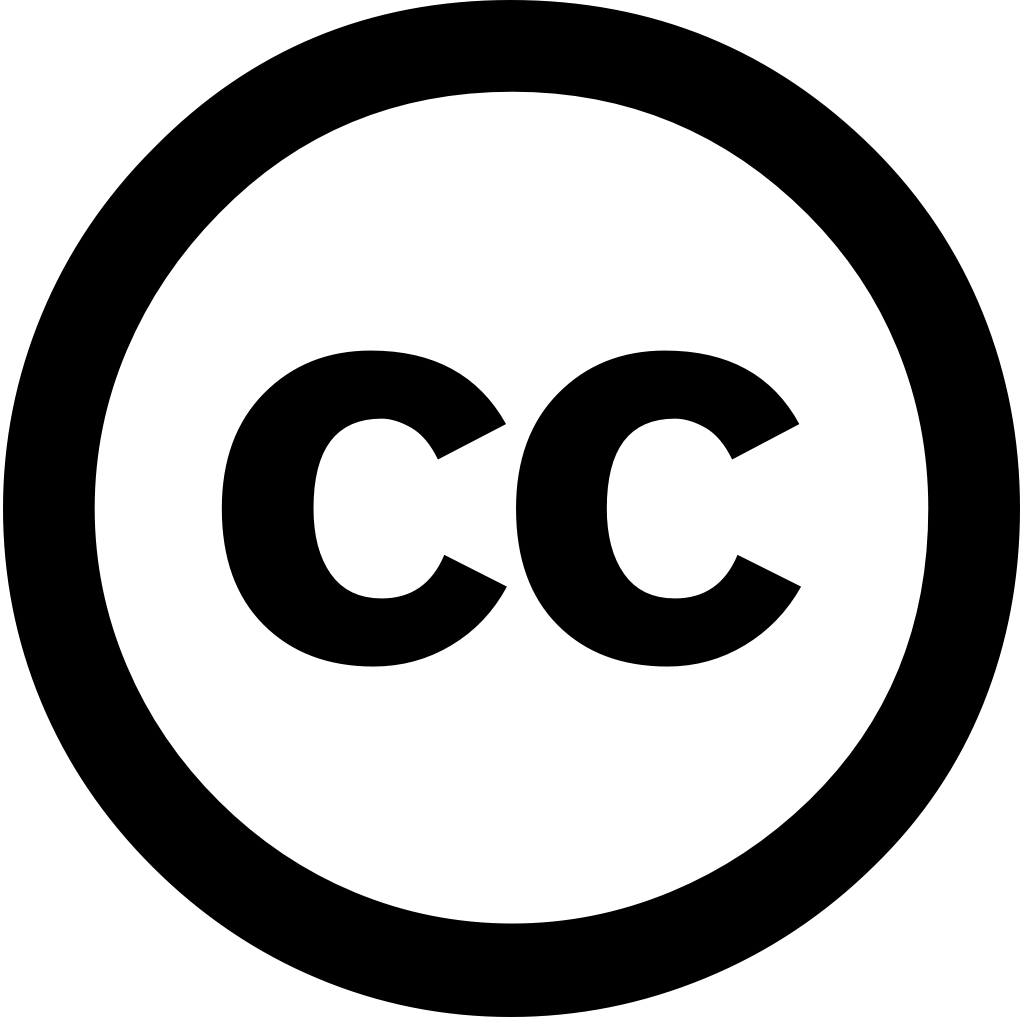
BMC Biology, Journal Year: 2025, Volume and Issue: 23(1)
Published: May 9, 2025
Circular RNAs (circRNAs) and microRNAs (miRNAs) interactions have essential implications in various biological processes diseases. Computational science approaches emerged as powerful tools for studying predicting these intricate molecular interactions, garnering considerable attention. Current methods face two significant limitations: the lack of precise interpretable models insufficient representation homogeneous heterogeneous molecules. We propose a novel method, MFERL, that addresses both limitations through multi-scale learning an explainable fine-grained model circRNA-miRNA (CMI). MFERL learns representations by aggregating node features interacting with features, well dual-convolution attention mechanisms contrastive to enhance features. utilize manifold-based method examine performance detail, revealing exhibits robust generalization, robustness, interpretability. Extensive experiments show outperforms state-of-the-art offers promising direction understanding CMI intrinsic mechanisms.
Language: Английский