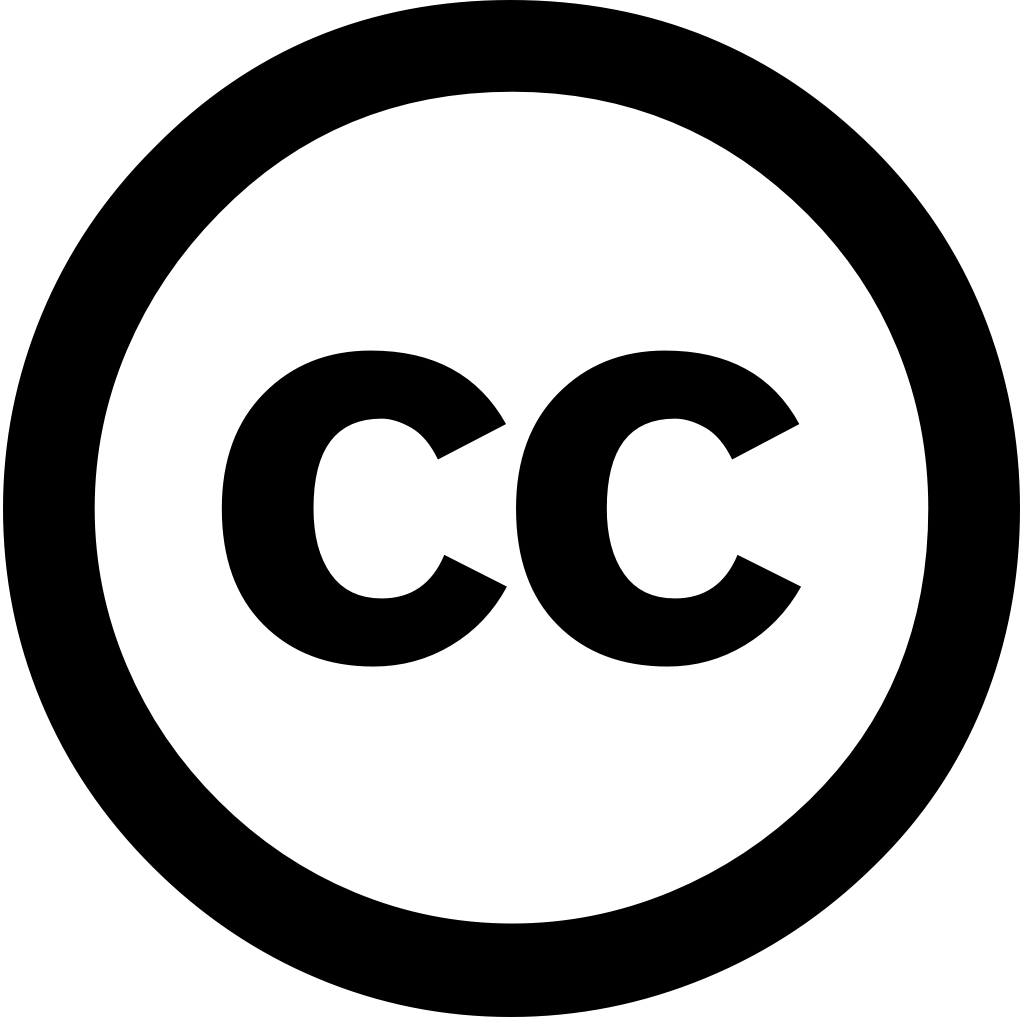
Journal of Magnesium and Alloys, Journal Year: 2024, Volume and Issue: unknown
Published: Dec. 1, 2024
Language: Английский
Journal of Magnesium and Alloys, Journal Year: 2024, Volume and Issue: unknown
Published: Dec. 1, 2024
Language: Английский
Nature Communications, Journal Year: 2024, Volume and Issue: 15(1)
Published: June 25, 2024
Abstract Data scarcity is one of the critical bottlenecks to utilizing machine learning in material discovery. Transfer can use existing big data assist property prediction on small sets, but premise that there must be a strong correlation between large and sets. To extend its applicability scenarios with different properties materials, here we develop hybrid framework combining adversarial transfer expert knowledge, which enables direct carrier mobility two-dimensional (2D) materials using knowledge learned from bulk effective mass. Specifically, training ensures only common 2D extracted while incorporated further improve accuracy generalizability. Successfully, mobilities are predicted over 90% crystal structure, 21 semiconductors far exceeding silicon suitable bandgap successfully screened out. This work simultaneous cross-property cross-material scenarios, providing an tool predict intricate limited data.
