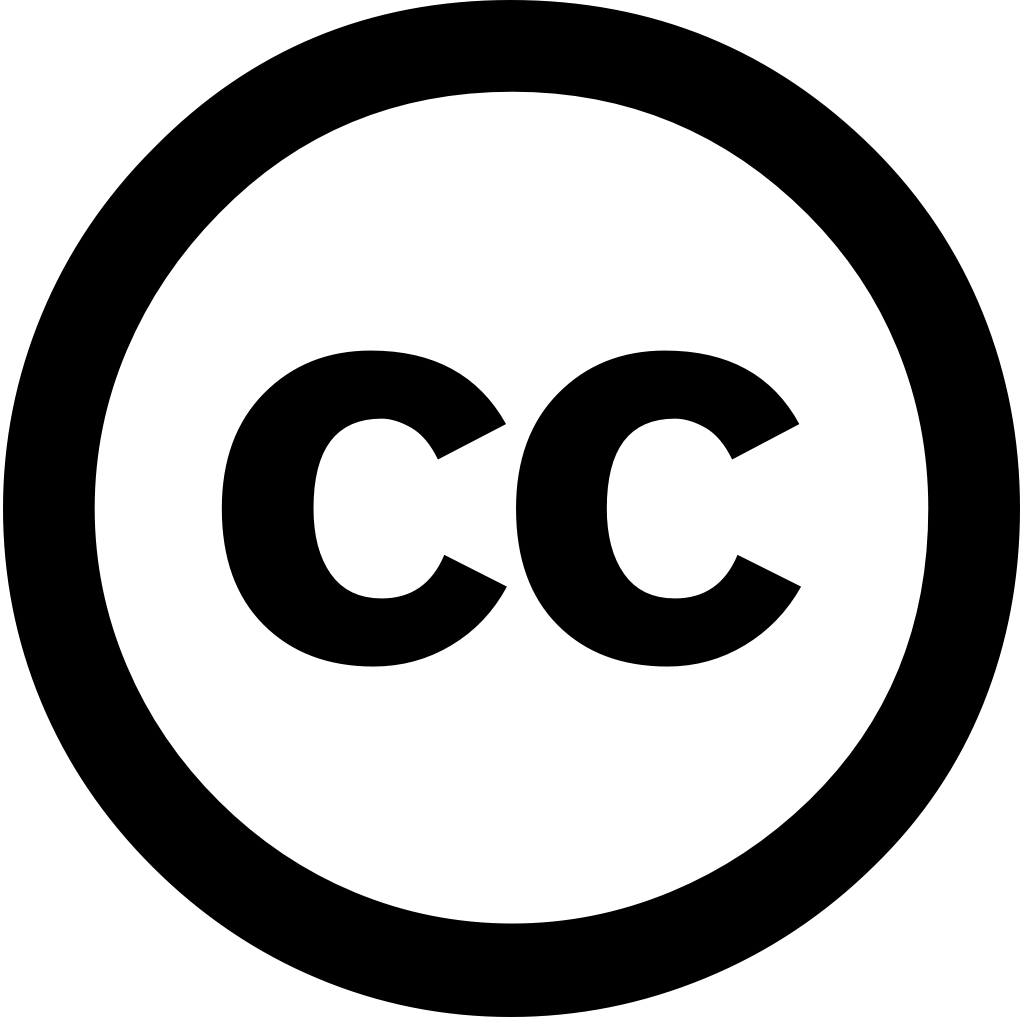
EarthArXiv (California Digital Library), Journal Year: 2022, Volume and Issue: unknown
Published: Oct. 15, 2022
Growth and contraction of headwater stream networks determine the extent quality ecologically critical habitat, open a window into storage dynamics catchments. A fundamental challenge is observation process itself: wetted channel highly dynamic in space time, with length sometimes varying by orders magnitude over course single storm event To date, observational datasets are produced from boots-on-the-ground campaigns, drone imaging, or flow presence sensors, which often laborious limited their spatial temporal extents. Here, we evaluate high-resolution, multi-band satellite imagery as means to detect via machine learning methods trained using existing surveys. Even where features smaller than resolution imagery, absence surface water may nevertheless be imprinted upon spectral signature an individual pixel. We leverage surveys at two oak savanna catchments northern California minimal riparian canopy cover due small subsurface capacity saturation overland flow. train random forest model on high-resolution ($\sim$5 m pixel) RapidEye captured contemporaneously Withheld test data indicates prediction accuracy wet vs. dry $>$91\%. This predictive ability used produce length-discharge (L-Q) relations calculate spatially distributed estimates hyporheic exchange. sharp break properties occurs transition main stem channels lower order tributaries, resulting stepped L-Q relationship that cannot traditionally power law models. Remotely sensed powerful tool for producing maps high ($\sim$10 this study $>$ 0.01 km$^2$ contributing area).
Language: Английский