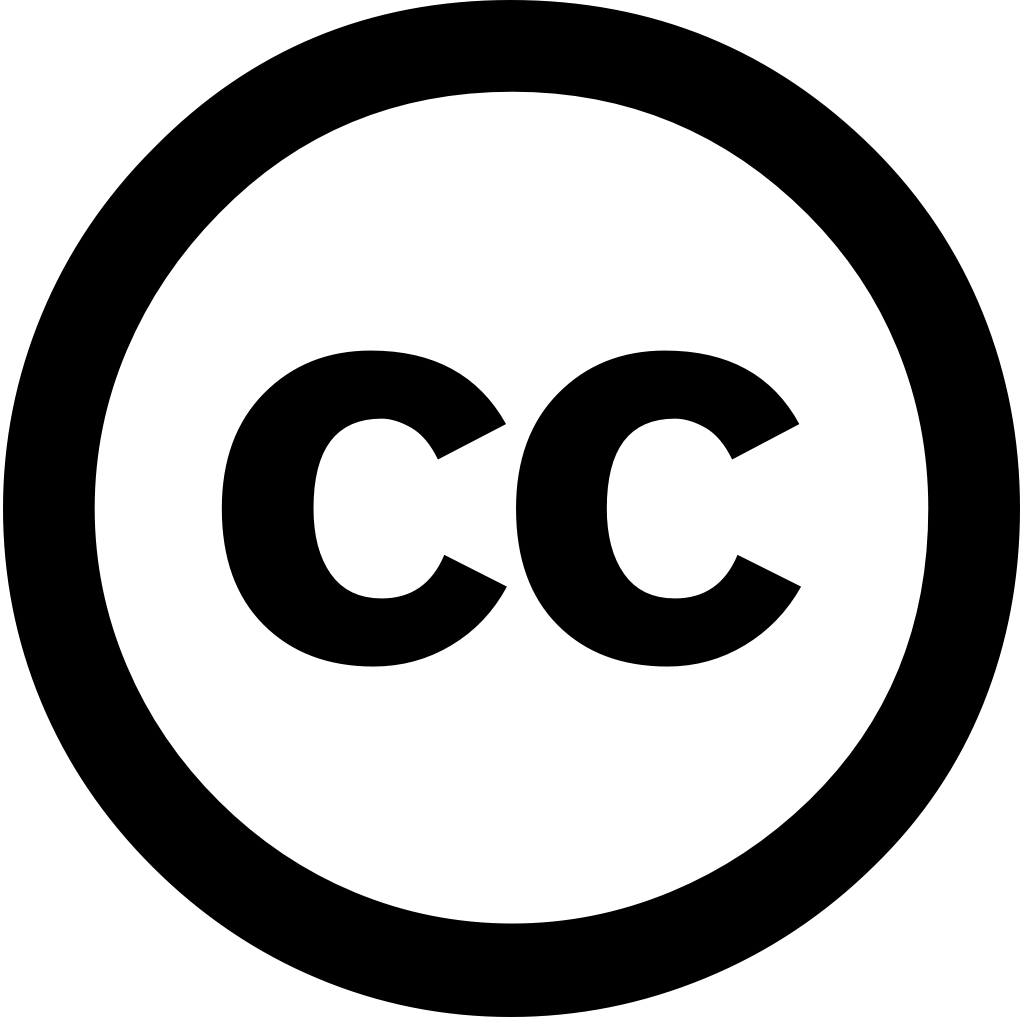
American Journal of Preventive Medicine, Journal Year: 2023, Volume and Issue: 66(3), P. 568 - 572
Published: Dec. 4, 2023
Language: Английский
American Journal of Preventive Medicine, Journal Year: 2023, Volume and Issue: 66(3), P. 568 - 572
Published: Dec. 4, 2023
Language: Английский
Scientific Reports, Journal Year: 2023, Volume and Issue: 13(1)
Published: Aug. 21, 2023
The COVID-19 virus caused a global pandemic leading to swift policy response. While this response was designed prevent the spread of and support those with COVID-19, there is growing evidence regarding measurable impacts on non-COVID-19 patients. paper uses large dataset from administrative records Brazilian public health system (SUS) estimate spillover effects in critically ill care delivery, i.e. additional mortality risk that ICU hospitalizations generate patients receiving intensive care. data contain universe SUS February 26, 2020 December 31, 2021. Spillover estimates are obtained high-dimensional fixed regression models control for number unobservable confounders. Our findings indicate that, average, increased by 1.296 percentage points, 95% CI 1.145-1.448. larger non-COVID due diseases respiratory system, skin subcutaneous tissue, infectious parasitic diseases. As July 2023, WHO reports more than 6.9 million deaths infection. However, our suggest pandemic's total death toll much higher.
