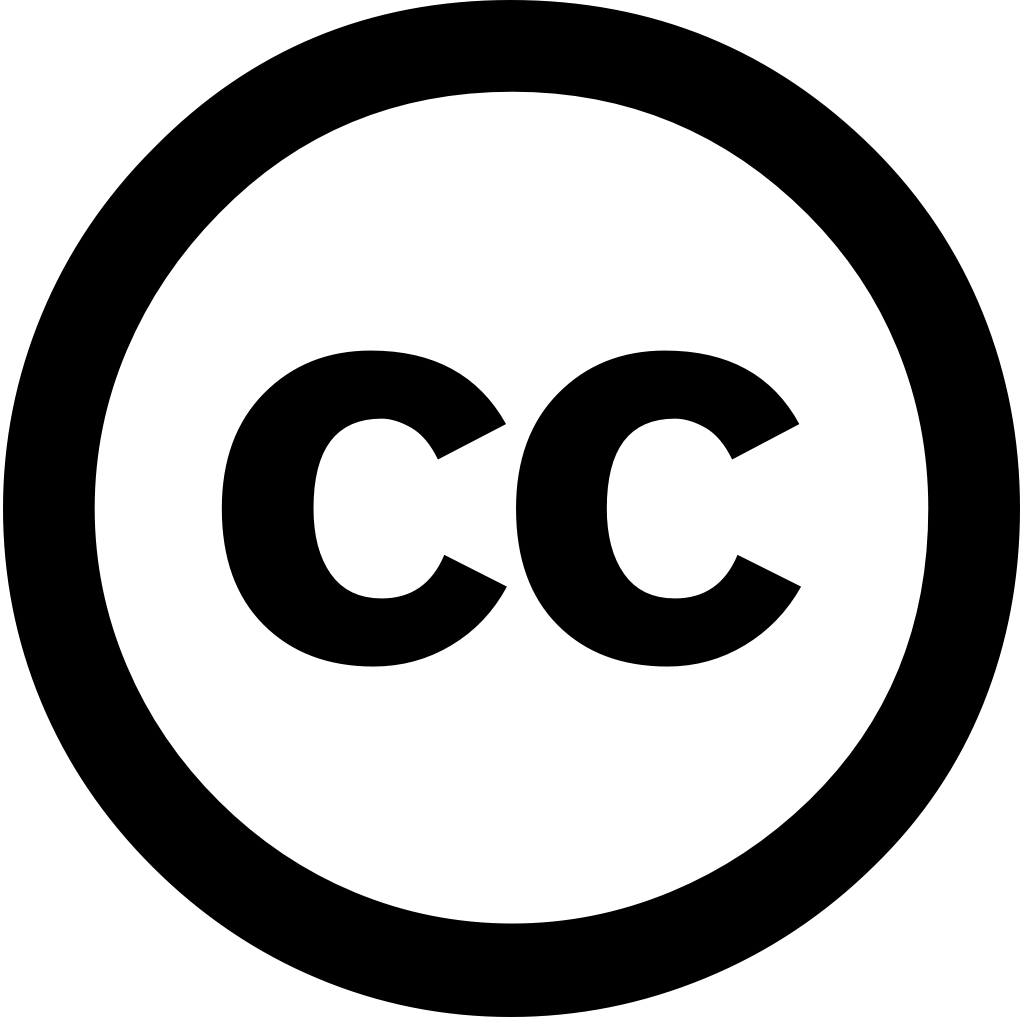
bioRxiv (Cold Spring Harbor Laboratory), Journal Year: 2025, Volume and Issue: unknown
Published: April 22, 2025
Abstract Spatial multi-omics data offer a powerful framework for integrating diverse molecular profiles while maintaining the spatial organization of cells. However, inherent variations in quality and noise levels across different modalities pose significant challenges to accurate integration analyses. In this paper, we introduce CANDIES, which leverages conditional diffusion model contrastive learning effectively denoise integrates data. With our innovative algorithm designs, CANDIES not only enhances data, but also yields unified comprehensive joint representation, thereby empowering many downstream analysis. We conduct extensive evaluations on synthetic real datasets, including CITE-seq from human skin biopsy tissue, MISAR-seq mouse brain, ATAC-RNA-seq embryo 10× visium lymph nodes. shows superior performance various tasks, denoising, domain identification, spatiotemporal trajectories reconstruction, association mapping complex traits. particular, show that representations can be integrated with rich resources genome-wide studies (GWASs), allowing domains linked traits, yielding spatially resolved interpretation traits their relevant tissues.
Language: Английский