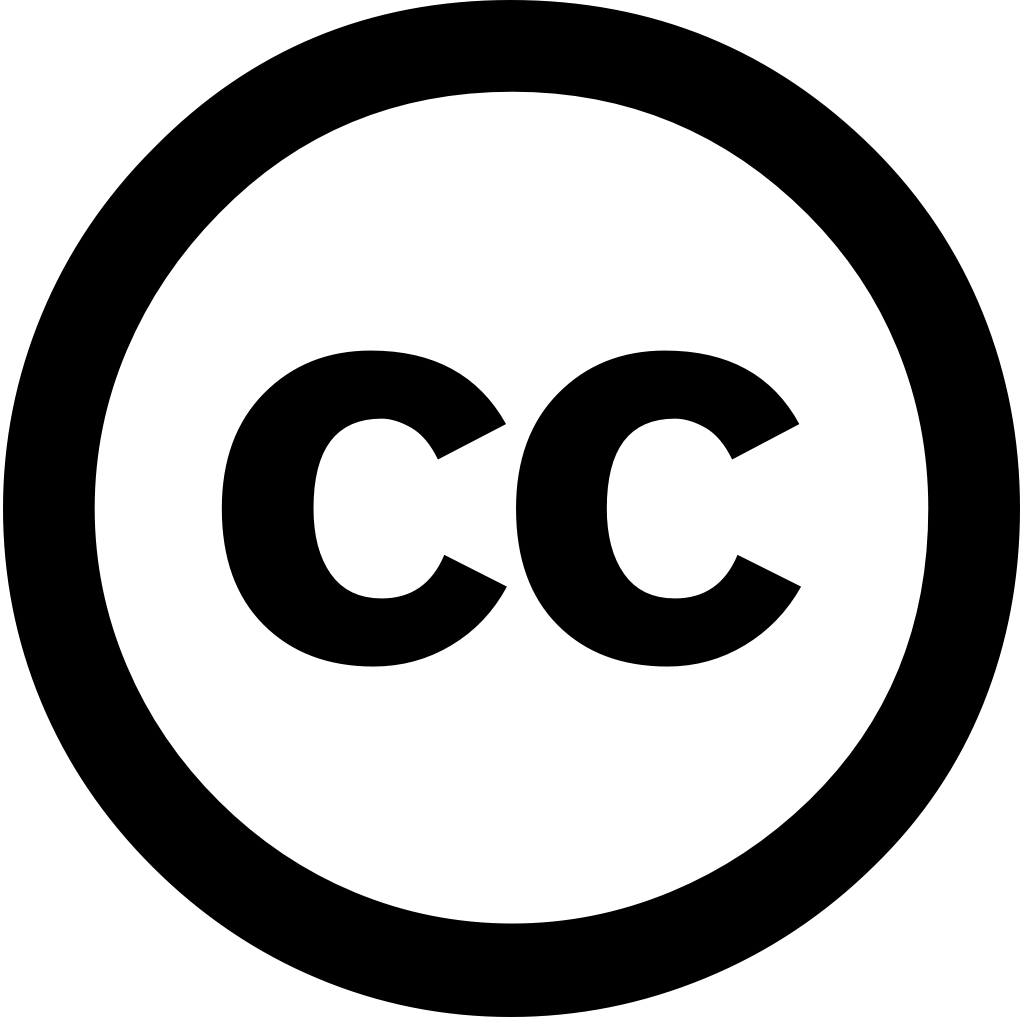
Wellcome Open Research, Journal Year: 2024, Volume and Issue: 9, P. 423 - 423
Published: Aug. 1, 2024
Language: Английский
Wellcome Open Research, Journal Year: 2024, Volume and Issue: 9, P. 423 - 423
Published: Aug. 1, 2024
Language: Английский
Current Opinion in Behavioral Sciences, Journal Year: 2020, Volume and Issue: 38, P. 49 - 56
Published: Nov. 6, 2020
Language: Английский
Citations
164Psychology and Aging, Journal Year: 2024, Volume and Issue: 39(1), P. 88 - 101
Published: Feb. 1, 2024
Deciding whether to explore unknown opportunities or exploit well-known options is a ubiquitous part of our everyday lives. Extensive work in college students suggests that young people make explore-exploit decisions using mixture information seeking and random behavioral variability. Whether, what extent, older adults use the same strategies unknown. To address this question, 51 (ages 65-74) 32 younger 18-25) completed Horizon Task, gambling task quantifies variability as well how these are controlled for purposes exploration. Qualitatively, we found performed similar on task, increasing both their when it was adaptive explore. Quantitively, however, there were substantial differences between age groups, with showing less overall reliance means In addition, subset approximately 26% whose close zero, avoiding informative even they clearly better choice. Unsurprisingly, "information avoiders" worse task. contrast, performance remaining seeking" comparable suggesting age-related decision making may be except taken extremes. (PsycInfo Database Record (c) 2024 APA, all rights reserved).