Language: Английский
Citations
10Healthcare, Journal Year: 2022, Volume and Issue: 10(2), P. 223 - 223
Published: Jan. 25, 2022
An increase in opioid use has led to an crisis during the last decade, leading declarations of a public health emergency. In response this call, Houston Emergency Opioid Engagement System (HEROES) was established and created emergency access pathway into long-term recovery for individuals with disorder. A major contributor success program is retention enrolled program.We have identified dropout from after 90 120 days. Based on more than 700 participants, we developed machine learning approach predict individualized risk dropping out program.Our model achieved sensitivity 0.81 specificity 0.65 at days improved performance 0.86 0.66 Additionally, individual factors dropout, including previous overdose relapse improvement reported quality life.Our informatics provides insight area where programs may allocate additional resources order retain high-risk chances recovery.
Language: Английский
Citations
14Current Opinion in Psychiatry, Journal Year: 2023, Volume and Issue: 36(4), P. 308 - 315
Published: April 25, 2023
Opioid overdose events are a time sensitive medical emergency, which is often reversible with naloxone administration if detected in time. Many countries facing rising opioid deaths and have been implementing rapid response Systems (ROORS). We describe how technology increasingly being used ROORS design, implementation delivery.Technology can contribute significant ways to implementation, delivery. Artificial intelligence-based modelling simulations alongside wastewater-based epidemiology be inform policy decisions around access laws effective distribution strategies. Data linkage machine learning projects support service delivery organizations mobilize distribute community resources of ROORS. Digital phenotyping an advancement data projects, potentially leading precision responses. At the coalface, detection devices through fixed location or wearable sensors, improved connectivity, smartphone applications drone-based emergency all role improving outcomes from overdose. driven technologies also important empowering responses overdose.This review highlights importance applied every aspect Key areas development include need protect marginalized groups algorithmic bias, better understanding individual trajectories new reversal agents drug methods.
Language: Английский
Citations
8International Journal of Drug Policy, Journal Year: 2021, Volume and Issue: 94, P. 103332 - 103332
Published: June 18, 2021
Language: Английский
Citations
17Journal of Addiction Medicine, Journal Year: 2022, Volume and Issue: unknown
Published: Aug. 1, 2022
Objective Patients receiving medication for opioid use disorder (MOUD) may continue using nonprescribed drugs or have trouble with adherence, and it is difficult to predict which patients will do so. In this study, we develop validate an automated risk-modeling framework abstinence adherence at a patient's next attended appointment evaluate the predictive performance of machine-learning algorithms versus logistic regression. Methods Urine drug screen attendance records from 40,005 appointments drawn 2742 multilocation office-based MOUD program were used train regression, ridge XGBoost models composite indicator treatment (opioid-negative norbuprenorphine-positive urine, no evidence urine adulteration) appointment. Results The model had similar accuracy discriminative ability (accuracy, 88%; area under receiver operating curve, 0.87) two regression 0.87). nearly perfect calibration in independent validation data; slightly overestimated likelihood. Historical rate, fentanyl-positive current strongest contributors Discussion There need risk prediction tools improve delivery MOUD. This study presents portable each algorithm appears provide classification models; however, offer improved estimates compared
Language: Английский
Citations
11INQUIRY The Journal of Health Care Organization Provision and Financing, Journal Year: 2024, Volume and Issue: 61
Published: Jan. 1, 2024
The substance use disorder epidemic has emerged as a serious public health crisis, presenting complex challenges. Visual analytics offers unique approach to address this complexity and facilitate effective interventions. This paper details the development of an innovative visual dashboard, aimed at enhancing our understanding epidemic. By employing record linkage techniques, we integrate diverse data sources provide comprehensive view Adherence responsive, open, user-centered design principles ensures dashboard’s usefulness usability. Our encourages collaboration among various stakeholders, including researchers, politicians, healthcare practitioners. Through illustrative outputs, demonstrate how dashboard can deepen epidemic, support intervention strategies, evaluate effectiveness implemented measures. concludes with discussion cases limitations.
