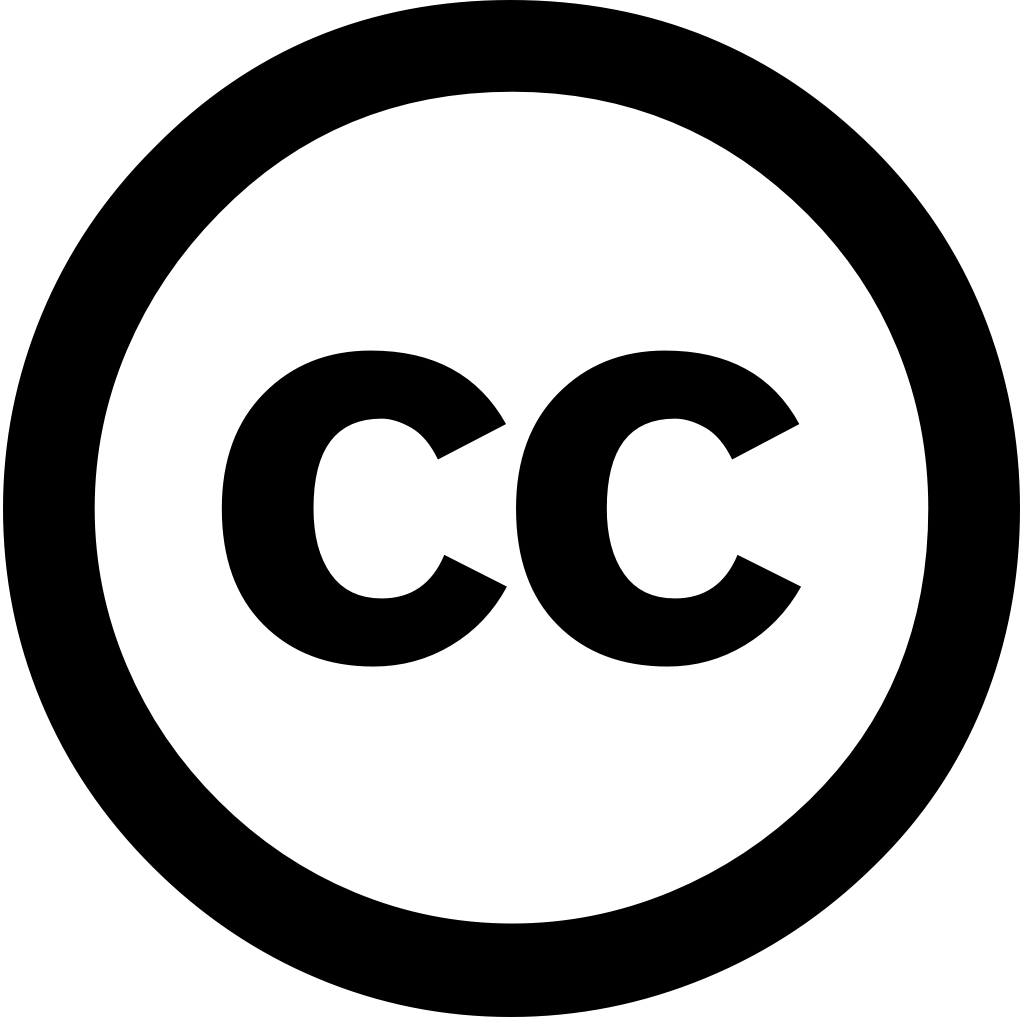
Computational Materials Science, Journal Year: 2024, Volume and Issue: 248, P. 113595 - 113595
Published: Dec. 10, 2024
Language: Английский
Computational Materials Science, Journal Year: 2024, Volume and Issue: 248, P. 113595 - 113595
Published: Dec. 10, 2024
Language: Английский
Wiley Interdisciplinary Reviews Computational Molecular Science, Journal Year: 2024, Volume and Issue: 14(5)
Published: Sept. 1, 2024
Abstract The design and discovery of new improved catalysts are driving forces for accelerating scientific technological innovations in the fields energy conversion, environmental remediation, chemical industry. Recently, use machine learning (ML) combination with experimental and/or theoretical data has emerged as a powerful tool identifying optimal various applications. This review focuses on how ML algorithms can be used computational catalysis materials science to gain deeper understanding relationships between properties their stability, activity, selectivity. development repositories, mining techniques, tools that navigate structural optimization problems highlighted, leading highly efficient sustainable future. Several data‐driven models commonly research diverse applications reaction prediction discussed. key challenges limitations using presented, which arise from catalyst's intrinsic complex nature. Finally, we conclude by summarizing potential future directions area ML‐guided catalyst development. article is categorized under: Structure Mechanism > Reaction Mechanisms Catalysis Data Science Artificial Intelligence/Machine Learning Electronic Theory Density Functional
Language: Английский
Citations
9Journal of Catalysis, Journal Year: 2025, Volume and Issue: unknown, P. 115983 - 115983
Published: Jan. 1, 2025
Language: Английский
Citations
0MetalMat, Journal Year: 2025, Volume and Issue: unknown
Published: Jan. 29, 2025
ABSTRACT High‐entropy materials (HEMs) have emerged as a pioneering paradigm in recent years, drawing substantial interest due to their unique combination of diverse elemental constituents and homogeneous solid‐solution structure. This novel material class not only opens up extensive potential for discovery through broad spectrum combinations but also facilitates fine‐tuning properties thanks its distinctive microstructural characteristics. HEMs garnered considerable attention across various applications, particularly catalysis. The virtually infinite variations compositional within these multi‐elemental systems enable meticulous optimization the catalytic performance. Additionally, high‐entropy structure potentially enhances structural, thermal, chemical stability, which is vital ensuring functionality under harsh conditions. Herein, we thoroughly explore exceptional attributes HEMs, designing strategies transition metal‐based catalysis, three major fields HEMs: electrocatalysis, photocatalysis, thermocatalysis. discussion aspires provide valuable perspectives into advancements innovations catalyst design development.
