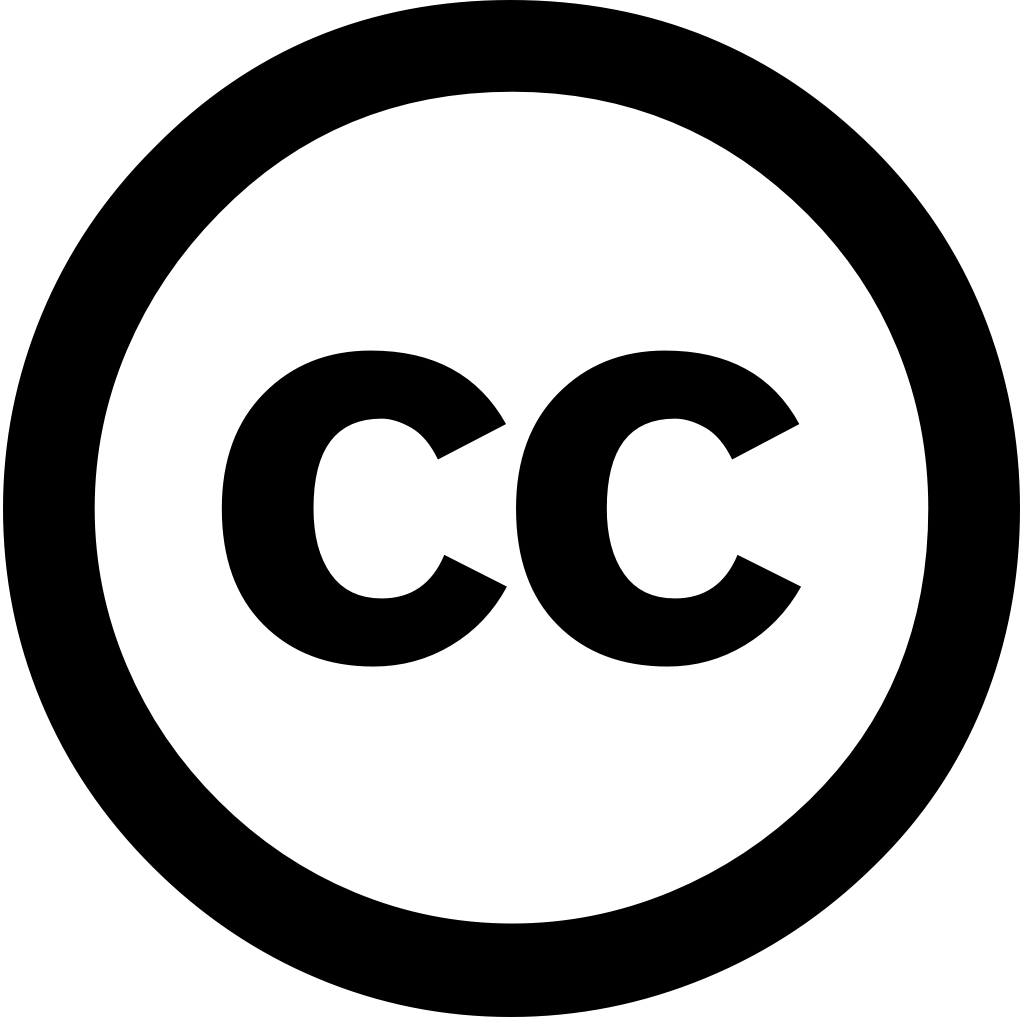
Agricultural and Forest Meteorology, Journal Year: 2024, Volume and Issue: 361, P. 110297 - 110297
Published: Nov. 23, 2024
Language: Английский
Agricultural and Forest Meteorology, Journal Year: 2024, Volume and Issue: 361, P. 110297 - 110297
Published: Nov. 23, 2024
Language: Английский
Scientific Data, Journal Year: 2024, Volume and Issue: 11(1)
Published: April 8, 2024
Rapeseed is a critical cash crop globally, and understanding its distribution can assist in refined agricultural management, ensuring sustainable vegetable oil supply, informing government decisions. China the leading consumer third-largest producer of rapeseed. However, there lack widely available, long-term, large-scale remotely sensed maps on rapeseed cultivation China. Here this study utilizes multi-source data such as satellite images, GLDAS environmental variables, land cover maps, terrain to create annual at 30 m spatial resolution from 2000 2022 (CARM30). Our product was validated using independent samples showed average F1 scores 0.869 0.971 for winter spring The CARM30 has high consistency with existing 10 20 maps. Additionally, CARM30-derived planted area significantly correlated statistics (R2 = 0.65-0.86; p < 0.001). obtained information serve reference stakeholders farmers, scientific communities, decision-makers.
Language: Английский
Citations
6World Journal of Microbiology and Biotechnology, Journal Year: 2025, Volume and Issue: 41(3)
Published: Feb. 27, 2025
Language: Английский
Citations
0Journal of Agriculture and Food Research, Journal Year: 2025, Volume and Issue: unknown, P. 101794 - 101794
Published: March 1, 2025
Language: Английский
Citations
0ISPRS Journal of Photogrammetry and Remote Sensing, Journal Year: 2025, Volume and Issue: 225, P. 163 - 179
Published: May 2, 2025
Language: Английский
Citations
0Remote Sensing of Environment, Journal Year: 2024, Volume and Issue: 315, P. 114430 - 114430
Published: Sept. 26, 2024
Language: Английский
Citations
2Remote Sensing, Journal Year: 2024, Volume and Issue: 16(15), P. 2731 - 2731
Published: July 26, 2024
Rapid and accurate mapping of soil properties in farmlands is crucial for guiding agricultural production maintaining food security. Traditional methods using spectral features from remote sensing prove valuable estimating properties, but are restricted to short periods bare occurrence within settings. Addressing the challenge predicting under crop cover, this study proposed an improved modeling framework that integrates dynamic growth information with machine learning techniques. The methodology’s robustness was tested on six key region China, including organic carbon (SOC), total nitrogen (TN), phosphorus (TP), dissolved (DOC), (DON), pH. Four experimental scenarios were established assess impact information, represented by normalized difference vegetation index (NDVI) phenological parameters. Specifically, Scenario I utilized only natural factors (terrain climate data); II added parameters based I; III incorporated time-series NDVI IV combined all variables (traditional information). These evaluated three advanced models: random forest (RF), Cubist, Extreme Gradient Boosting (XGBoost). Results demonstrated incorporating significantly model accuracy, enhancing predictions up 36% over models factors. Moreover, although both factors, contribution variable accuracy surpassed most properties. Relative importance analysis suggested derived phenology data, collectively explained 14–45% spatial variation This highlights significant benefits integrating sensing-based into property inversion crop-covered conditions, providing insights digital mapping.
Language: Английский
Citations
1Agricultural and Forest Meteorology, Journal Year: 2024, Volume and Issue: 361, P. 110297 - 110297
Published: Nov. 23, 2024
Language: Английский
Citations
1