Language: Английский
Citations
2Journal of Epidemiology & Community Health, Journal Year: 2024, Volume and Issue: unknown, P. jech - 222263
Published: Oct. 10, 2024
The opioid overdose mortality crisis in the USA is an ongoing public health epidemic. Ongoing law enforcement strategies to disrupt local unregulated drug markets can have iatrogenic effect of increasing by driving consumers towards new suppliers with unpredictable products unknown potency.
Language: Английский
Citations
2International Journal of Drug Policy, Journal Year: 2023, Volume and Issue: 117, P. 104065 - 104065
Published: May 23, 2023
In this paper, we offer a sociological analysis of early warning and outbreak in the field drug policy, focusing on opioid overdose. We trace how 'outbreak' is enacted as rupturing event which enables rapid reflex responses precautionary control, based largely short-term proximal indicators. make case for an alternative view outbreak. argue that practices detection projection help to materialise drug-related outbreaks are too focused short-term. Engaging with epidemiological work investigating epidemics overdose, show short-termism response fails appreciate slow violent pasts indicative ongoing need care structural societal change. Accordingly, gather together ideas 'slow emergency' (Ben Anderson), death' (Lauren Berlant) violence' (Rob Nixon), re-assemble 'long view'. This locates overdose long-term attritional processes deindustrialisation, pharmaceuticalisation, other forms violence, including criminalisation problematisation people who use drugs. Outbreaks evolve relation their pasts. To ignore can perpetuate harm. Attending social conditions create possibilities invites goes 'beyond outbreak' epidemic' generally configured.
Language: Английский
Citations
6Addiction, Journal Year: 2023, Volume and Issue: 118(5), P. 954 - 966
Published: Jan. 7, 2023
Likelihood of alcohol dependence (AD) is increased among people who transition to greater levels involvement at a younger age. Indicated interventions delivered early may be effective in reducing risk, but could costly. One way increase cost-effectiveness would develop prediction model that targeted the subset youth with use are highest risk subsequent AD.
Language: Английский
Citations
4Addiction, Journal Year: 2024, Volume and Issue: 119(10), P. 1792 - 1802
Published: June 24, 2024
Abstract Background and aims Opioid use disorder (OUD) opioid dependence lead to significant morbidity mortality, yet treatment retention, crucial for the effectiveness of medications like buprenorphine‐naloxone, remains unpredictable. Our objective was determine predictability 6‐month retention in buprenorphine‐naloxone using electronic health record (EHR) data from diverse clinical settings identify key predictors. Design This retrospective observational study developed validated machine learning‐based risk prediction models EHR data. Setting cases Data were sourced Stanford University's healthcare system Holmusk's NeuroBlu database, reflecting a wide range settings. The analyzed 1800 7957 encounters 2008 2023 2003 2023, respectively. Measurements Predict continuous prescription at least 6 months, without gap more than 30 days. performance learning assessed by area under receiver operating characteristic (ROC‐AUC) analysis as well precision, recall calibration. To further validate our approach's applicability, we conducted two secondary analyses: time‐to‐event on single site estimate duration continuity evaluated C‐index comparative evaluation against predictions made three human experts. Findings Attrition rates months 58% (NeuroBlu) 61% (Stanford). Prediction trained internally achieved ROC‐AUCs up 75.8 (95% confidence interval [CI] = 73.6–78.0). Addiction medicine specialists' show ROC‐AUC 67.8 CI 50.4–85.2). Time‐to‐event indicated median time 65 days, with random survival forest model achieving an average 65.9. top predictor identified included diagnosis dependence. Conclusions US patients or treated prescriptions appear have high (∼60%) attrition months. Machine datasets be able predict accuracy comparable that
Language: Английский
Citations
1