Language: Английский
Citations
9bioRxiv (Cold Spring Harbor Laboratory), Journal Year: 2025, Volume and Issue: unknown
Published: April 5, 2025
1People differ widely in how they make decisions uncertain environments. While many studies leverage this variability to measure differences specific cognitive processes and parameters, the key dimension(s) of individual decision-making tasks has not been identified. Here, we analyzed behavioral data from 1001 participants performing a restless three-armed bandit task, where reward probabilities fluctuated unpredictably over time. Using novel analytical approach that controlled for stochasticity tasks, identified dominant nonlinear axis variability. We found primary was strongly selectively correlated with probability exploration, as inferred by latent state modeling. This suggests major factor shaping task performance is tendency explore (versus exploit), rather than personality characteristics, reinforcement learning model or low-level strategies. Certain demographic characteristics also predicted variance along principle axis: at exploratory end tended be younger exploitative end, self-identified men were overrepresented both extremes. Together, these findings offer principled framework understanding behavior while highlighting factors shape under uncertainty.
Language: Английский
Citations
0Proceedings of the National Academy of Sciences, Journal Year: 2024, Volume and Issue: 121(45)
Published: Oct. 28, 2024
To understand human learning and progress, it is crucial to curiosity. But how consistent curiosity’s conception assessment across scientific research disciplines? We present the results of a large collaborative project assessing correspondence between curiosity measures in personality psychology cognitive science. A total 820 participants completed 15 trait 9 tasks that tested multiple aspects information demand. show shared variance was captured by dimension reflecting directed (uncertainty-driven) versus random (stochasticity-driven) exploration individual differences along this axis were significantly consistently predicted traits. However, metrics best demand not central traits openness experience, deprivation sensitivity, joyous exploration, but instead included more peripheral (need for cognition, thrill seeking, stress tolerance) traditionally associated with (extraversion behavioral inhibition). The suggest umbrella term “curiosity” reflects constellation emotional processes, only some which are tasks. reflect distinct methods used these fields, indicating need caution comparing fields future interdisciplinary collaborations strengthen our emerging understanding
Language: Английский
Citations
3Neuroscience & Biobehavioral Reviews, Journal Year: 2024, Volume and Issue: 167, P. 105940 - 105940
Published: Nov. 7, 2024
Language: Английский
Citations
3bioRxiv (Cold Spring Harbor Laboratory), Journal Year: 2023, Volume and Issue: unknown
Published: April 27, 2023
Abstract A key feature of animal and human decision-making is to balance the exploration unknown options for information gain (directed exploration) versus selecting known immediate reward (exploitation), which often examined using restless bandit tasks. Recurrent neural network models (RNNs) have recently gained traction in both systems neuroscience work on reinforcement learning, due their ability show meta-learning task domains. Here we comprehensively compared performance a range RNN architectures as well learners four-armed problems. The best-performing architecture (LSTM with computation noise) exhibited human-level performance. Computational modeling behavior first revealed that behavioral data contain signatures higher-order perseveration, i.e., perseveration beyond last trial, but this effect was more pronounced RNNs. In contrast, learners, not RNNs, positive uncertainty choice probability exploration). hidden unit dynamics exploratory choices were associated disruption predictive signals during states low state value, resembling win-stay-loose-shift strategy, resonating previous single recording findings monkey prefrontal cortex. Our results highlight similarities differences between it emerges computational mechanisms identified cognitive work.
Language: Английский
Citations
2npj Aging, Journal Year: 2023, Volume and Issue: 9(1)
Published: Oct. 30, 2023
Abstract Apathy is a pervasive clinical syndrome in neurocognitive disorders, characterized by quantitative reduction goal-directed behaviors. The brain structures involved the physiopathology of apathy have also been connected to probabilistic reward learning exploration-exploitation dilemma. This dilemma question involves challenge selecting between familiar option with more predictable outcome, and another whose outcome uncertain may yield potentially greater rewards compared known option. aim this study was combine experimental procedures computational modeling examine whether, older adults mild affects performance Through using four-armed bandit reinforcement-learning task, we showed that apathetic explored performed worse than non-apathetic subjects. Moreover, mental flexibility assessed Trail-making test-B negatively associated percentage exploration. These results suggest an increased explorative behavior inefficient decision-making, possibly due weak switch toward exploitation rewarding options. Apathetic participants took longer make choice failed often respond allotted time, which could reflect difficulties action initiation selection. In conclusion, present disorders specific disturbances trade-off sheds light on processing patients apathy.
Language: Английский
Citations
1Computational Brain & Behavior, Journal Year: 2024, Volume and Issue: 7(3), P. 314 - 356
Published: May 24, 2024
Abstract A key feature of animal and human decision-making is to balance the exploration unknown options for information gain (directed exploration) versus selecting known immediate reward (exploitation), which often examined using restless bandit tasks. Recurrent neural network models (RNNs) have recently gained traction in both systems neuroscience work on reinforcement learning, due their ability show meta-learning task domains. Here we comprehensively compared performance a range RNN architectures as well learners four-armed problems. The best-performing architecture (LSTM with computation noise) exhibited human-level performance. Computational modeling behavior first revealed that behavioral data contain signatures higher-order perseveration, i.e., perseveration beyond last trial, but this effect was more pronounced RNNs. In contrast, learners, not RNNs, positive uncertainty choice probability exploration). hidden unit dynamics exploratory choices were associated disruption predictive signals during states low state value, resembling win-stay-loose-shift strategy, resonating previous single recording findings monkey prefrontal cortex. Our results highlight similarities differences between it emerges computational mechanisms identified cognitive work.
Language: Английский
Citations
0Wellcome Open Research, Journal Year: 2024, Volume and Issue: 9, P. 423 - 423
Published: Aug. 1, 2024
Language: Английский
Citations
0