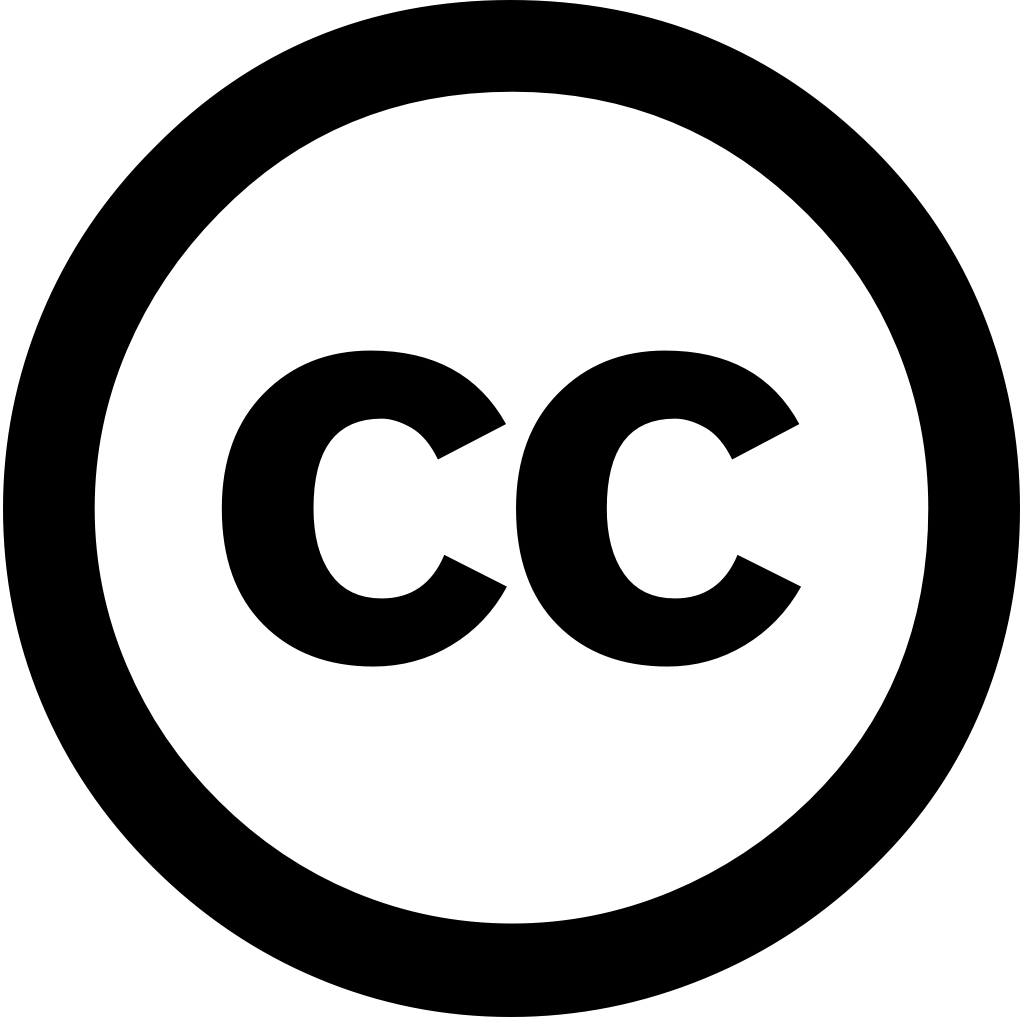
Water Research, Journal Year: 2024, Volume and Issue: 268, P. 122779 - 122779
Published: Nov. 9, 2024
Language: Английский
Water Research, Journal Year: 2024, Volume and Issue: 268, P. 122779 - 122779
Published: Nov. 9, 2024
Language: Английский
Sustainability, Journal Year: 2023, Volume and Issue: 15(13), P. 10543 - 10543
Published: July 4, 2023
Floods are a devastating natural calamity that may seriously harm both infrastructure and people. Accurate flood forecasts control essential to lessen these effects safeguard populations. By utilizing its capacity handle massive amounts of data provide accurate forecasts, deep learning has emerged as potent tool for improving prediction control. The current state applications in forecasting management is thoroughly reviewed this work. review discusses variety subjects, such the sources utilized, models used, assessment measures adopted judge their efficacy. It assesses approaches critically points out advantages disadvantages. article also examines challenges with accessibility, interpretability models, ethical considerations prediction. report describes potential directions deep-learning research enhance predictions Incorporating uncertainty estimates into integrating many sources, developing hybrid mix other methodologies, enhancing few these. These goals can help become more precise effective, which will result better plans forecasts. Overall, useful resource academics professionals working on topic management. reviewing art, emphasizing difficulties, outlining areas future study, it lays solid basis. Communities prepare destructive floods by implementing cutting-edge algorithms, thereby protecting people infrastructure.