Language: Английский
Citations
10Chemical Physics Reviews, Journal Year: 2025, Volume and Issue: 6(1)
Published: March 1, 2025
Surfaces and interfaces play key roles in chemical material science. Understanding physical processes at complex surfaces is a challenging task. Machine learning provides powerful tool to help analyze accelerate simulations. This comprehensive review affords an overview of the applications machine study systems materials. We categorize into following broad categories: solid–solid interface, solid–liquid liquid–liquid surface solid, liquid, three-phase interfaces. High-throughput screening, combined first-principles calculations, force field accelerated molecular dynamics simulations are used rational design such as all-solid-state batteries, solar cells, heterogeneous catalysis. detailed information on for
Language: Английский
Citations
1Physical Review Materials, Journal Year: 2025, Volume and Issue: 9(2)
Published: Feb. 18, 2025
Language: Английский
Citations
0The Journal of Physical Chemistry C, Journal Year: 2025, Volume and Issue: unknown
Published: Feb. 27, 2025
Language: Английский
Citations
0Materia Japan, Journal Year: 2025, Volume and Issue: 64(3), P. 184 - 189
Published: Feb. 28, 2025
Language: Английский
Citations
0Acta Materialia, Journal Year: 2025, Volume and Issue: unknown, P. 121034 - 121034
Published: April 1, 2025
Language: Английский
Citations
0The Journal of Physical Chemistry Letters, Journal Year: 2025, Volume and Issue: unknown, P. 5244 - 5251
Published: May 18, 2025
Machine learning (ML) approaches have become ubiquitous in the search for new materials recent years. Bayesian optimization (BO) based on Gaussian processes (GPs) has a widely recognized approach material exploration. However, feature engineering critical impacts efficiency of GP-based BO, because GPs cannot automatically generate descriptors. To address this limitation, study applies deep kernel (DKL), which combines neural network with GP, to BO. The DKL model was comparable or significantly better than that standard GP data set 922 oxide sets, covering band gaps, ionic dielectric constants, and effective masses electrons, as well experimental gaps 610 hybrid organic-inorganic perovskite alloys. When searching alloy highest Curie temperature among 4560 alloys, outperformed strongly correlated descriptor could be directly utilized. Additionally, supports transfer learning, further enhances its efficiency. Thus, we believe BO paves way exploring diverse spaces more effectively GPs.
Language: Английский
Citations
0Science and Technology of Advanced Materials, Journal Year: 2024, Volume and Issue: 25(1)
Published: Nov. 4, 2024
Computational approaches using theoretical calculations and data scientific methods have become increasingly important in materials science technology, with the development of relevant methodologies algorithms, availability large data, enhancement computer performance. As reviewed herein, we developed computational for design prediction inorganic a particular focus on exploration semiconductors dielectrics. High-throughput first-principles are used to systematically accurately predict local atomic electronic structures polarons, point defects, surfaces, interfaces, as well bulk fundamental properties. Machine learning techniques utilized efficiently various material properties, construct phase diagrams, search satisfying target These elucidated mechanisms behind functionalities explored promising combination synthesis, characterization, device fabrication. Examples include ternary nitride potential optoelectronic photovoltaic applications, phosphide optimization heterointerfaces toward improvement phosphide-based cells, discovery ferroelectricity layered perovskite oxides understanding its origin, all which demonstrate effectiveness our computer-aided research.
Language: Английский
Citations
1Journal of Magnesium and Alloys, Journal Year: 2024, Volume and Issue: unknown
Published: Dec. 1, 2024
Language: Английский
Citations
0