Language: Английский
Citations
0Chemical Physics Reviews, Journal Year: 2025, Volume and Issue: 6(1)
Published: March 1, 2025
Surfaces and interfaces play key roles in chemical material science. Understanding physical processes at complex surfaces is a challenging task. Machine learning provides powerful tool to help analyze accelerate simulations. This comprehensive review affords an overview of the applications machine study systems materials. We categorize into following broad categories: solid–solid interface, solid–liquid liquid–liquid surface solid, liquid, three-phase interfaces. High-throughput screening, combined first-principles calculations, force field accelerated molecular dynamics simulations are used rational design such as all-solid-state batteries, solar cells, heterogeneous catalysis. detailed information on for
Language: Английский
Citations
0Advanced Functional Materials, Journal Year: 2025, Volume and Issue: unknown
Published: March 16, 2025
Abstract High‐entropy nanoparticles (HENPs) present a vast opportunity for the development of advanced electrocatalysts. The optimization their chemical compositions, including careful selection and combination elements, is critical to tailoring HENPs specific catalytic processes. To reduce extensive experimental effort involved in composition optimization, active learning techniques can be utilized predict suggest materials with enhanced electrocatalytic activity. In this study, sub‐2 nm high‐entropy catalysts incorporating eight transition metal elements are developed through an workflow aimed at identifying optimal compositions. Using initial data, approach successfully guided discovery new octonary HENP catalyst state‐of‐the‐art performance hydrogen evolution reaction (HER). Catalyst improved within prediction uncertainty machine model. For oxygen (OER), however, model demonstrated limited predictive accuracy, leading assessment workflow's boundaries. These findings underscore how integration curated data accelerate electrocatalyst discovery, while also highlighting areas further refinement.
Language: Английский
Citations
0Small Methods, Journal Year: 2024, Volume and Issue: unknown
Published: Nov. 10, 2024
The complex compositional space of high entropy alloys (HEAs) has shown a great potential to reduce the cost and further increase catalytic activity for hydrogen evolution reaction (HER) by optimization. Without uncovering specifics HER mechanism on given HEA surface, it is unfeasible apply modifications enhance performance save costs. In this work, combination density functional theory Bayesian machine learning used demonstrate unique IrPdPtRhRu catalysts HER. At coverage underpotential-deposited hydrogen, d-band investigation active sites surface conducted elucidate superior through electronic interactions between elements. low coverage, novel with oversampling approach then outlined optimize composition improvement reduction. This proves more efficacious efficient yields higher-quality structures less training set bias compared neural-network proposed optimization theoretically outperforms benchmark Pt catalysts' overpotential ≈40% at 15% reduced synthesis comparing equiatomic ratio HEA.
Language: Английский
Citations
2Langmuir, Journal Year: 2024, Volume and Issue: unknown
Published: July 13, 2024
The electrocatalytic nitrate reduction to ammonia holds significant values for water remediations and energy applications, which quests the development of highly effective catalysts with considerable stability selectivity. Recently, high-entropy alloys (HEAs) are attracting growing attention processes. Nonetheless, studies HEA-based still at early stage, it remains unclear how HEA compositions affect adsorption activation reaction intermediates. Herein, high-throughput density functional theory (DFT) calculations were integrated machine learning investigate dependence on FeCoNiCuZn structures. In particular, a total 1268 different structures sampled constructed from multidimensional configuration space, followed by DFT Gibbs free (i.e., Δ
Language: Английский
Citations
1Computational Materials Science, Journal Year: 2024, Volume and Issue: 248, P. 113595 - 113595
Published: Dec. 10, 2024
Language: Английский
Citations
1