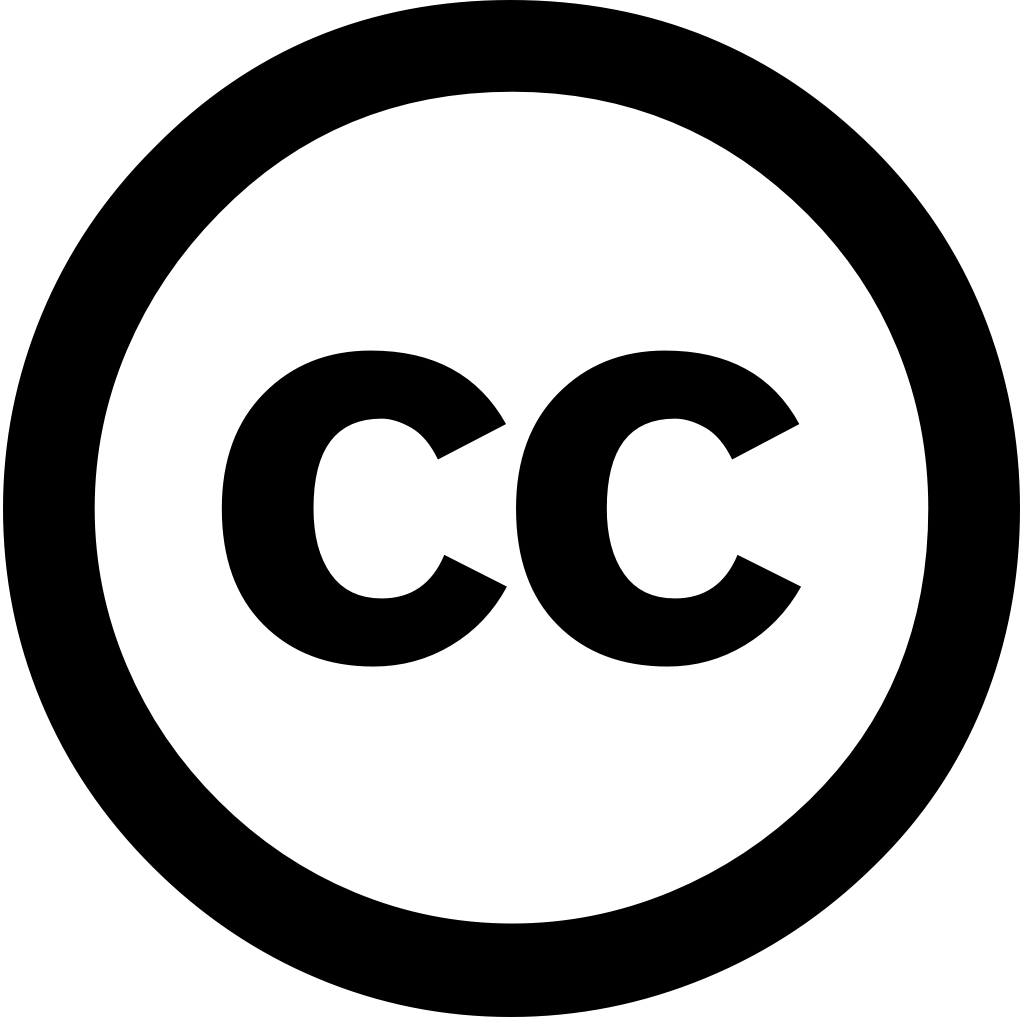
Machine Learning Science and Technology, Journal Year: 2024, Volume and Issue: 5(4), P. 041001 - 041001
Published: Nov. 5, 2024
The field of computational chemistry is increasingly leveraging machine learning (ML) potentials to predict molecular properties with high accuracy and efficiency, providing a viable alternative traditional quantum mechanical (QM) methods, which are often computationally intensive. Central the success ML models quality comprehensiveness data sets on they trained. Quantum databases, comprising extensive information structures, energies, forces, other derived from QM calculations, crucial for developing robust generalizable potentials. In this review, we provide an overview current landscape chemical databases. We examine key characteristics functionalities prominent resources, including types store, level electronic structure theory employed, diversity space covered, methodologies used creation. Additionally, updatable resource provided track new databases at https://github.com/Arif-PhyChem/datasets_and_databases_4_MLPs. Looking forward, discuss challenges associated rapid growth emphasizing need accessible resources ensure long-term utility them. also address importance format standardization ongoing efforts align FAIR principles enhance interoperability reusability. Drawing inspiration established materials advocate development user-friendly sustainable platforms these
Language: Английский