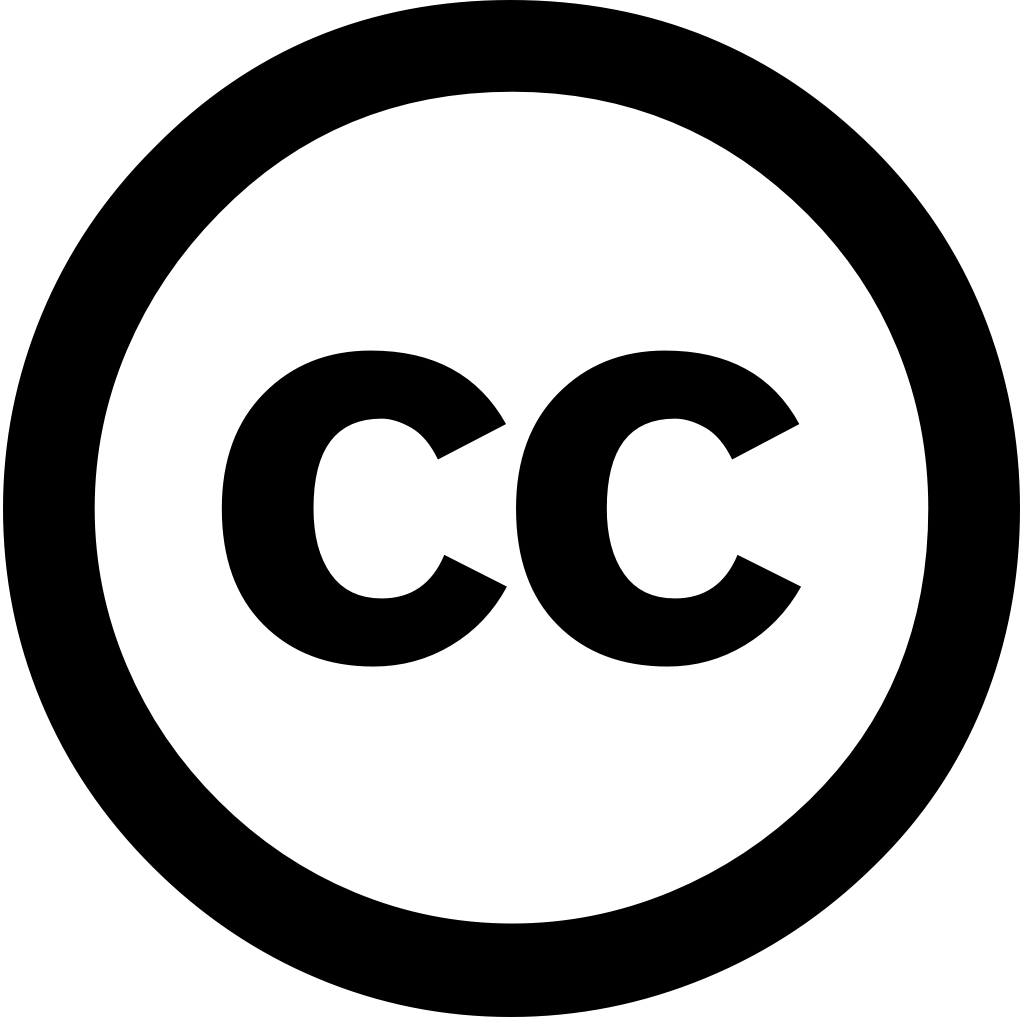
Polymer science & technology., Journal Year: 2025, Volume and Issue: unknown
Published: April 10, 2025
Language: Английский
Polymer science & technology., Journal Year: 2025, Volume and Issue: unknown
Published: April 10, 2025
Language: Английский
Bioprinting, Journal Year: 2024, Volume and Issue: 38, P. e00331 - e00331
Published: Jan. 28, 2024
Language: Английский
Citations
34SmartMat, Journal Year: 2025, Volume and Issue: 6(1)
Published: Jan. 9, 2025
ABSTRACT Machine learning (ML), material genome, and big data approaches are highly overlapped in their strategies, algorithms, models. They can target various definitions, distributions, correlations of concerned physical parameters given polymer systems, have expanding applications as a new paradigm indispensable to conventional ones. Their inherent advantages building quantitative multivariate largely enhanced the capability scientific understanding discoveries, thus facilitating mechanism exploration, prediction, high‐throughput screening, optimization, rational inverse designs. This article summarizes representative progress recent two decades focusing on design, preparation, application, sustainable development materials based exploration key composition–process–structure–property–performance relationship. The integration both data‐driven insights through ML deepen fundamental discover novel is categorically presented. Despite construction application robust models, strategies algorithms deal with variant tasks science still rapid growth. challenges prospects then We believe that innovation will thrive along approaches, from efficient design applications.
