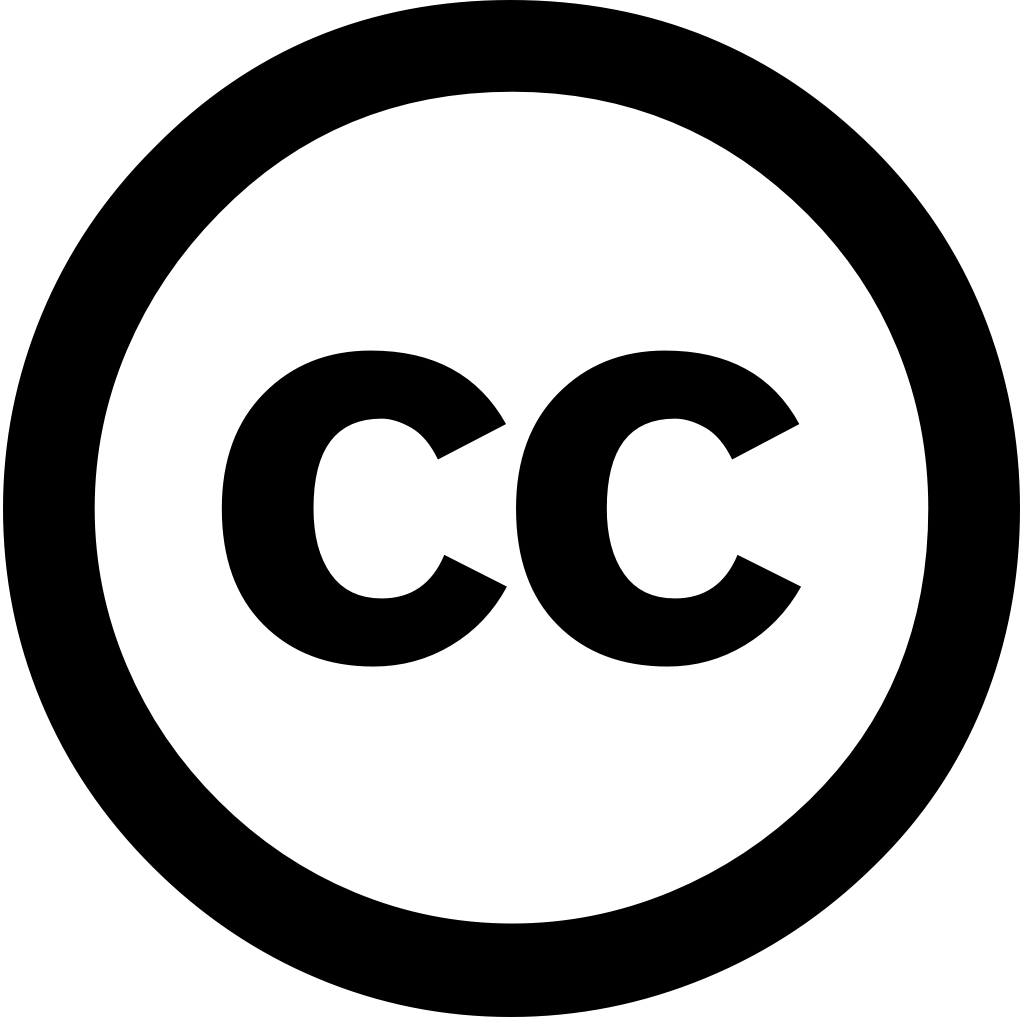
Journal of Pharmaceutical Analysis, Journal Year: 2025, Volume and Issue: unknown, P. 101265 - 101265
Published: March 1, 2025
Language: Английский
Journal of Pharmaceutical Analysis, Journal Year: 2025, Volume and Issue: unknown, P. 101265 - 101265
Published: March 1, 2025
Language: Английский
Computational and Structural Biotechnology Journal, Journal Year: 2025, Volume and Issue: 27, P. 265 - 277
Published: Jan. 1, 2025
Despite the wealth of single-cell multi-omics data, it remains challenging to predict consequences novel genetic and chemical perturbations in human body. It requires knowledge molecular interactions at all biological levels, encompassing disease models humans. Current machine learning methods primarily establish statistical correlations between genotypes phenotypes but struggle identify physiologically significant causal factors, limiting their predictive power. Key challenges modeling include scarcity labeled generalization across different domains, disentangling causation from correlation. In light recent advances data integration, we propose a new artificial intelligence (AI)-powered biology-inspired multi-scale framework tackle these issues. This will integrate organism hierarchies, species genotype-environment-phenotype relationships under various conditions. AI inspired by biology may targets, biomarkers, pharmaceutical agents, personalized medicines for presently unmet medical needs.
