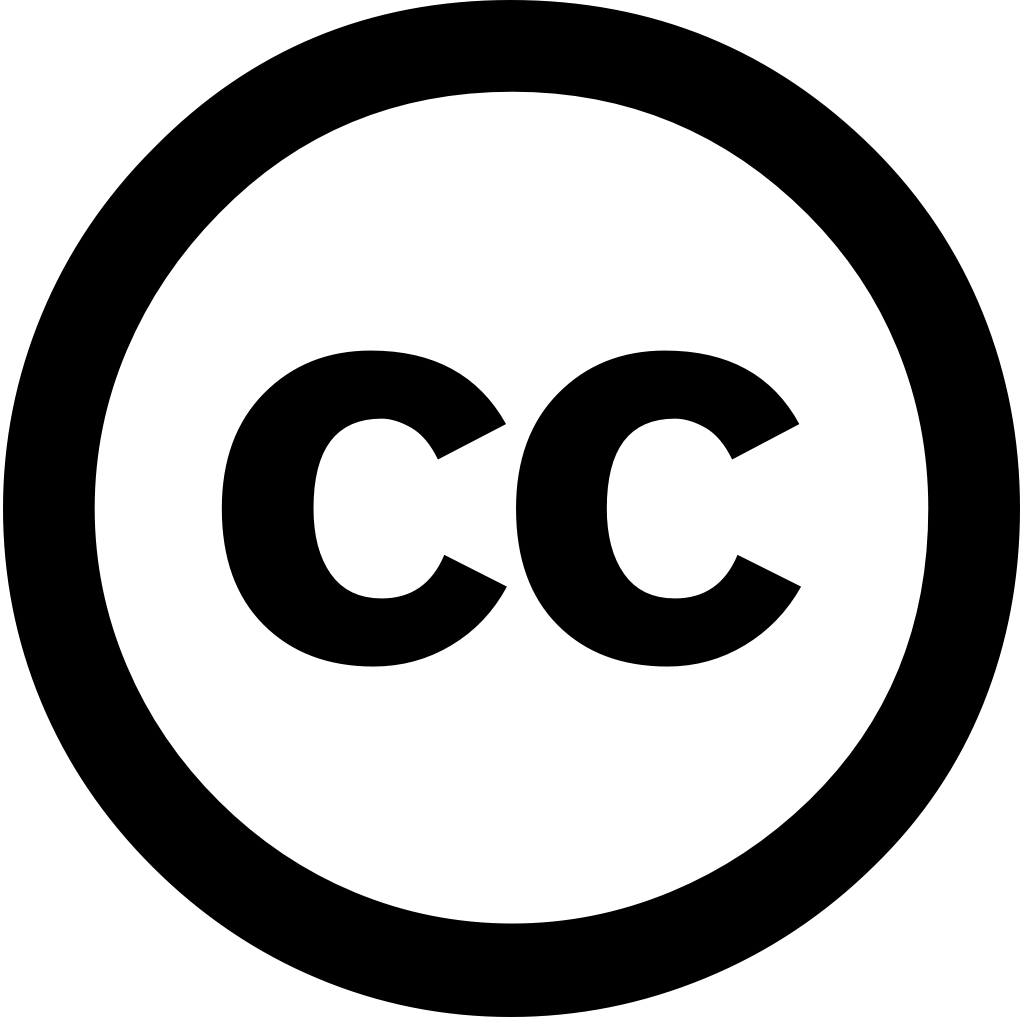
Heliyon, Journal Year: 2024, Volume and Issue: 11(1), P. e40566 - e40566
Published: Nov. 22, 2024
Language: Английский
Heliyon, Journal Year: 2024, Volume and Issue: 11(1), P. e40566 - e40566
Published: Nov. 22, 2024
Language: Английский
Pathophysiology, Journal Year: 2023, Volume and Issue: 30(4), P. 443 - 466
Published: Sept. 29, 2023
One of the primary challenges regarding chronic kidney disease (CKD) diagnosis is absence reliable methods to detect early-stage damage. A metabolomic approach expected broaden current diagnostic modalities by enabling timely detection and making prognosis more accurate. Analysis performed on urine has several advantages, such as ease collection using noninvasive its lower protein lipid content compared with other bodily fluids. This review highlights trends in applied analytical methods, major discoveries concerning pathways, investigated populations context research for CKD over past five years. Also, we are presenting approaches, instrument upgrades, sample preparation modifications that have improved parameters methods. The onset leads alterations metabolism apparent molecular composition urine. Recent works highlight prevalence metabolic pathways related tricarboxylic acid cycle amino acids. Including diverse patient cohorts, numerous techniques appropriate annotation explanation discovered biomarkers will help develop effective models different subtypes renal injury clinical applications.
