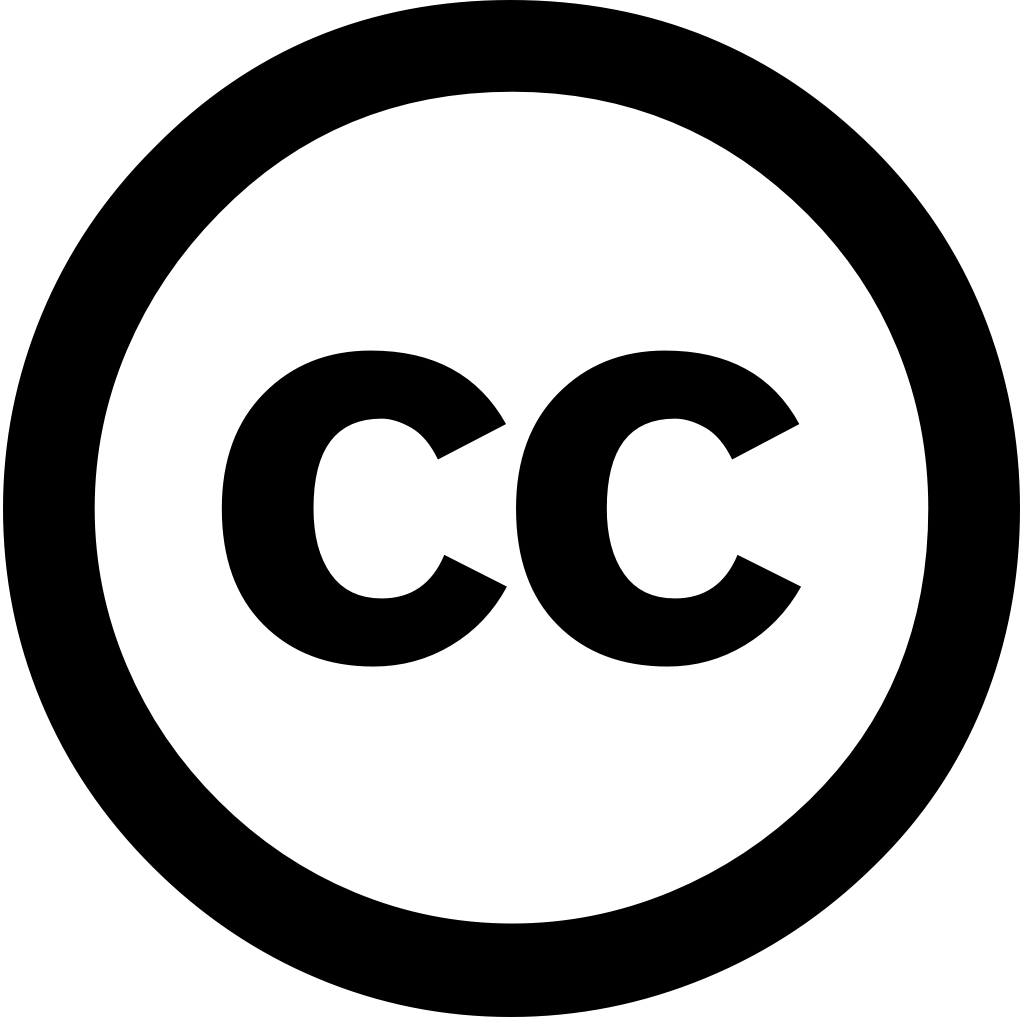
Environment International, Journal Year: 2025, Volume and Issue: 197, P. 109341 - 109341
Published: Feb. 18, 2025
Language: Английский
Environment International, Journal Year: 2025, Volume and Issue: 197, P. 109341 - 109341
Published: Feb. 18, 2025
Language: Английский
Electronics, Journal Year: 2025, Volume and Issue: 14(2), P. 386 - 386
Published: Jan. 20, 2025
While large language models (LLMs) are vital for retrieving relevant information from extensive knowledge bases, they always face challenges, including high costs and issues of credibility. Here, we developed a question answering system focused on human health risk using Retrieval-Augmented Generation (RAG). We first proposed framework to generate question–answer pairs, resulting in 300 high-quality pairs across six subfields. Subsequently, created both Naive RAG an Advanced RAG-based Question-Answering (Q&A) system. Performance evaluation the individual research subfields demonstrated that outperformed traditional LLMs (including ChatGPT ChatGLM) RAG. Finally, integrated module single subfield launch multi-knowledge base Our study represents novel application technology optimize retrieval methods assessment.
Language: Английский
Citations
0ACS ES&T Engineering, Journal Year: 2025, Volume and Issue: unknown
Published: Jan. 30, 2025
Electrochemical enhancing anaerobic cofermentation of waste activated sludge and food to produce volatile fatty acids (VFAs) represents an innovative promising approach. Despite its potential, optimizing system performance, providing early warnings, identifying biomarkers remain challenging tasks due the intricate interplay numerous environmental variables unclear dynamics microbial interactions. This study first employed machine learning (ML) models including XGBoost, random forest (RF), support vector regression (SVR), CatBoost forecast VFA production by integrating initial feedstock properties, electrochemical pretreatment conditions, fermentation parameters. demonstrated highest R2 0.977 lowest root-mean-square error (RMSE) at 95.69 mg COD/L. Key factors, days (VFA reaching 90% day 5), salinity (0.5–1.0 g/L), carbon-to-nitrogen (C/N) ratio (16.53–22), were identified as optimal for production. To enhance long-term monitoring facilitate warning systems, process indicators (pH, ORP, PNs, SCOD, PSs) from last used predict on following fine-tuning generative pretrain transformer (GPT), with gpt-3.5-turbo-0125 model exhibiting 0.837 ± 0.004 RMSE 296.98 3.65 Local sensitivity analysis revealed that SCOD was most important factor affecting Moreover, this ML uncover genus levels, Prevotella_7, Veillonella, Megasphaera, Lactobacillus, thereby elucidating nexus among communities, offered a novel modeling workflow cofermentation, enabling optimization mechanism exploration assistance large language models.
Language: Английский
Citations
0Environment International, Journal Year: 2025, Volume and Issue: 197, P. 109341 - 109341
Published: Feb. 18, 2025
Language: Английский
Citations
0