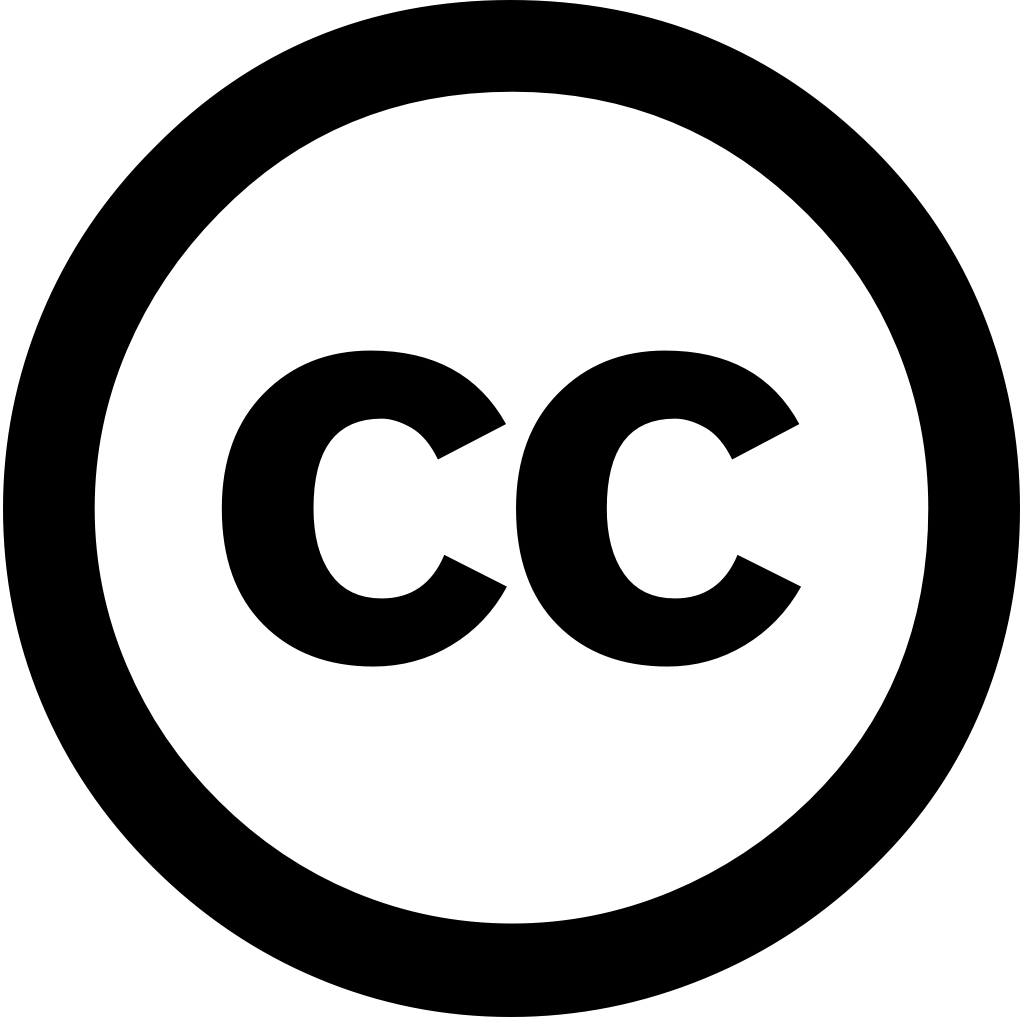
Journal of Geophysical Research Machine Learning and Computation, Journal Year: 2025, Volume and Issue: 2(1)
Published: March 1, 2025
Abstract Marine boundary layer clouds are crucial in Earth's climate system. They frequently manifest as closed or open cell mesoscale cellular convection (MCC). MCC challenging to represent accurately current models, highlighting the need for detailed observational data sets and in‐depth analyses. This study utilizes over 8 years of observations from U.S. Department Energy (DOE) Atmospheric Radiation Measurement (ARM) User Facility Eastern North Atlantic (ENA) site at Graciosa Island, Azores, investigate these clouds. We first apply a convolutional neural network with U‐Net architecture classify cells, marking application such an approach automatically detecting patterns ground‐based radar measurements. method addresses some gaps satellite related low temporal resolution, nighttime challenges, limited vertical structure capture. The analysis cases shows clear differences between MCCs: Closed characterized by lower cloud tops bases, shallower geometrical depth, weaker horizontal wind speeds, stronger atmospheric stability, more homogeneous liquid water path than MCCs. Finally, we demonstrate two potential applications our radar‐based classifications: (a) facilitating investigation aerosol‐cloud interactions (b) exploring meteorological factors along MCC's evolution integrating imagery back‐trajectory analysis. identified offer valuable resource scientific community processes further improve model accuracy.
Language: Английский