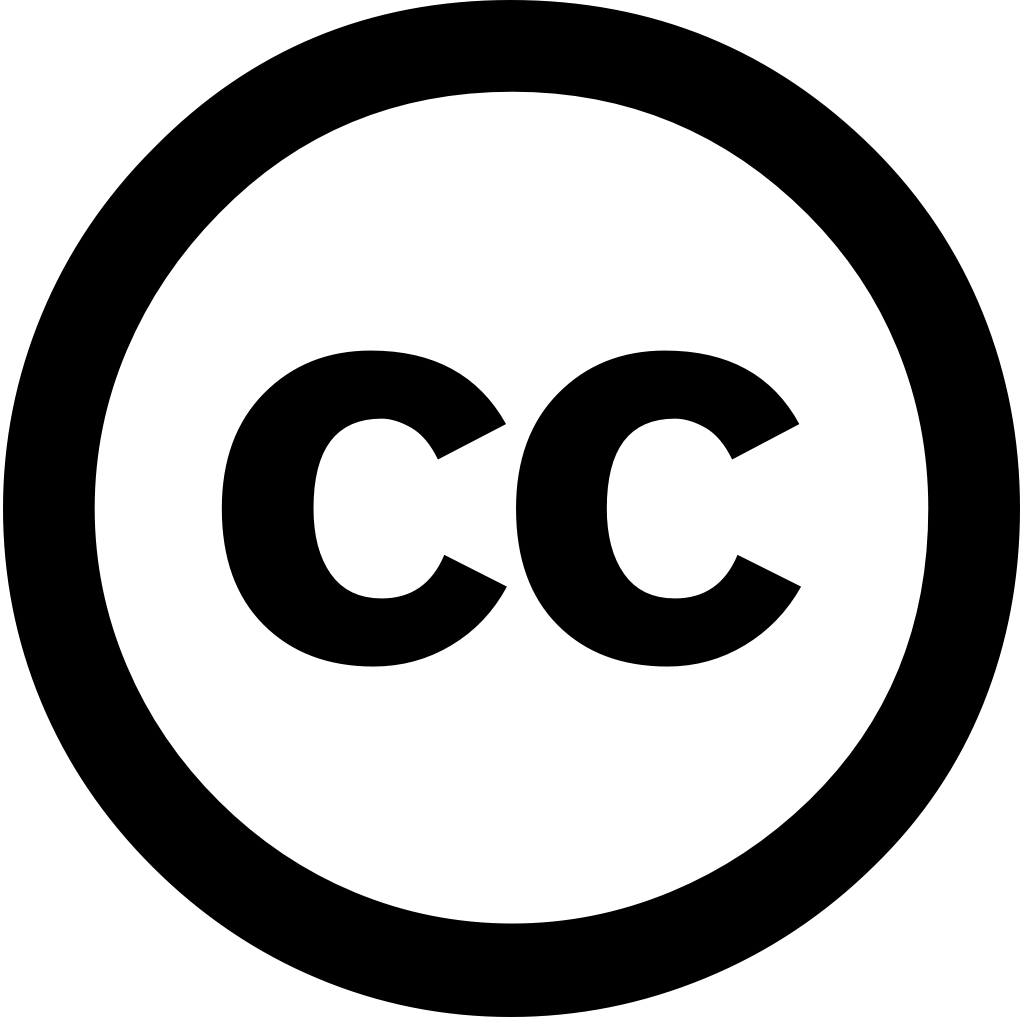
Precision Chemistry, Journal Year: 2024, Volume and Issue: unknown
Published: Nov. 5, 2024
Language: Английский
Precision Chemistry, Journal Year: 2024, Volume and Issue: unknown
Published: Nov. 5, 2024
Language: Английский
Journal of Chemical Theory and Computation, Journal Year: 2025, Volume and Issue: unknown
Published: Jan. 3, 2025
Electron density is a fundamental quantity that can in principle determine all ground state electronic properties of given system. Although machine learning (ML) models for electron based on either an atom-centered basis or real-space grid have been proposed, the demand number high-order functions points enormous. In this work, we propose efficient grid-point sampling strategy combines targeted favoring large and screening associated with linearly independent atomic features. This new integrated field-induced recursively embedded atom neural network model to develop grid-based ML its response electric field. approach applied QM9 molecular data set, H2O/Pt(111) interfacial system, Au(100) electrode, Au nanoparticle under The training found be much smaller than previous models, while yielding comparably accurate predictions entire grid. resultant machine-learned enables us properly partition partial charge onto each analyze variation upon proton transfer machine-learning allows predict electrostatic potential change induced by field electrode nanoparticle.
Language: Английский
Citations
0ACS Catalysis, Journal Year: 2025, Volume and Issue: 15(3), P. 1616 - 1634
Published: Jan. 15, 2025
The production of many bulk chemicals relies on heterogeneous catalysis. rational design or improvement the required catalysts critically depends insights into underlying mechanisms atomic scale. In recent years, substantial progress has been made in applying advanced experimental techniques to complex catalytic reactions operando, but order achieve a comprehensive understanding, additional information from computer simulations is indispensable cases. particular, ab initio molecular dynamics (AIMD) become an important tool explicitly address atomistic level structure, dynamics, and reactivity interfacial systems, high computational costs limit applications systems consisting at most few hundred atoms for simulation times up tens picoseconds. Rapid advances development modern machine learning potentials (MLP) now offer promising approach bridge this gap, enabling with accuracy small fraction costs. Perspective, we provide overview current state art MLPs relevant catalysis along discussion prospects use science years come.
Language: Английский
Citations
0Chemical Society Reviews, Journal Year: 2025, Volume and Issue: unknown
Published: Jan. 1, 2025
This review offers a comprehensive overview of the development machine learning potentials for molecules, reactions, and materials over past two decades, evolving from traditional models to state-of-the-art.
Language: Английский
Citations
0Published: Jan. 1, 2025
Language: Английский
Citations
0Published: Jan. 1, 2025
Language: Английский
Citations
0Precision Chemistry, Journal Year: 2024, Volume and Issue: unknown
Published: Nov. 5, 2024
Language: Английский
Citations
0