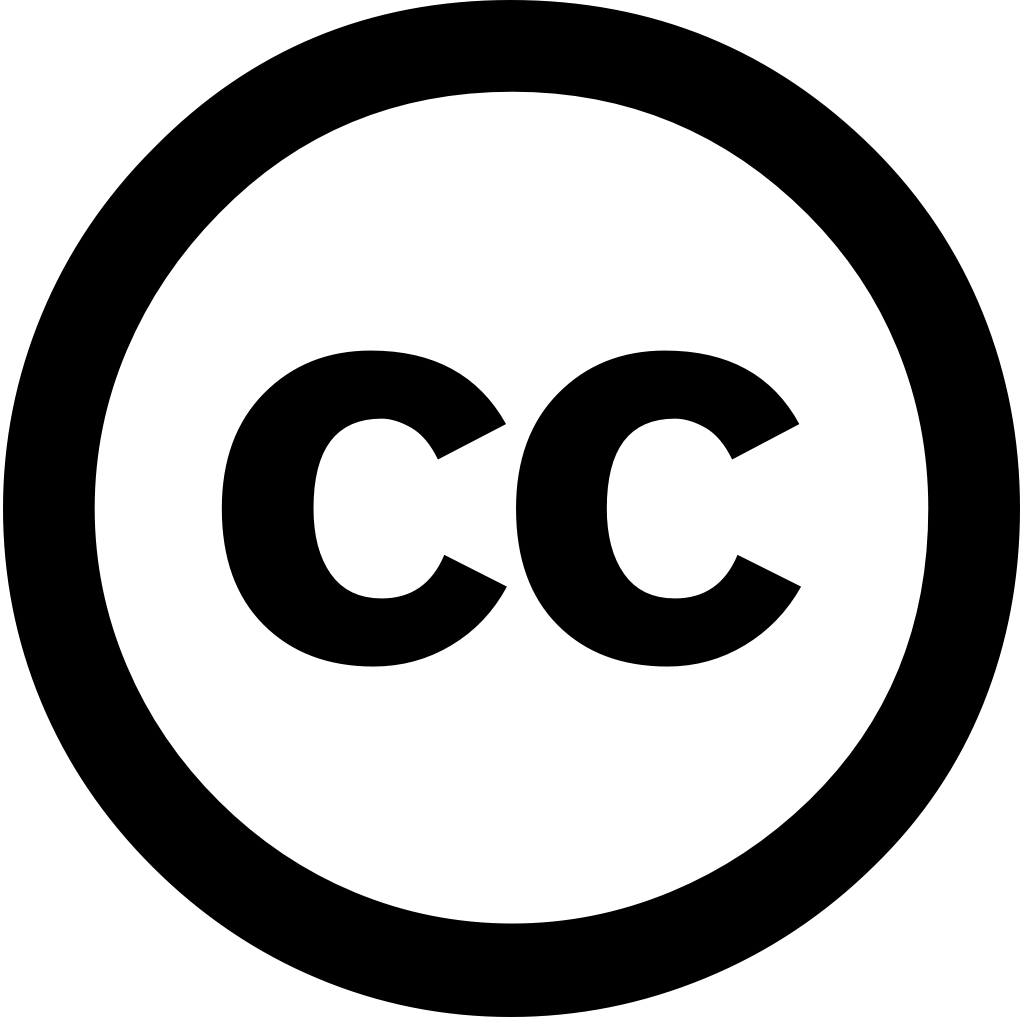
Water Resources Research, Journal Year: 2025, Volume and Issue: 61(3)
Published: March 1, 2025
Abstract Machine learning (ML) is increasingly considered the solution to environmental problems where limited or no physico‐chemical process understanding exists. But in supporting high‐stakes decisions, ability explain possible solutions key their acceptability and legitimacy, ML can fall short. Here, we develop a method, rooted formal sensitivity analysis , uncover primary drivers behind predictions. Unlike many methods for explainable artificial intelligence (XAI), this method (a) accounts complex multi‐variate distributional properties of data, common systems, (b) offers global assessment input‐output response surface formed by ML, rather than focusing solely on local regions around existing data points, (c) scalable data‐size independent, ensuring computational efficiency with large sets. We apply suite models predicting various water quality variables pilot‐scale experimental pit lake. A critical finding that subtle alterations design some (such as variations random seed, functional class, hyperparameters, splitting) lead different interpretations how outputs depend inputs. Further, from families (decision trees, connectionists, kernels) may focus aspects information provided despite displaying similar predictive power. Overall, our results underscore need assess explanatory robustness advocate using model ensembles gain deeper insights into system improve prediction reliability.
Language: Английский