Language: Английский
Citations
2Macromolecular Symposia, Journal Year: 2025, Volume and Issue: 414(1)
Published: Feb. 1, 2025
Abstract Technology, health care, and transport are merely some of the industries that historically rely on polymer‐based materials. In past centuries, creation innovative polymer materials has been dependent upon extensive experiments error procedures require an number resources as well time. With objective to explore transformative potential machine learning (ML) artificial intelligence (AI) in material discovery, design, optimization, this paper explores integration ML AI research. Researchers able speed development new with improved properties functionalities by utilizing sophisticated algorithms computational models. The use research is examined, a focus how these technologies may stimulate innovation expand science
Language: Английский
Citations
2Chemistry of Materials, Journal Year: 2024, Volume and Issue: 36(12), P. 5878 - 5896
Published: March 28, 2024
The modulation of fluorescence properties light-emitting molecules, especially in solid or aggregate states, is crucial for their practical applications. Polymeric systems offer flexible structural design and solution-processing abilities, affording versatile platforms achieving color-tunable fluorescence, those with well-defined structures obtained by controlled polymerization. Meanwhile, the emergence aggregation-induced emission (AIE) thermally activated delayed (TADF) concepts has revolutionized field materials, including fluorescent polymers. This Review focuses on recently developed polymer that have realized fine-tuning emissions. It delves into various color-tuning mechanisms, exploring molecular interactions such as dye–dye interactions, charge transfer, energy polymeric microenvironment. Special attention given to through-space transfer (TSCT) unconventional Förster resonance (FRET), which garnered significant interest recently. We aim valuable insights from perspective eventually provide an outlook potential directions future research this field.
Language: Английский
Citations
13Angewandte Chemie, Journal Year: 2024, Volume and Issue: 136(24)
Published: April 8, 2024
Abstract Environmentally friendly crosslinked polymer networks feature degradable covalent or non‐covalent bonds, with many of them manifesting dynamic characteristics. These attributes enable convenient degradation, facile reprocessibility, and self‐healing capabilities. However, the inherent instability these crosslinking bonds often compromises mechanical properties networks, limiting their practical applications. In this context, environmentally dual‐crosslinking (denoted EF‐DCPNs) have emerged as promising alternatives to address challenge. materials effectively balance need for high ability degrade, recycle, and/or self‐heal. Despite potential, investigations into EF‐DCPNs remain in nascent stages, several gaps limitations persist. This Review provides a comprehensive overview synthesis, properties, applications recent progress EF‐DCPNs. Firstly, synthetic routes rich variety possessing two distinct types (i.e., imine, disulfide, ester, hydrogen bond, coordination other bonds) are introduced. Subsequently, complex structure‐ nature‐dependent mechanical, thermal, electrical discussed, followed by exemplary electronics biotechnology. Finally, future research directions rapidly evolving field outlined.
