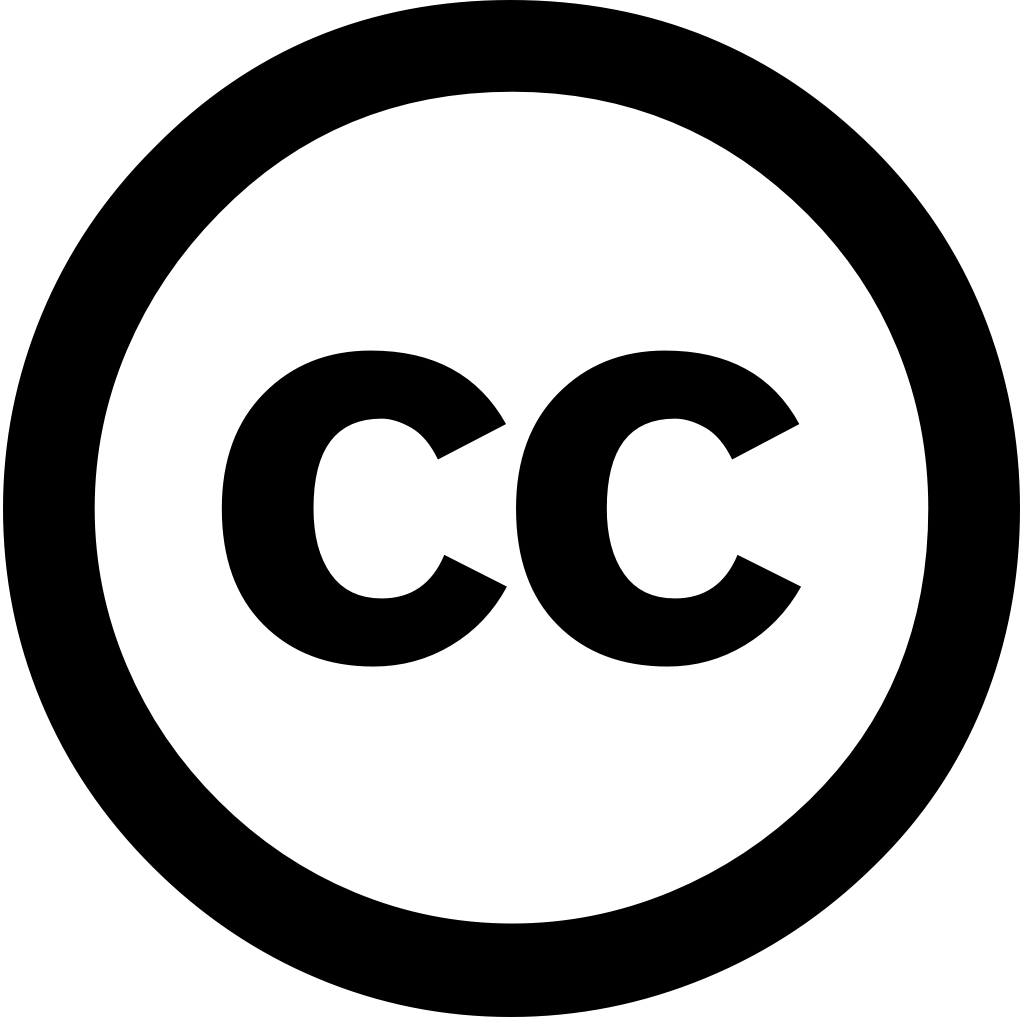
Cell, Journal Year: 2020, Volume and Issue: 182(6), P. 1606 - 1622.e23
Published: Sept. 1, 2020
Language: Английский
Cell, Journal Year: 2020, Volume and Issue: 182(6), P. 1606 - 1622.e23
Published: Sept. 1, 2020
Language: Английский
Cell, Journal Year: 2019, Volume and Issue: 177(7), P. 1888 - 1902.e21
Published: June 1, 2019
Language: Английский
Citations
12849Genome biology, Journal Year: 2019, Volume and Issue: 20(1)
Published: Dec. 1, 2019
Single-cell RNA-seq (scRNA-seq) data exhibits significant cell-to-cell variation due to technical factors, including the number of molecules detected in each cell, which can confound biological heterogeneity with effects. To address this, we present a modeling framework for normalization and variance stabilization molecular count from scRNA-seq experiments. We propose that Pearson residuals "regularized negative binomial regression," where cellular sequencing depth is utilized as covariate generalized linear model, successfully remove influence characteristics downstream analyses while preserving heterogeneity. Importantly, show an unconstrained model may overfit data, overcome this by pooling information across genes similar abundances obtain stable parameter estimates. Our procedure omits need heuristic steps pseudocount addition or log-transformation improves common analytical tasks such variable gene selection, dimensional reduction, differential expression. approach be applied any UMI-based dataset freely available part R package sctransform, direct interface our single-cell toolkit Seurat.
