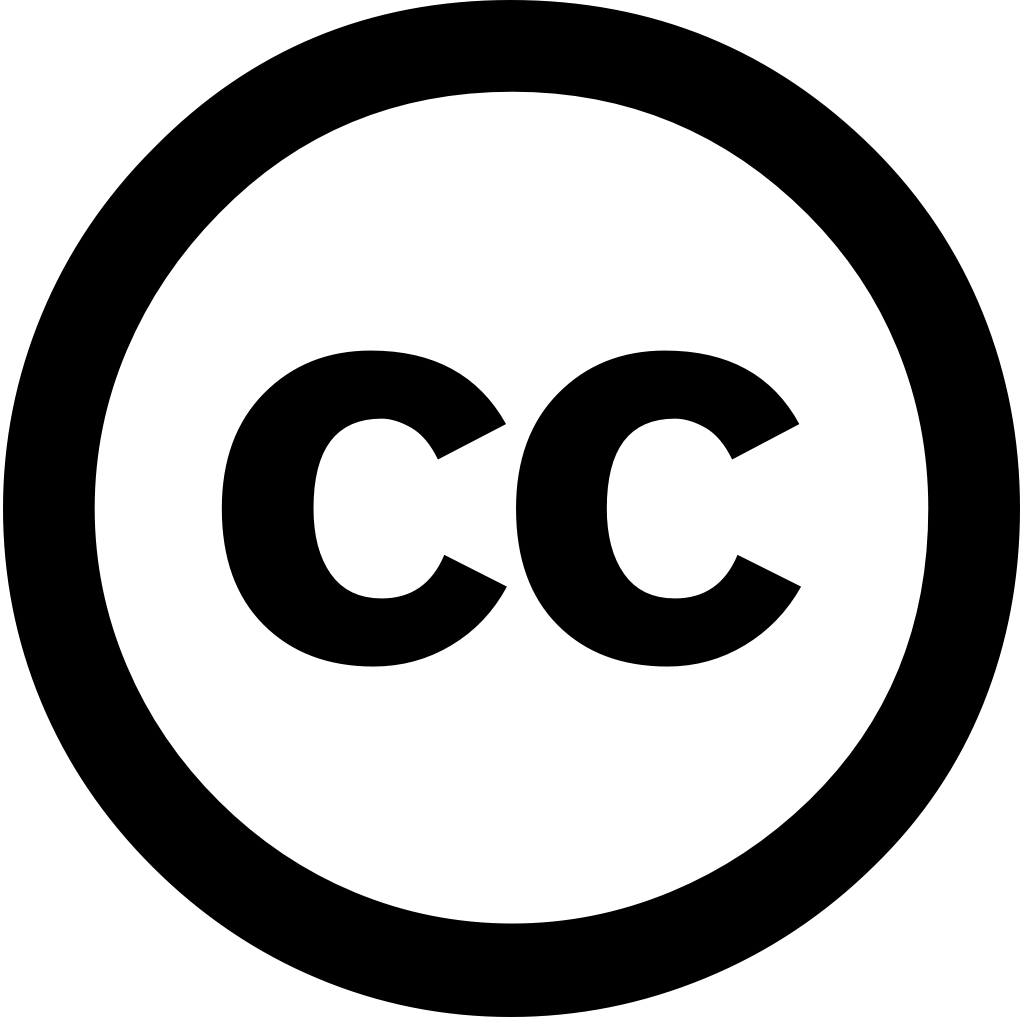
ACS Omega, Journal Year: 2025, Volume and Issue: unknown
Published: April 28, 2025
Language: Английский
ACS Omega, Journal Year: 2025, Volume and Issue: unknown
Published: April 28, 2025
Language: Английский
The Journal of Physical Chemistry A, Journal Year: 2025, Volume and Issue: unknown
Published: April 14, 2025
Infrared (IR) spectroscopy is a potent tool for identifying molecular structures and studying the chemical properties of compounds, hence, various theoretical approaches have been developed to simulate predict IR spectra. However, based on quantum calculations suffer from high computational cost (e.g., density functional theory, DFT) or insufficient accuracy semiempirical methods orders magnitude faster than DFT). Here, we introduce new approach, universal machine learning (ML) models AIQM series targeting CCSD(T)/CBS level, that can deliver spectra with close DFT (compared experiment) speed GFN2-xTB method. This approach harmonic oscillator approximation frequency scaling factors fitted experimental data. While benchmarks reported here are focused spectra, our implementation supports anharmonic simulations via dynamics VPT2. These implementations available in MLatom as described https://github.com/dralgroup/mlatom be performed online web browser.
Language: Английский
Citations
0Journal of Chemical Theory and Computation, Journal Year: 2025, Volume and Issue: unknown
Published: April 24, 2025
The development of machine learning interatomic potentials (MLIPs) has revolutionized computational chemistry by enhancing the accuracy empirical force fields while retaining a large speed-up compared to first-principles calculations. Despite these advancements, calculation Hessian matrices for systems remains challenging, in particular because analytical second-order derivatives are often not implemented. This necessitates use computationally expensive finite-difference methods, which can furthermore display low precision some cases. Automatic differentiation (AD) offers promising alternative reduce this effort and makes more efficient accurate. Here, we present implementation AD-based popular MACE equivariant graph neural network architecture. benefits method showcased via high-throughput prediction heat capacities porous materials with MACE-MP-0 foundation model. is essential precisely describing gas adsorption was previously possible only bespoke ML models or We find that availability accurate comparable zero-shot manner additionally allows investigation finite-size rounding errors data.
Language: Английский
Citations
0ACS Omega, Journal Year: 2025, Volume and Issue: unknown
Published: April 28, 2025
Language: Английский
Citations
0