Language: Английский
Citations
7Genome Medicine, Journal Year: 2025, Volume and Issue: 17(1)
Published: Feb. 7, 2025
Abstract Ineffective medication is a major healthcare problem causing significant patient suffering and economic costs. This issue stems from the complex nature of diseases, which involve altered interactions among thousands genes across multiple cell types organs. Disease progression can vary between patients over time, influenced by genetic environmental factors. To address this challenge, digital twins have emerged as promising approach, led to international initiatives aiming at clinical implementations. Digital are virtual representations health disease processes that integrate real-time data simulations predict, prevent, personalize treatments. Early applications DTs shown potential in areas like artificial organs, cancer, cardiology, hospital workflow optimization. However, widespread implementation faces several challenges: (1) characterizing dynamic molecular changes biological scales; (2) developing computational methods into DTs; (3) prioritizing mechanisms therapeutic targets; (4) creating interoperable DT systems learn each other; (5) designing user-friendly interfaces for clinicians; (6) scaling technology globally equitable access; (7) addressing ethical, regulatory, financial considerations. Overcoming these hurdles could pave way more predictive, preventive, personalized medicine, potentially transforming delivery improving outcomes.
Language: Английский
Citations
4Nature Reviews Drug Discovery, Journal Year: 2025, Volume and Issue: unknown
Published: March 18, 2025
Language: Английский
Citations
2Environmental Science & Technology, Journal Year: 2025, Volume and Issue: unknown
Published: Jan. 2, 2025
Language: Английский
Citations
2Aging, Journal Year: 2025, Volume and Issue: unknown
Published: Jan. 16, 2025
With the global population aging at an unprecedented rate, there is a need to extend healthy productive life span. This review examines how Deep Learning (DL) and Generative Artificial Intelligence (GenAI) are used in biomarker discovery, deep clock development, geroprotector identification generation of dual-purpose therapeutics targeting disease. The paper explores emergence multimodal, multitasking research systems highlighting promising future directions for GenAI human animal research, as well clinical application longevity medicine.
Language: Английский
Citations
1Pharmacological Research, Journal Year: 2025, Volume and Issue: unknown, P. 107633 - 107633
Published: Jan. 1, 2025
There is an urgent need for mechanistically novel and more efficacious treatments schizophrenia, especially those targeting negative cognitive symptoms with a favorable side-effect profile. Drug repurposing-the process of identifying new therapeutic uses already approved compounds-offers promising approach to overcoming the lengthy, costly, high-risk traditional CNS drug discovery. This review aims update our previous findings on clinical repurposing pipeline in schizophrenia. We examined studies conducted between 2018 2024, 61 trials evaluating 40 unique repurposed candidates. These encompassed broad range pharmacological mechanisms, including immunomodulation, enhancement, hormonal, metabolic, neurotransmitter modulation. A notable development combination muscarinic modulators xanomeline, compound antipsychotic properties, trospium, included mitigate peripheral side effects, now by FDA as first decades fundamentally mechanism action. Moving beyond dopaminergic paradigm such highlight opportunities improve treatment-resistant alleviate adverse effects. Overall, evolving landscape illustrates significant shift rationale schizophrenia development, highlighting potential silico strategies, biomarker-based patient stratification, personalized that align underlying pathophysiological processes.
