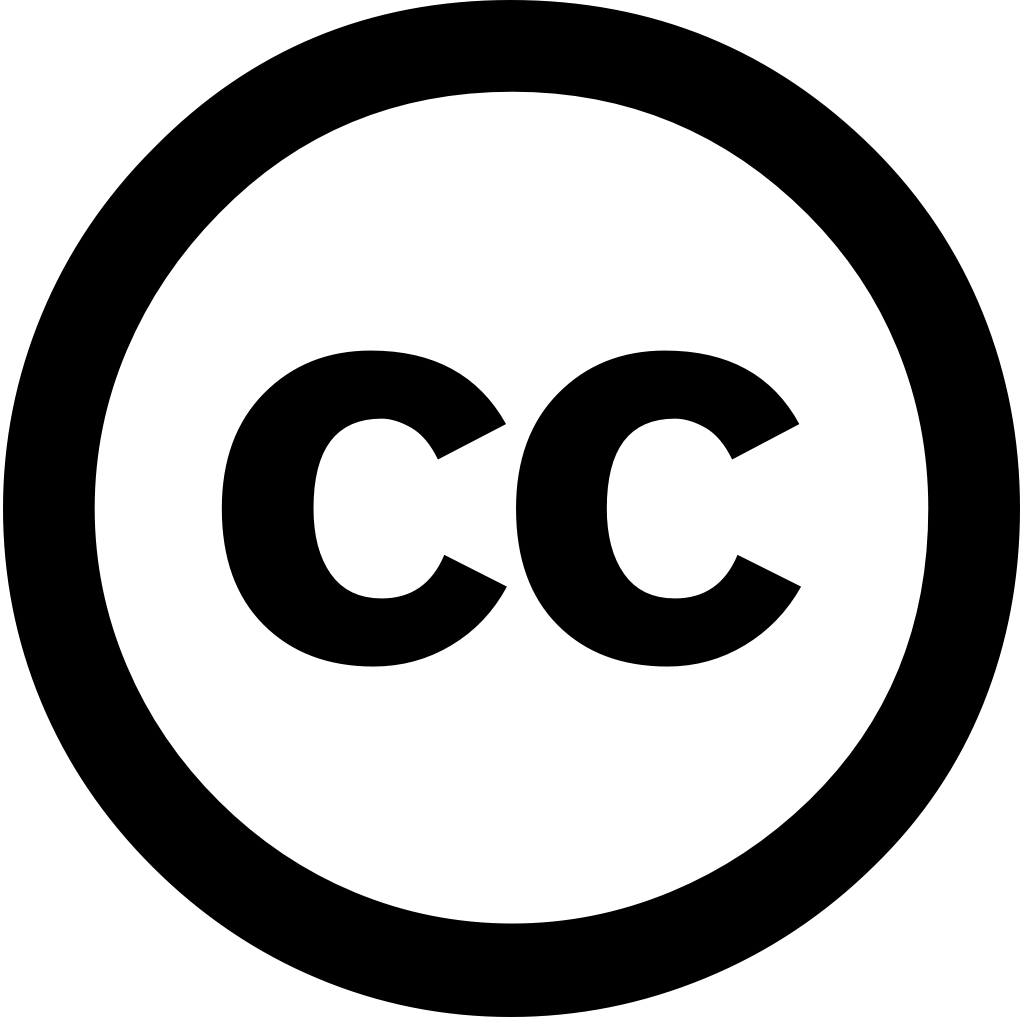
Journal of Imaging, Journal Year: 2024, Volume and Issue: 11(1), P. 6 - 6
Published: Dec. 31, 2024
Detection and segmentation of brain abnormalities using Magnetic Resonance Imaging (MRI) is an important task that, nowadays, the role AI algorithms as supporting tools well established both at research clinical-production level. While performance state-of-the-art models increasing, reaching radiologists other experts’ accuracy levels in many cases, there still a lot needed on direction in-depth transparent evaluation correct results failures, especially relation to aspects radiological practice: abnormality position, intensity level, volume. In this work, we focus analysis pre-trained U-net model trained validated MRI examinations containing four different pathologies: Tumors, Strokes, Multiple Sclerosis (MS), White Matter Hyperintensities (WMH). We present for whole abnormal volume each component inside validation set. first case, dice score coefficient (DSC), sensitivity, precision 0.76, 0.78, 0.82, respectively, were found, while second case detected segmented (True positives) 48.8% (DSC ≥ 0.5) components, partially 27.1% (0.05 > DSC 0.5), missed (False Negatives) 24.1%, it produced 25.1% False Positives. Finally, extended between True positives, Negatives, positives versus their position brain, three modalities (FLAIR, T2, T1ce)
Language: Английский