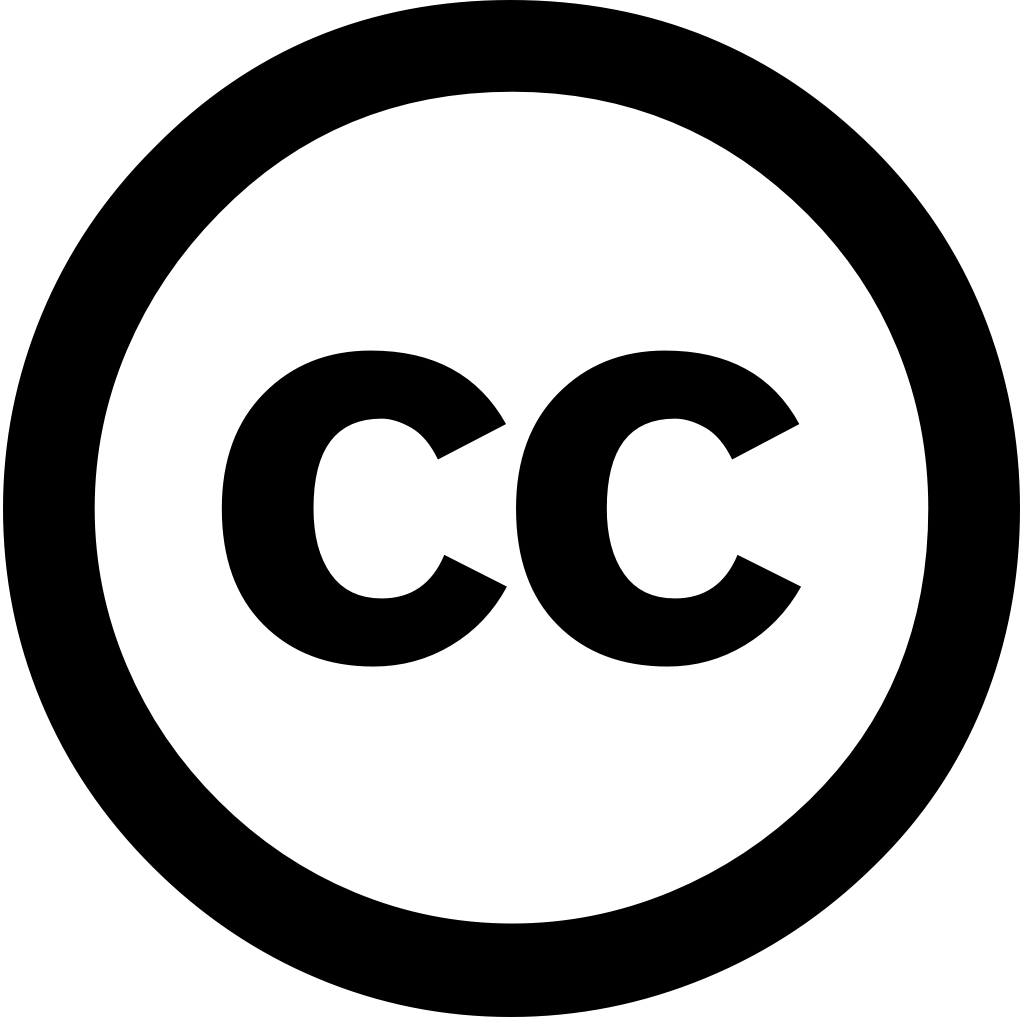
Journal of Geophysical Research Machine Learning and Computation, Journal Year: 2025, Volume and Issue: 2(2)
Published: April 25, 2025
Abstract Land surface temperature (LST) is crucial for understanding earth system processes. We expanded the Advanced Baseline Imager Live Imaging of Vegetated Ecosystems (ALIVE) framework to estimate LST in near‐real‐time both cloudy and clear sky conditions at a five‐minute resolution. compared two machine learning (ML) models, Long Short‐Term Memory (LSTM) networks Gradient Boosting Regressor (GBR), using top‐of‐atmosphere observations from (ABI) on GOES‐16 satellite against hundreds observation sites five‐year period. outperformed GBR, especially coarser resolutions under challenging conditions, with R 2 0.96 (RMSE 2.31K) 0.83 4.10K) across CONUS, based 10‐repeat Leave‐One‐Out Cross‐Validation (LOOCV). GBR maintained high accuracy ran 5.3 times faster, only 0.01–0.02 drop. Feature importance revealed infrared bands were key LSTM adapting dynamically atmospheric changes, while utilized more time information conditions. A comparative analysis physically ABI product showed strong agreement winter, particularly also highlighting challenges summer estimation due increased thermal variability. This study underscores strengths limitations data‐driven models suggests potential pathways integrating ML enhance coverage products.
Language: Английский