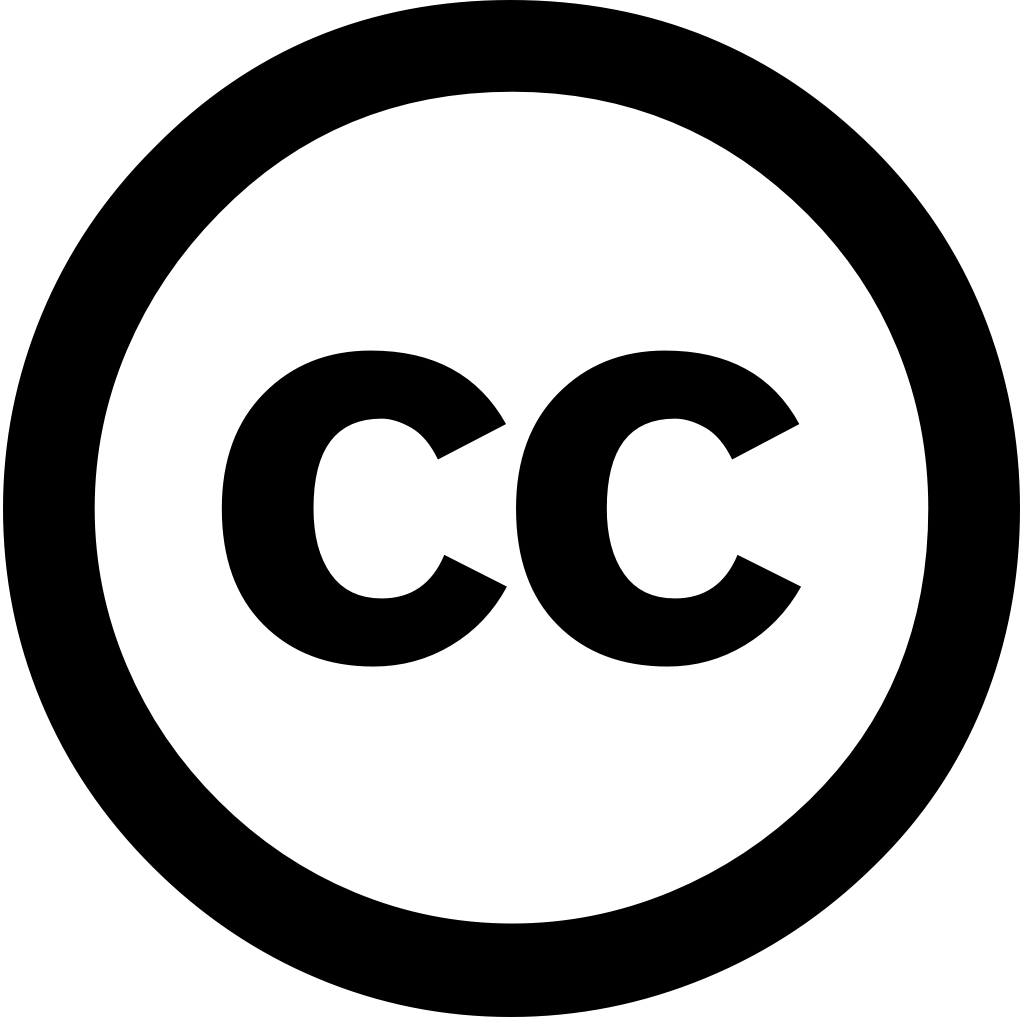
Remote Sensing, Journal Year: 2025, Volume and Issue: 17(8), P. 1333 - 1333
Published: April 8, 2025
The Qinghai–Tibet Plateau (QTP), a critical hydrological regulator for Asia through its extensive glacier systems, high-altitude lakes, and intricate network of rivers, exhibits amplified sensitivity to climate-driven alterations in precipitation regimes ice mass balance. While the Gravity Recovery Climate Experiment (GRACE) Follow-On (GRACE-FO) missions have revolutionized monitoring terrestrial water storage anomalies (TWSAs) across this hydrologically sensitive region, spatial resolution limitations (3°, equivalent ~300 km) constrain process-scale analysis, compounded by mission temporal discontinuity (data gaps). In study, we present novel downscaling framework integrating gap compensation refinement 0.25° Gated Recurrent Unit (GRU) neural networks, an architecture optimized univariate time series modeling. Through assimilation multi-source parameters (glacier flux, cryosphere–precipitation interactions, land surface processes), GRU-based result resolves nonlinear dynamics while bridging inter-mission observational gaps. Grid-level implementation preserves conservation principles heterogeneous topographies, successfully reconstructing seasonal-to-interannual TWSA variability also long-term trends. Comparative validation against GRACE mascon solutions process-based models demonstrates enhanced capacity resolving sub-basin heterogeneity. This GRU-derived high-resolution is especially valuable dissecting local areas such as Brahmaputra Basin, where complex cycling can affect downstream security. Our study provides transferable methodologies mountainous hydrogeodesy analysis under evolving climate regimes. Future enhancements physics-informed deep learning next-generation climatology–hydrology–gravimetry synergy (e.g., observations models) could further uncertainties extreme elevation zones, advancing predictive understanding Asia’s tower sustainability.
Language: Английский