Language: Английский
Citations
3479Molecular Systems Biology, Journal Year: 2019, Volume and Issue: 15(6)
Published: June 1, 2019
Single-cell RNA-seq has enabled gene expression to be studied at an unprecedented resolution. The promise of this technology is attracting a growing user base for single-cell analysis methods. As more tools are becoming available, it increasingly difficult navigate landscape and produce up-to-date workflow analyse one's data. Here, we detail the steps typical analysis, including pre-processing (quality control, normalization, data correction, feature selection, dimensionality reduction) cell- gene-level downstream analysis. We formulate current best-practice recommendations these based on independent comparison studies. have integrated into workflow, which apply public dataset further illustrate how work in practice. Our documented case study can found https://www.github.com/theislab/single-cell-tutorial This review will serve as tutorial new entrants field, help established users update their pipelines.
Language: Английский
Citations
1724Genome biology, Journal Year: 2019, Volume and Issue: 20(1)
Published: March 19, 2019
Single-cell RNA-seq quantifies biological heterogeneity across both discrete cell types and continuous transitions. Partition-based graph abstraction (PAGA) provides an interpretable graph-like map of the arising data manifold, based on estimating connectivity manifold partitions ( https://github.com/theislab/paga ). PAGA maps preserve global topology data, allow analyzing at different resolutions, result in much higher computational efficiency typical exploratory analysis workflow. We demonstrate method by inferring structure-rich with consistent four hematopoietic datasets, adult planaria zebrafish embryo benchmark performance one million neurons.
Language: Английский
Citations
1325Nature Biotechnology, Journal Year: 2023, Volume and Issue: 42(2), P. 293 - 304
Published: May 25, 2023
Language: Английский
Citations
1322Genome biology, Journal Year: 2020, Volume and Issue: 21(1)
Published: Feb. 7, 2020
Abstract The recent boom in microfluidics and combinatorial indexing strategies, combined with low sequencing costs, has empowered single-cell technology. Thousands—or even millions—of cells analyzed a single experiment amount to data revolution biology pose unique science problems. Here, we outline eleven challenges that will be central bringing this emerging field of forward. For each challenge, highlight motivating research questions, review prior work, formulate open This compendium is for established researchers, newcomers, students alike, highlighting interesting rewarding problems the coming years.
Language: Английский
Citations
1037Science Advances, Journal Year: 2020, Volume and Issue: 6(28)
Published: July 8, 2020
Human lung single-cell atlas reveals the complexity and diversity of aberrant cellular populations in pulmonary fibrosis.
Language: Английский
Citations
998Nature Reviews Genetics, Journal Year: 2019, Volume and Issue: 20(7), P. 389 - 403
Published: April 10, 2019
Language: Английский
Citations
954European Journal of Immunology, Journal Year: 2019, Volume and Issue: 49(10), P. 1457 - 1973
Published: Oct. 1, 2019
These guidelines are a consensus work of considerable number members the immunology and flow cytometry community. They provide theory key practical aspects enabling immunologists to avoid common errors that often undermine immunological data. Notably, there comprehensive sections all major immune cell types with helpful Tables detailing phenotypes in murine human cells. The latest techniques applications also described, featuring examples data can be generated and, importantly, how analysed. Furthermore, tips, tricks pitfalls avoid, written peer-reviewed by leading experts field, making this an essential research companion.
Language: Английский
Citations
876Nature Methods, Journal Year: 2021, Volume and Issue: 19(1), P. 41 - 50
Published: Dec. 23, 2021
Abstract Single-cell atlases often include samples that span locations, laboratories and conditions, leading to complex, nested batch effects in data. Thus, joint analysis of atlas datasets requires reliable data integration. To guide integration method choice, we benchmarked 68 preprocessing combinations on 85 batches gene expression, chromatin accessibility simulation from 23 publications, altogether representing >1.2 million cells distributed 13 atlas-level tasks. We evaluated methods according scalability, usability their ability remove while retaining biological variation using 14 evaluation metrics. show highly variable selection improves the performance methods, whereas scaling pushes prioritize removal over conservation variation. Overall, scANVI, Scanorama, scVI scGen perform well, particularly complex tasks, single-cell ATAC-sequencing is strongly affected by choice feature space. Our freely available Python module benchmarking pipeline can identify optimal for new data, benchmark improve development.
Language: Английский
Citations
792