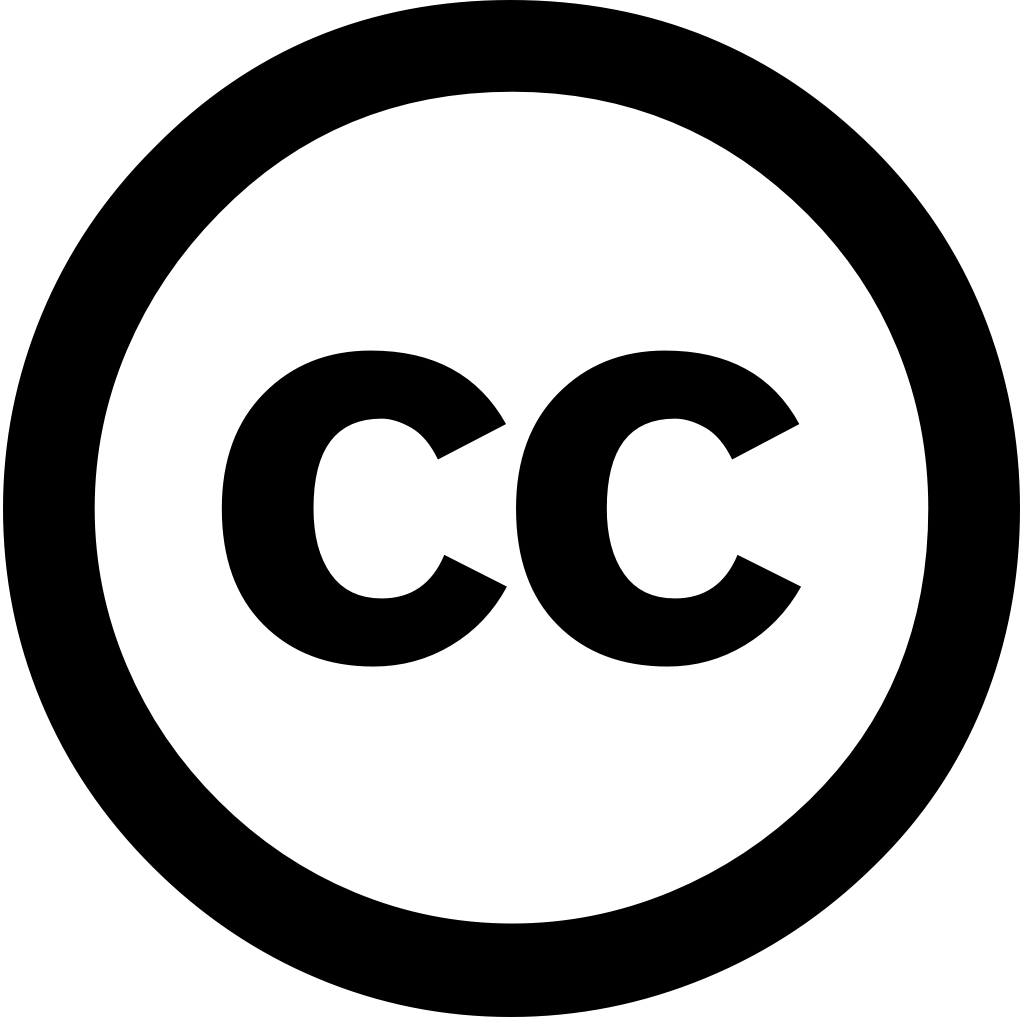
medRxiv (Cold Spring Harbor Laboratory), Journal Year: 2024, Volume and Issue: unknown
Published: Oct. 18, 2024
Abstract Understanding the past, current, and future dynamics of dengue epidemics is challenging yet increasingly important. To date, many techniques across statistics, mathematics, machine learning have provided us with quantitative tools for studying epidemics. Here, using data from provinces in northern Peru 2010 to 2021, we provide a new interdisciplinary pipeline that draws on existing comprehensive understanding robust prediction epidemic dynamics. Wavelet analyses can unveil spatiotemporal patterns annual multi-annual time periods. these included climatic forcing greater spatial similarity large outbreak years. Space-varying drivers influences shorter pairwise distances driving more northerly coastal provinces. Then, Bayesian model, probabilistically quantify timing, structure, intensity such Dengue Incidence Rates (DIRs), while simultaneously considering other influences. Recognising single model generally sub-optimal any forecasting task, demonstrate how form trained untrained probabilistic ensembles cases settings reflective real-world conditions. We introduce suite climate-informed covariate-free deep approaches leverage big foundational series, temporal convolutional networks, conformal inference. complement modern statistically principled training assessment ensemble frameworks, explicitly strong benchmark models, computational costs, public health priorities, availability limitations. In doing so, show frameworks consistently outperform individual models space time, produce sharp accurate forecasts robust, reliable descriptions uncertainty. report interpretable classification metrics detection outbreaks communicate our outputs wider authorities. Looking forward, whether objective understand and/or predict dynamics, modelling be used setting robustly inform decision-making planning
Language: Английский