Language: Английский
Citations
104Journal of Hydrology, Journal Year: 2025, Volume and Issue: unknown, P. 132697 - 132697
Published: Jan. 1, 2025
Language: Английский
Citations
5Water Research, Journal Year: 2023, Volume and Issue: 250, P. 121018 - 121018
Published: Dec. 14, 2023
Language: Английский
Citations
39Environmental Research, Journal Year: 2023, Volume and Issue: 238, P. 117268 - 117268
Published: Sept. 28, 2023
Language: Английский
Citations
36Environmental Modelling & Software, Journal Year: 2023, Volume and Issue: 161, P. 105623 - 105623
Published: Jan. 11, 2023
Language: Английский
Citations
32Journal of Water Resources Planning and Management, Journal Year: 2023, Volume and Issue: 149(7)
Published: May 12, 2023
In recent years, a vision has been shared of how artificial intelligence (AI) can optimize the increasingly complex operations drinking water utilities. However, it unclear if and utilities use technology. Here, we surveyed simple random sample 49 large US to provide snapshot progress. We found that 12 them (24%) have used some form AI. Of those not, majority plan or may AI in next 5 years. The reported uses were experimental, manual, partial models rather than fully integrated, ongoing applications. Respondents are motivated for improving quality, detecting leaks, automating systems, but they cited payback uncertainty lack expertise as most common barriers implementation. To better demonstrate join other tools available assist human operators, researchers should focus on top motivations identified here partner with convincing case studies full-scale projects. These steps will support further responsible adoption utility part more sustainable communities.
Language: Английский
Citations
32Wiley Interdisciplinary Reviews Water, Journal Year: 2023, Volume and Issue: 10(3)
Published: Jan. 21, 2023
Abstract Sewer systems are an essential part of sanitation infrastructure for protecting human and ecosystem health. Initially, they were used to solely convey stormwater, but over time municipal sewage was discharged these conduits transformed them into combined sewer (CSS). Due climate change rapid urbanization, no longer sufficient overflow in wet weather conditions. Mechanistic data‐driven models have been frequently research on (CSO) management integrating low‐impact development gray‐green infrastructures. Recent advances measurement, communication, computation technologies simplified data collection methods. As a result, such as artificial intelligence (AI), geographic information system, remote sensing can be integrated CSO stormwater the smart city digital twin concepts build climate‐resilient infrastructures services. Therefore, CSS is now both technically economically feasible tackle challenges ahead. This review article explores characteristics associated impact receiving waterbodies, evaluates suitable management, presents studies including above‐mentioned context management. Although integration all has big potential, further required achieve AI‐controlled robust agile mitigation. categorized under: Engineering Water > Sustainable Science Environmental Change
Language: Английский
Citations
23Cambridge Prisms Water, Journal Year: 2023, Volume and Issue: 1
Published: Jan. 1, 2023
Abstract The potential of artificial intelligence (AI) in water management is widely recognised by research and practice communities alike, with an increasing number applications showed tackling supply, stormwater wastewater challenges. However, there a critical knowledge gap understanding the fundamental role AI development urban infrastructure (UWI). This review aimed to provide analysis how could be aligned support future UWI systems. Four types analytics – descriptive, diagnostic, predictive prescriptive are discussed linked improvement performance systems from three categories: reliability, resilience sustainability. It envisioned that technology will play pivotal transitioning through underpinning five pathways: decentralisation, circular economy, greening, decarbonisation automation. barriers improving adoption real world also highlighted four dimensions: cyber-physical infrastructure, institutional governance, social-economic technological wider society. Embedding pathways can ensure AI-empowered deployed equitable responsible way improve sustainability
Language: Английский
Citations
23International Journal of Applied Earth Observation and Geoinformation, Journal Year: 2024, Volume and Issue: 131, P. 103972 - 103972
Published: June 25, 2024
Given the increased incidence of pluvial floods due to climate change and urbanization, demand for highly efficient accurate modeling within urban drainage systems has intensified, making machine learning deep techniques increasingly popular. Nonetheless, these data-driven approaches face challenges in adequately capturing interpreting dynamic process-evolving features, especially spatiotemporal effects emanating from manholes during waterlogging events. To address issues, this study proposes a general framework that extracts using spatial Durbin model, integrates such with four models (i.e., artificial neural network, Bayesian network (BNN), light gradient boosting machine, long short-term memory network), clarifies decision-making processes best model by employing Shapley Additive Explanations (SHAP) method. The results indicate (1) BNN (BNNST) not only outperforms other benchmark but also provides forecasts quantifiable uncertainties; (2) compared original enhance models' understanding flooding dynamics, thereby improving predictive precision; (3) comprise roughly 14 % contributions BNNST's output, as interpreted SHAP-based explanations; (4) incorporating interpretability into technique underscores trustworthiness explanations at varying confidence levels, deepening processes.
Language: Английский
Citations
11The Science of The Total Environment, Journal Year: 2024, Volume and Issue: 926, P. 171793 - 171793
Published: March 20, 2024
Language: Английский
Citations
10