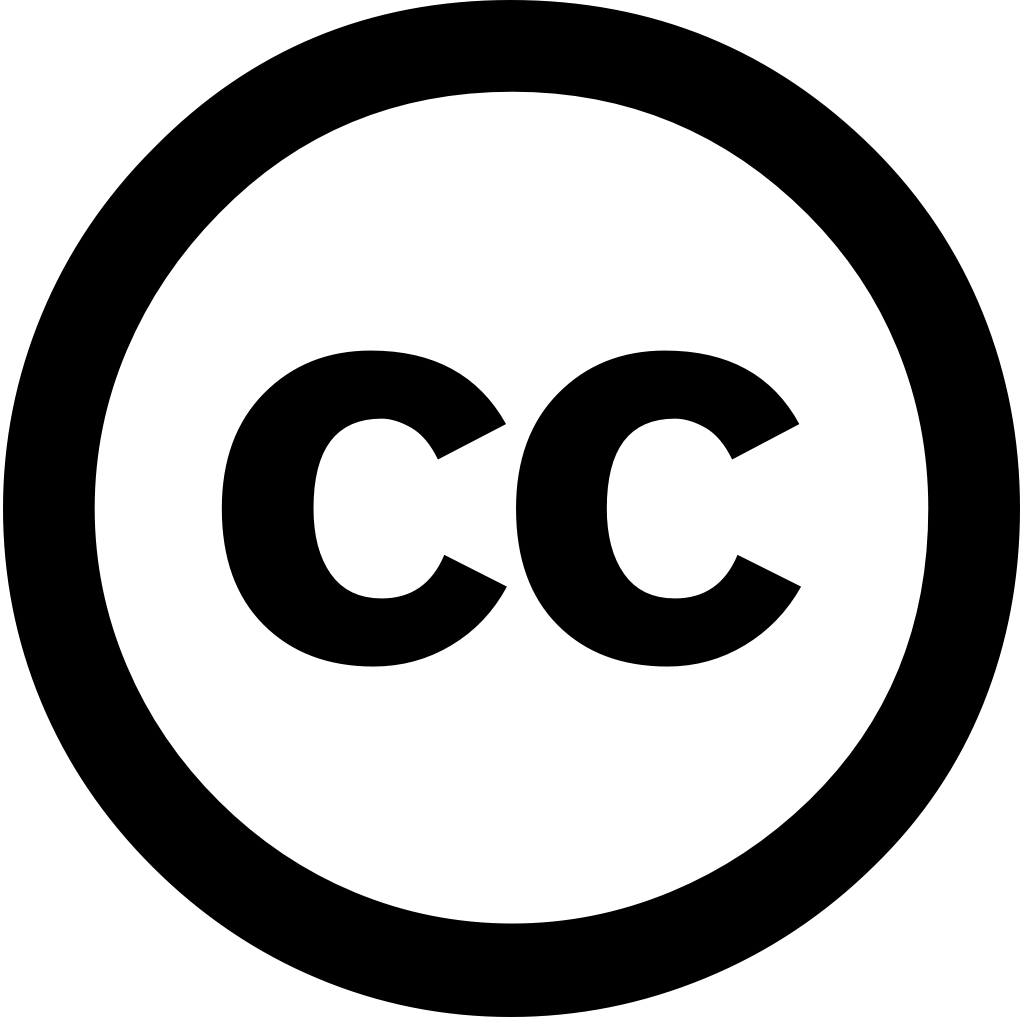
Research Square (Research Square), Journal Year: 2024, Volume and Issue: unknown
Published: Nov. 27, 2024
Language: Английский
Research Square (Research Square), Journal Year: 2024, Volume and Issue: unknown
Published: Nov. 27, 2024
Language: Английский
Frontiers in Pharmacology, Journal Year: 2025, Volume and Issue: 16
Published: April 8, 2025
Background: Parkinson's disease (PD), a prevalent and progressive neurodegenerative disorder, currently lacks effective satisfactory pharmacological treatments. Computational drug repurposing represents promising efficient strategy for discovery, aiming to identify new therapeutic indications existing pharmaceuticals. Methods: We employed drug-target network approach computationally repurpose FDA-approved drugs from databases such as DrugBank. A literature review was conducted select candidates not previously reported pharmacoprotective against PD. Subsequent in vitro evaluation utilized Cell Counting Kit-8 (CCK8) assays assess the neuroprotective effects of selected compounds SH-SY5Y cell model induced by 1-methyl-4-phenylpyridinium (MPP+). Furthermore, an vivo mouse 1-methyl-4-phenyl-1,2,3,6-tetrahydropyridine (MPTP) developed investigate mechanisms action potential identified candidates. Results: Our 176 candidates, with 28 their anti-Parkinsonian lack prior PD-related reporting. CCK8 showed significant neuroprotection cells Omaveloxolone Cyproheptadine. In MPTP-induced model, Cyproheptadine inhibited interleukin-6 (IL-6) expression prevented Tyrosine Hydroxylase (TH) downregulation via MAPK/NFκB pathway, while alleviated TH downregulation, potentially through Kelch-like ECH-associated protein 1 (KEAP1)-NF-E2-related factor 2 (Nrf2)/antioxidant response element (ARE) pathway. Both preserved dopaminergic neurons improved neurological deficits PD model. Conclusion: This study elucidates treatment application computational repurposing, thereby underscoring its efficacy discovery strategy.
