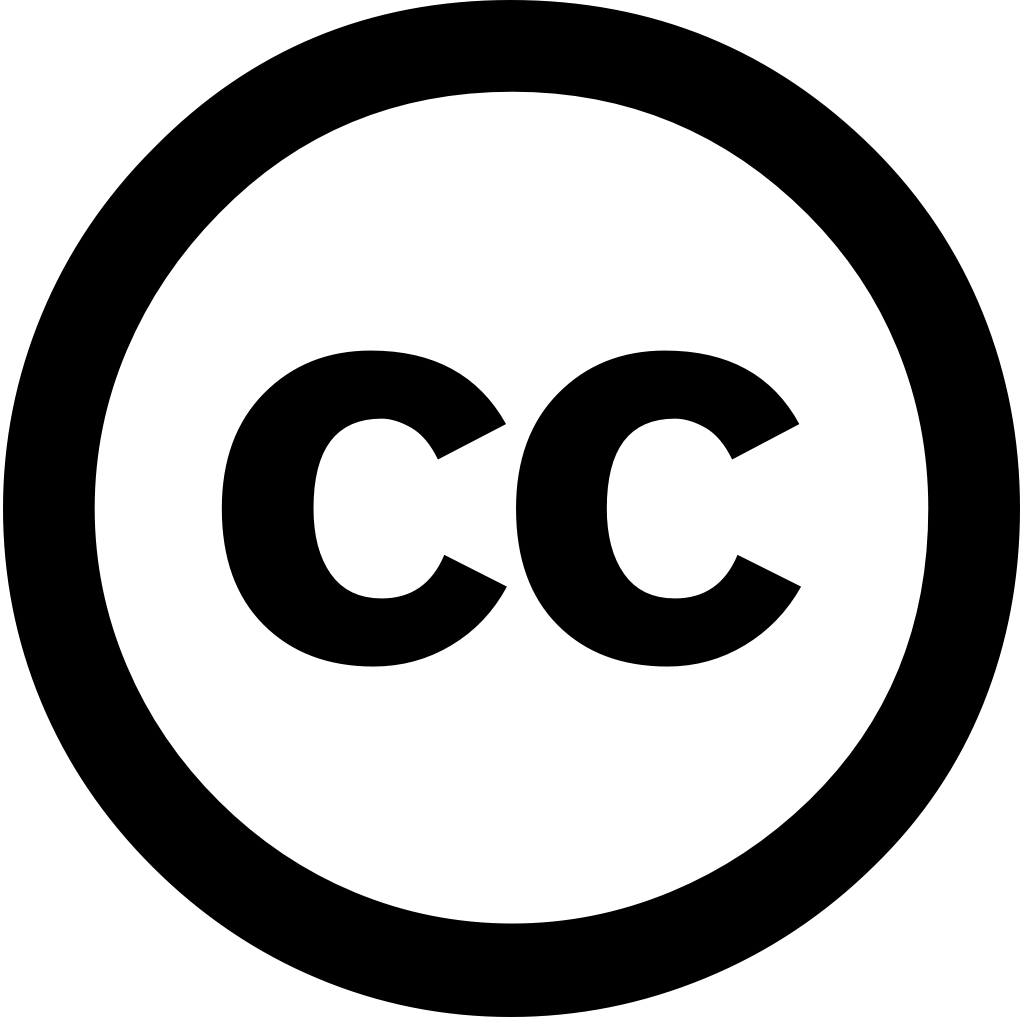
Nature Communications, Journal Year: 2025, Volume and Issue: 16(1)
Published: March 25, 2025
Partitioning precipitation into rain and snow with near-surface meteorology is a well-known challenge. However, whether limit exists to its potential performance remains unknown. Here, we evaluate this possibility by applying set of benchmark phase partitioning methods plus three machine learning (ML) models (an artificial neural network, random forest, XGBoost) two independent datasets: 38.5 thousand crowdsourced observations 17.8 million synoptic reports. The ML provide negligible improvements over the best benchmarks, increasing accuracy only up 0.6% reducing biases -4.7%. fail identify mixed sub-freezing rainfall events, while expressing their worst values from 1.0 °C–2.5 °C. A cause these shortcomings air temperature overlap in distributions (peaking between °C–1.6 °C), which expresses significant negative relationship (p < 0.0005) accuracy. Thus, meteorological characteristics are similar at temperatures slightly above freezing associated decreasing performance. We suggest researchers switch focus marginally improving inherently limited using creating new that assimilate novel data sources—e.g., observations. This paper shows used partition fundamentally flawed near point. Machine cannot overcome dip existing traditional techniques, highlighting need for approaches.
Language: Английский