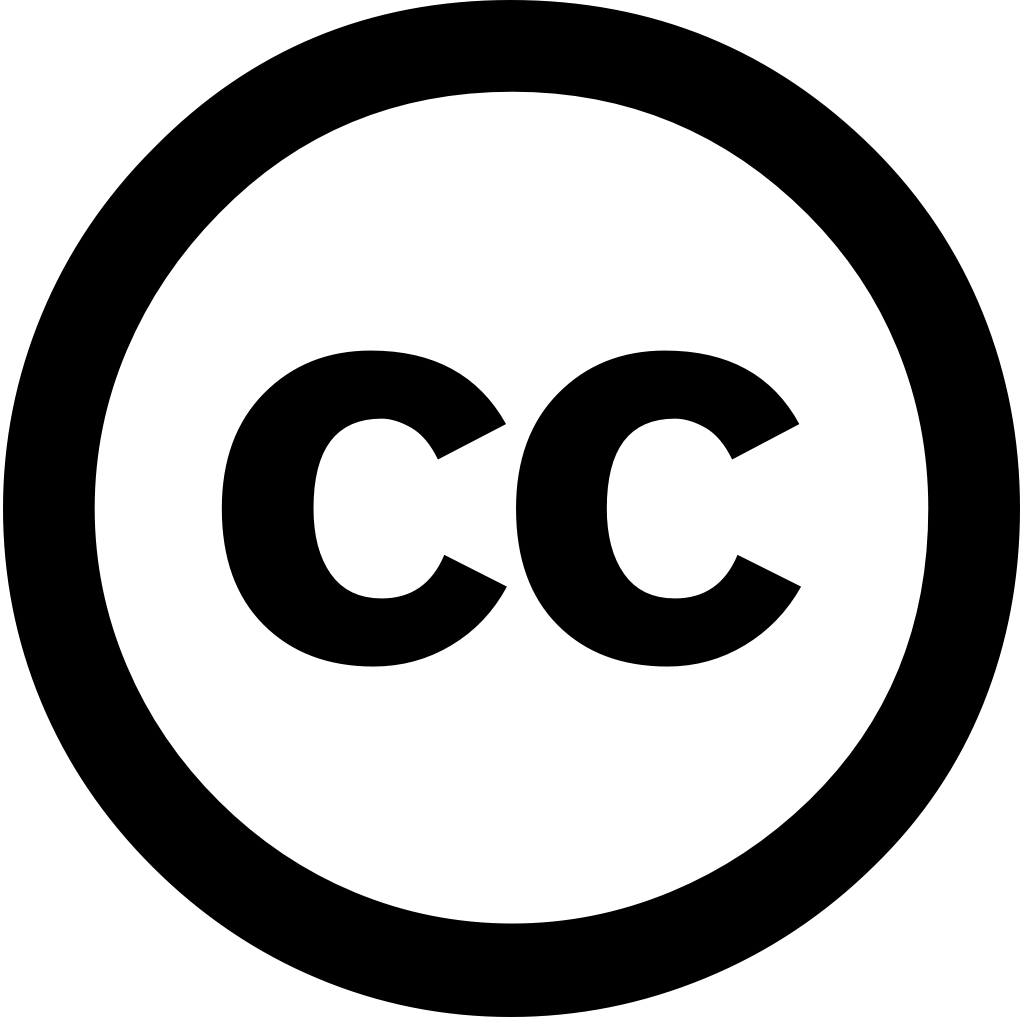
Published: Dec. 4, 2023
<p>Monkeypox has recently emerged as a public health emergency with rising cases worldwide. Early clinical diagnosis is challenging due to symptom overlap other diseases, but characteristic skin lesions provide distinguishing visual cues. This work proposes deep convolutional neural network (CNN) tailored for automated monkeypox screening from lesion images. A dataset of over 3000 dermatological images was compiled, data augmentation enhance diversity. The CNN architecture comprised blocks feature extraction and dense layers classification. Rigorous training cross-validation were conducted 100 epochs optimize model performance. On an unseen test set, the achieved 86.87\% accuracy in classifying lesions, 94\% precision, 79\% recall 86\% F1-score. These metrics better than baseline models, indicating reliable potential. Though overlooked some atypical presentations, successes showcase utility mass case-finding. As monitoring intensifies, robust computer vision approaches can assist clinicians through explainable, real-time forecasts. Prospective validation across demographics integration workflows warranted before full-scale deployment. Overall, study demonstrates learning's promise tackling outbreak enhanced diagnosis.</p>
Language: Английский