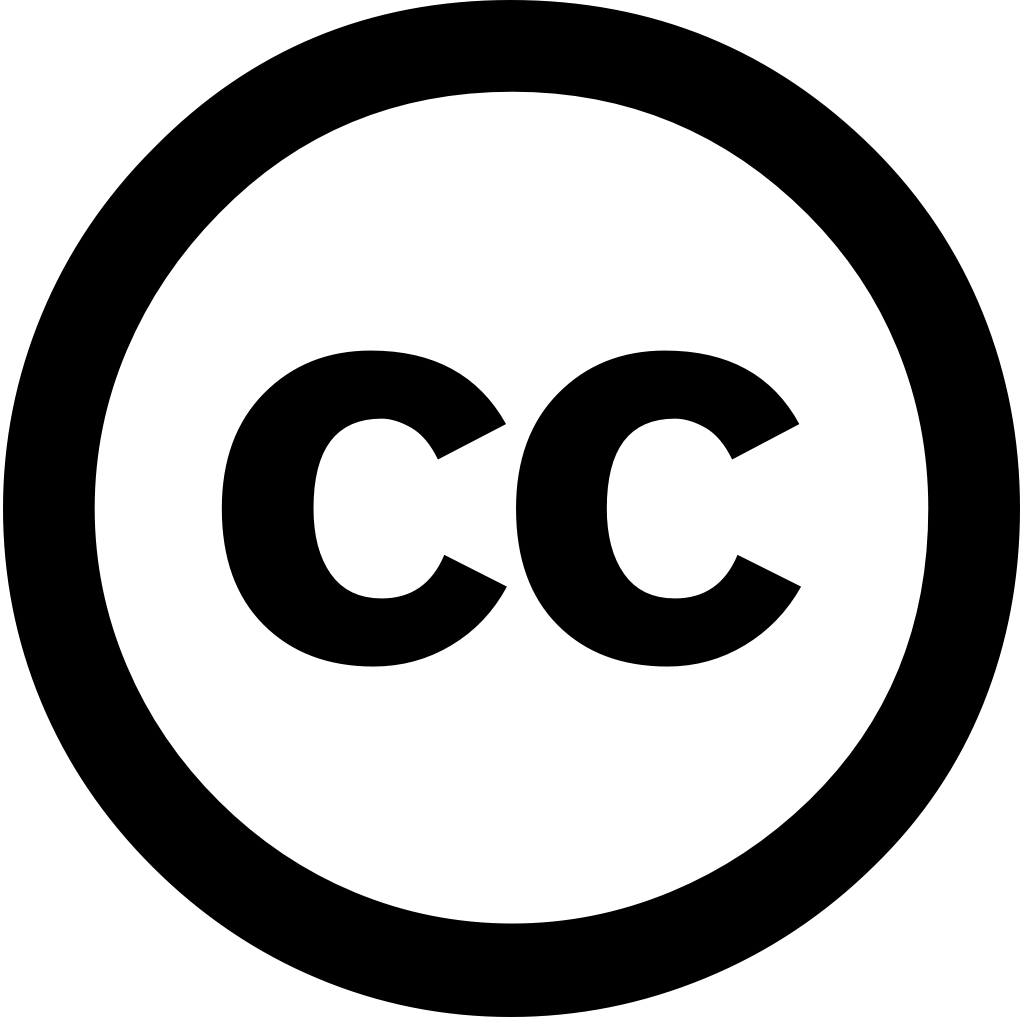
Research Square (Research Square), Journal Year: 2024, Volume and Issue: unknown
Published: April 8, 2024
Language: Английский
Research Square (Research Square), Journal Year: 2024, Volume and Issue: unknown
Published: April 8, 2024
Language: Английский
npj Climate and Atmospheric Science, Journal Year: 2025, Volume and Issue: 8(1)
Published: May 7, 2025
Language: Английский
Citations
0Nature Communications, Journal Year: 2024, Volume and Issue: 15(1)
Published: Aug. 28, 2024
We present GPSat; an open-source Python programming library for performing efficient interpolation of non-stationary satellite altimetry data, using scalable Gaussian process techniques. use GPSat to generate complete maps daily 50 km-gridded Arctic sea ice radar freeboard, and find that, relative a previous scheme, offers 504 × computational speedup, with less than 4 mm difference on the derived freeboards average. then demonstrate scalability through freeboard at 5 km resolution, Sea-Level Anomalies (SLA) resolution altimeter footprint. Interpolated show strong agreement airborne data (linear correlation 0.66). Footprint-level SLA also shows improvements in predictive skill over linear regression. In this work, we suggest that could overcome bottlenecks faced many altimetry-based routines, hence advance critical understanding ocean variability short spatio-temporal scales.
Language: Английский
Citations
2Research Square (Research Square), Journal Year: 2024, Volume and Issue: unknown
Published: April 8, 2024
Language: Английский
Citations
1