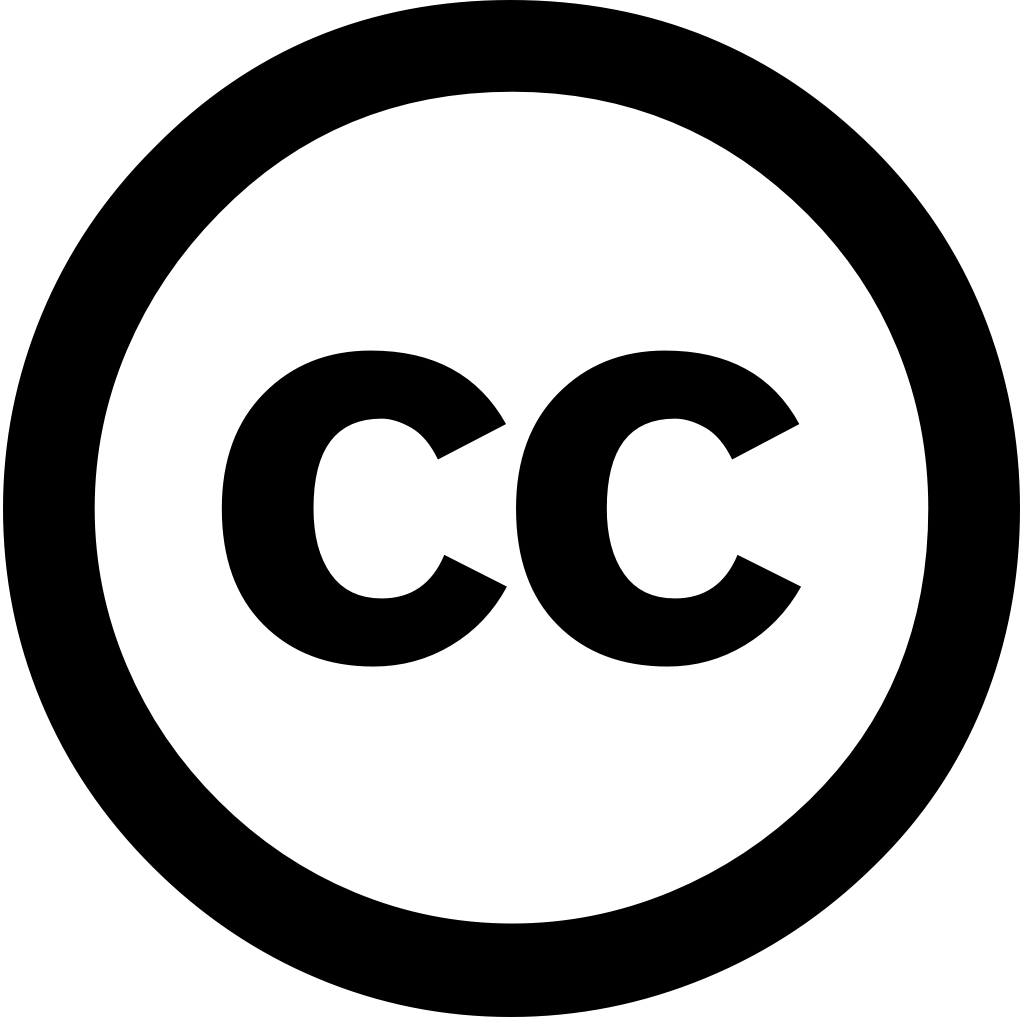
Calphad, Journal Year: 2023, Volume and Issue: 82, P. 102580 - 102580
Published: June 26, 2023
Language: Английский
Calphad, Journal Year: 2023, Volume and Issue: 82, P. 102580 - 102580
Published: June 26, 2023
Language: Английский
Nature Communications, Journal Year: 2024, Volume and Issue: 15(1)
Published: Feb. 15, 2024
Extracting structured knowledge from scientific text remains a challenging task for machine learning models. Here, we present simple approach to joint named entity recognition and relation extraction demonstrate how pretrained large language models (GPT-3, Llama-2) can be fine-tuned extract useful records of complex knowledge. We test three representative tasks in materials chemistry: linking dopants host materials, cataloging metal-organic frameworks, general composition/phase/morphology/application information extraction. Records are extracted single sentences or entire paragraphs, the output returned as English more format such list JSON objects. This represents simple, accessible, highly flexible route obtaining databases specialized research papers.
Language: Английский
Citations
138Digital Discovery, Journal Year: 2023, Volume and Issue: 2(5), P. 1233 - 1250
Published: Jan. 1, 2023
We report the findings of a hackathon focused on exploring diverse applications large language models in molecular and materials science.
Language: Английский
Citations
115Journal of Cleaner Production, Journal Year: 2022, Volume and Issue: 385, P. 135671 - 135671
Published: Dec. 19, 2022
In order to keep the "good" status of coastal water quality, it is essential monitor and assess frequently. The Water quality index (WQI) model one most widely used techniques for assessment quality. It consists five components, with indicator selection technique being more crucial components. Several studies conducted recently have shown that use existing results in a significant amount uncertainty produced final due inappropriate selection. present study carried out comprehensive various features (FS) selecting indicators develop an efficient WQI model. This aims analyse effects eighteen different FS techniques, including (i) nine filter methods, (ii) two wrapper (iii) seven embedded methods comparison performance WQI. total, fifteen combinations (subsets) were constructed, values calculated each combination using improvement methodology model's was tested machine-learning algorithms, which validated metrics. indicated tree-based random forest algorithm could be effective terms assessing water. Deep neural network showed better predicting accurately incorporating subset forest.