Language: Английский
Citations
1Journal of Chemical Information and Modeling, Journal Year: 2025, Volume and Issue: unknown
Published: Jan. 31, 2025
Accurately identifying new therapeutic uses for drugs is essential to advancing pharmaceutical research and development. Graph inference techniques have shown great promise in predicting drug–disease associations, offering both high convergence accuracy efficiency. However, most existing methods fail sufficiently address the issue of numerous missing information association networks. Moreover, are often constrained by local or single-directional reasoning. To overcome these limitations, we propose a novel approach, truncated arctangent rank minimization double-strategy neighborhood constraint graph (TARMDNGI), prediction. First, calculate Gaussian kernel Laplace similarities diseases, which then integrated using nonlinear fusion techniques. We introduce matrix completion technique, referred as TARM. TARM takes adjacency heterogeneous networks target enhances robustness formability edges DDA minimization. Additionally, method predict associations. This technique focuses on neighboring nodes filtering out potential noise from more distant nodes. Furthermore, DNGI employs top-down bottom-up strategies infer associations entire network. The synergy dual can enhance comprehensive processing complex structures cross-domain graphs, ensuring that rich network fully utilized. Experimental results consistently demonstrate TARMDNGI outperforms state-of-the-art models across two datasets, one lncRNA-disease dataset, microbe-disease dataset.
Language: Английский
Citations
1Journal of Chemical Information and Modeling, Journal Year: 2024, Volume and Issue: unknown
Published: Dec. 17, 2024
Rare diseases (RDs), affecting 300 million people globally, present a daunting public health challenge characterized by complexity, limited treatment options, and diagnostic hurdles. Despite legislative efforts, such as the 1983 US Orphan Drug Act, more than 90% of RDs lack effective therapies. Traditional drug discovery models, marked lengthy development cycles high failure rates, struggle to meet unique demands RDs, often yielding poor returns on investment. However, advent artificial intelligence (AI), encompassing machine learning (ML) deep (DL), offers groundbreaking solutions. This review explores AI's potential revolutionize for overcoming these challenges. It discusses AI-driven advancements, repurposing, biomarker discovery, personalized medicine, genetics, clinical trial optimization, corporate innovations, novel target identification. By synthesizing current knowledge recent breakthroughs, this provides crucial insights into how AI can accelerate therapeutic ultimately improving patient outcomes. comprehensive analysis fills critical gap in literature, enhancing understanding pivotal role transforming RD research guiding future efforts vital area medicine.
Language: Английский
Citations
4Bioinformatics, Journal Year: 2025, Volume and Issue: unknown
Published: Jan. 20, 2025
Abstract Motivation The drug-disease, gene-disease, and drug-gene relationships, as high-frequency edge types, describe complex biological processes within the biomedical knowledge graph. structural patterns formed by these three edges are graph motifs of (disease, drug, gene) triplets. Among them, triangle is a steady important motif structure in network, other various different from also indicate rich semantic relationships. However, existing methods only focus on representation learning for classification, fail to further discriminate A comprehensive method needed predict triplets, which will uncover new pharmacological mechanisms improve our understanding disease-gene-drug interactions. Identifying structures triplets can help us study properties triangles. Results We consider seven typical propose novel contrastive learning-based triplet prediction (TriMoGCL). TriMoGCL utilizes convolutional encoder extract node features global network topology. Next, pooling context information local views. To avoid redundant imbalance problem caused dense edges, we employ class-prototype denoise enhance discrimination between motifs. experiments two different-scale graphs demonstrate effectiveness reliability identifying types. Additionally, model reveals mechanisms, providing analysis Availability Implementation Codes datasets available at https://github.com/zhanglabNKU/TriMoGCL https://doi.org/10.5281/zenodo.14633572
Language: Английский
Citations
0Published: Jan. 22, 2025
Language: Английский
Citations
0