Language: Английский
Citations
11Acta Biomaterialia, Journal Year: 2025, Volume and Issue: unknown
Published: Feb. 1, 2025
Language: Английский
Citations
1Advanced Materials, Journal Year: 2025, Volume and Issue: unknown
Published: Feb. 9, 2025
Machine learning is increasingly being applied in polymer chemistry to link chemical structures macroscopic properties of polymers and identify patterns the that help improve specific properties. To facilitate this, a dataset needs be translated into machine readable descriptors. However, limited inadequately curated datasets, broad molecular weight distributions, irregular configurations pose significant challenges. Most off shelf mathematical models often need refinement for applications. Addressing these challenges demand close collaboration between chemists mathematicians as must formulate research questions terms while are required refine This review unites both disciplines address curation hurdles highlight advances synthesis modeling enhance data availability. It then surveys ML approaches used predict solid-state properties, solution behavior, composite performance, emerging applications such drug delivery polymer-biology interface. A perspective field concluded importance FAIR (findability, accessibility, interoperability, reusability) integration theory discussed, thoughts on machine-human interface shared.
Language: Английский
Citations
1Advanced Engineering Materials, Journal Year: 2025, Volume and Issue: unknown
Published: Feb. 13, 2025
Electrospinning is a versatile technique for producing micro‐ and nanoscale fibers, offering vast potential to address critical market demands, particularly in biomedical engineering. However, the industrial adoption of electrospinning as manufacturing technology faces significant hurdles, notably achieving precise control over fiber properties ensuring reproducibility scalability. These challenges directly impact its viability creating advanced products. Bridging gap between material properties, end‐user requirements, process parameters essential unlocking full electrospinning. This work provides comprehensive review modalities, operational factors, modeling techniques, emphasizing their role optimizing process. The use strategies machine learning methods explored, showcasing enhance performance. highlights connection product performance electrospinning, well necessary conditions applications. In addition, identifies gaps unexplored areas, roadmap future innovation fabrication. By synergy intelligent design applications, this lays groundwork advancements, positioning cornerstone next‐generation technologies.
Language: Английский
Citations
1Macromolecular Symposia, Journal Year: 2025, Volume and Issue: 414(1)
Published: Feb. 1, 2025
Abstract Artificial intelligence (AI) and machine learning (ML) have advanced tremendously in the previous 5 years regarding polymer science. Polymers are materials with enormous versatility that now widely used. found extensive applications several fields such as energy storage, construction, medical, aerospace, other industries. This study is presently era of 4.0 industry, a transformative period profoundly reshaping both business society an unprecedented manner specifically developing countries. Data‐driven strategies for process analysis control crucial expediting creation production processes while maintaining product quality avoiding rise cost. More more scientists utilizing informatics data science to create new understand connections between their molecular structure characteristics. The field relatively new. Even though there lot helpful databases tools accessible, not many used frequently. application AI starting influence on aspects human existence, including technology. Polymer utilizes ML techniques enhance developing, designing, discovering polymers. Based these ideas, it examines burgeoning ML‐assisted this research. It also looks at developments polymeric ecosystem talks about upcoming potential problems applications.
Language: Английский
Citations
1Angewandte Chemie International Edition, Journal Year: 2024, Volume and Issue: 63(24)
Published: April 8, 2024
Environmentally friendly crosslinked polymer networks feature degradable covalent or non-covalent bonds, with many of them manifesting dynamic characteristics. These attributes enable convenient degradation, facile reprocessibility, and self-healing capabilities. However, the inherent instability these crosslinking bonds often compromises mechanical properties networks, limiting their practical applications. In this context, environmentally dual-crosslinking (denoted EF-DCPNs) have emerged as promising alternatives to address challenge. materials effectively balance need for high ability degrade, recycle, and/or self-heal. Despite potential, investigations into EF-DCPNs remain in nascent stages, several gaps limitations persist. This Review provides a comprehensive overview synthesis, properties, applications recent progress EF-DCPNs. Firstly, synthetic routes rich variety possessing two distinct types (i.e., imine, disulfide, ester, hydrogen bond, coordination other bonds) are introduced. Subsequently, complex structure- nature-dependent mechanical, thermal, electrical discussed, followed by exemplary electronics biotechnology. Finally, future research directions rapidly evolving field outlined.
Language: Английский
Citations
7