Language: Английский
Citations
94Advanced Functional Materials, Journal Year: 2023, Volume and Issue: 33(17)
Published: Feb. 15, 2023
Abstract Data‐driven epoch, the development of machine learning (ML) in materials and device design is an irreversible trend. Its ability efficiency to handle nonlinear game‐playing problems unmatched by traditional simulation computing software trial‐error experiments. Perovskite solar cells are complex physicochemical devices (systems) that consist perovskite materials, transport layer electrodes. Predicting properties screening component related strong point ML. However, applications ML has only begun boom last two years, so it necessary provide a review involved technologies, application status, facing urgent challenges blueprint.
Language: Английский
Citations
81Nano-Micro Letters, Journal Year: 2023, Volume and Issue: 15(1)
Published: Oct. 13, 2023
Abstract Efficient electrocatalysts are crucial for hydrogen generation from electrolyzing water. Nevertheless, the conventional "trial and error" method producing advanced is not only cost-ineffective but also time-consuming labor-intensive. Fortunately, advancement of machine learning brings new opportunities discovery design. By analyzing experimental theoretical data, can effectively predict their evolution reaction (HER) performance. This review summarizes recent developments in low-dimensional electrocatalysts, including zero-dimension nanoparticles nanoclusters, one-dimensional nanotubes nanowires, two-dimensional nanosheets, as well other electrocatalysts. In particular, effects descriptors algorithms on screening investigating HER performance highlighted. Finally, future directions perspectives electrocatalysis discussed, emphasizing potential to accelerate electrocatalyst discovery, optimize performance, provide insights into electrocatalytic mechanisms. Overall, this work offers an in-depth understanding current state its research.
Language: Английский
Citations
74Journal of Composites Science, Journal Year: 2023, Volume and Issue: 7(9), P. 364 - 364
Published: Sept. 1, 2023
The determination of mechanical properties plays a crucial role in utilizing composite materials across multiple engineering disciplines. Recently, there has been substantial interest employing artificial intelligence, particularly machine learning and deep learning, to accurately predict the materials. This comprehensive review paper examines applications intelligence forecasting different types composites. begins with an overview then outlines process predicting material properties. primary focus this lies exploring various techniques employed Furthermore, highlights theoretical foundations, strengths, weaknesses each method used for Finally, based on findings, discusses key challenges suggests future research directions field prediction, offering valuable insights further exploration. is intended serve as significant reference researchers engaging studies within domain.
Language: Английский
Citations
71Applied Surface Science Advances, Journal Year: 2023, Volume and Issue: 18, P. 100523 - 100523
Published: Nov. 28, 2023
This comprehensive review investigates the multifaceted applications of machine learning in materials research across six key dimensions, redefining field's boundaries. It explains various knowledge acquisition mechanisms starting with supervised, unsupervised, reinforcement, and deep techniques. These techniques are transformative tools for transforming unactionable data into insightful actions. Moving on to synthesis, emphasizes profound influence learning, as demonstrated by predictive models that speed up material selection, structure-property relationships reveal crucial connections, data-driven discovery fosters innovation. Machine reshapes our comprehension manipulation accelerating enabling tailored design through property prediction relationships. extends its image processing, improving object detection, classification, segmentation precision methods like generation, revolutionizing potential processing research. The most recent developments show how can have a impact at atomic level precise intricate extraction, representing significant advancements understanding highlights has revolutionize discovery, performance, stimulating does so while acknowledging obstacles poor quality complicated algorithms. offers wide range exciting prospects scientific investigation technological advancement, positioning it powerful force influencing future
Language: Английский
Citations
61npj Computational Materials, Journal Year: 2023, Volume and Issue: 9(1)
Published: April 7, 2023
Abstract Recent advances in machine learning (ML) have led to substantial performance improvement material database benchmarks, but an excellent benchmark score may not imply good generalization performance. Here we show that ML models trained on Materials Project 2018 can severely degraded new compounds 2021 due the distribution shift. We discuss how foresee issue with a few simple tools. Firstly, uniform manifold approximation and projection (UMAP) be used investigate relation between training test data within feature space. Secondly, disagreement multiple illuminate out-of-distribution samples. demonstrate UMAP-guided query by committee acquisition strategies greatly improve prediction accuracy adding only 1% of data. believe this work provides valuable insights for building databases enable better robustness generalizability.
Language: Английский
Citations
56Matter, Journal Year: 2023, Volume and Issue: 6(6), P. 1831 - 1859
Published: June 1, 2023
Language: Английский
Citations
49Journal of Chemical Theory and Computation, Journal Year: 2023, Volume and Issue: 19(8), P. 2149 - 2160
Published: March 27, 2023
Chemists can be skeptical in using deep learning (DL) decision making, due to the lack of interpretability "black-box" models. Explainable artificial intelligence (XAI) is a branch (AI) which addresses this drawback by providing tools interpret DL models and their predictions. We review principles XAI domain chemistry emerging methods for creating evaluating explanations. Then, we focus on developed our group applications predicting solubility, blood-brain barrier permeability, scent molecules. show that like chemical counterfactuals descriptor explanations explain predictions while giving insight into structure-property relationships. Finally, discuss how two-step process developing black-box model explaining uncover
Language: Английский
Citations
48