Language: Английский
Citations
0Journal of Parkinson s Disease, Journal Year: 2025, Volume and Issue: unknown
Published: April 27, 2025
Recent years have seen successes in symptomatic drugs for Parkinson's disease, but the development of treatments stopping disease progression continues to fail clinical drug trials, largely due lack efficacy drugs. This may be related limited understanding mechanisms, data heterogeneity, poor target screening and candidate selection, challenges determining optimal dosage levels, reliance on animal models, insufficient patient participation, adherence trials. Most recent applications digital health technologies artificial intelligence (AI)-based tools focused mainly stages before used AI-based algorithms or models discover novel targets, inhibitors indications, recommend candidates dosage, promote remote collection. paper reviews state literature highlights strengths limitations approaches discovery from 2021 2024, offers recommendations future research practice success
Language: Английский
Citations
0Briefings in Bioinformatics, Journal Year: 2024, Volume and Issue: 25(6)
Published: Sept. 12, 2024
Drug repurposing has emerged as a effective and efficient strategy to identify new treatments for variety of diseases. One the most approaches discovering potential drug candidates involves utilization Knowledge Graphs (KGs). This review comprehensively explores some prominent KGs, detailing their structure, data sources, how they facilitate drugs. In addition this paper delves into various artificial intelligence techniques that enhance process repurposing. These methods not only accelerate identification viable but also improve precision predictions by leveraging complex datasets advanced algorithms. Furthermore, importance explainability in is emphasized. Explainability are crucial provide insights reasoning behind AI-generated predictions, thereby increasing trustworthiness transparency process. We will discuss several can be employed validate these ensuring both reliable understandable.
Language: Английский
Citations
3Journal of Chemical Information and Modeling, Journal Year: 2024, Volume and Issue: unknown
Published: Sept. 20, 2024
A knowledge graph (KG) is a technique for modeling entities and their interrelations. Knowledge embedding (KGE) translates these relationships into continuous vector space to facilitate dense efficient representations. In the domain of chemistry, applying KG KGE techniques integrates heterogeneous chemical information coherent user-friendly framework, enhances representation data features, beneficial downstream tasks, such as property prediction. This paper begins with comprehensive review classical contemporary methodologies, including distance-based models, semantic matching neural network-based approaches. We then catalogue primary databases employed in chemistry biochemistry that furnish KGs essential data. Subsequently, we explore latest applications focusing on risk assessment, prediction, drug discovery. Finally, discuss current challenges provide perspective potential future developments.
Language: Английский
Citations
2Methods, Journal Year: 2024, Volume and Issue: 227, P. 78 - 85
Published: May 14, 2024
Language: Английский
Citations
1Chinese Medicine, Journal Year: 2024, Volume and Issue: 19(1)
Published: Sept. 11, 2024
Language: Английский
Citations
1Research Square (Research Square), Journal Year: 2024, Volume and Issue: unknown
Published: Nov. 27, 2024
Language: Английский
Citations
0