Language: Английский
Citations
5Diagnostics, Journal Year: 2022, Volume and Issue: 12(11), P. 2803 - 2803
Published: Nov. 15, 2022
According to the World Health Organization (WHO), type 2 diabetes mellitus (T2DM) is a result of inefficient use insulin by body. More than 95% people with have T2DM, which largely due excess weight and physical inactivity. This study proposes an intelligent feature selection metabolites related different stages diabetes, genetic algorithms (GA) implementation support vector machines (SVMs), K-Nearest Neighbors (KNNs) Nearest Centroid (NEARCENT) dataset obtained from Instituto Mexicano del Seguro Social protocol name following: "Análisis metabolómico y transcriptómico diferencial en orina suero de pacientes pre diabéticos, diabéticos con nefropatía diabética para identificar potenciales biomarcadores pronósticos daño renal" (differential metabolomic transcriptomic analyses in urine serum pre-diabetic, diabetic nephropathy patients identify potential prognostic biomarkers kidney damage). In order analyze machine learning (ML) model most optimal for classifying some stage novelty this work provide algorithm approach that detects significant each progression. 100 were identified as between all stages; data analyzed, average accuracies five most-accurate implementations range 0.8214-0.9893 respect accuracy, providing precise tool detections backing up diagnosis constructed entirely metabolomics. By progression, these extremely are follows: "Cer(d18:1/24:1) i2", "PC(20:3-OH/P-18:1)", "Ganoderic acid C2", "TG(16:0/17:1/18:1)" "GPEtn(18:0/20:4)".
Language: Английский
Citations
7Kidney Research and Clinical Practice, Journal Year: 2023, Volume and Issue: 42(4), P. 409 - 411
Published: July 28, 2023
See the article "Urine myo-inositol as a novel prognostic biomarker for diabetic kidney disease: targeted metabolomics study using nuclear magnetic resonance" in Volume 42 on page 445.
Language: Английский
Citations
4Journal of Nephrology, Journal Year: 2024, Volume and Issue: unknown
Published: Aug. 12, 2024
Abstract Diabetic kidney disease (DKD) is a significant complication of type 2 diabetes, posing global health risk. Detecting and predicting diabetic at an early stage crucial for timely interventions improved patient outcomes. Artificial intelligence (AI) has demonstrated promise in healthcare, several tools have recently been developed that utilize Machine Learning with clinical data to detect predict DKD. This review aims explore the current landscape AI machine learning applications DKD, specifically examining existing literature on risk scores approaches DKD development. A search was conducted using Medline (PubMed), Google Scholar, Scopus databases until July 2023. Relevant keywords were used extract studies described role The revealed successfully progression, outperforming traditional score models. intelligence-driven research extends beyond prediction models, offering opportunities integrating genetic epigenetic data, advancing understanding disease’s molecular basis, personalizing treatment strategies, fostering development novel drugs. However, challenges remain, including requirement large datasets lack standardization AI-driven potential revolutionize management care patients, surpassing limitations methods reliant knowledge. Future should address associated focus developing practice. Graphical abstract
Language: Английский
Citations
1Journal of Cellular Biochemistry, Journal Year: 2024, Volume and Issue: unknown
Published: Sept. 30, 2024
ABSTRACT Diabetic Kidney Disease (DKD), a frequent consequence of diabetes, has substantial implications for both morbidity and mortality rates, prompting the exploration new metabolic biomarkers due to limitations in current methods like creatinine albumin measurements. Pentraxin 3 (PTX3) shows promise assessing renal inflammation DKD. This study investigates how DKD metabolites could influence PTX3 expression through molecular docking, ADMET profiling, dynamic simulation. Network pathway analyses were conducted explore metabolite interactions with genes their contributions pathogenesis. Thirty‐three DKD‐associated screened, using pentoxifylline (PEN) as reference. The pharmacokinetic properties these compounds evaluated docking profiling. Molecular dynamics simulations over 200 ns assessed stability (apo), PRE‐PTX3 complex, PEN‐PTX3 across multiple parameters. Cytoscape identified 1082 nodes 1381 edges linking genes. KEGG analysis underscored PTX3's role inflammation. revealed pregnenolone sulfate (PRE) highest binding affinity (−6.25 kcal/mol), followed by hydrocortisone (−6.03 kcal/mol) 2‐arachidonoylglycerol (−5.92 compared PEN (−5.35 kcal/mol). profiling selected PRE simulation alongside PEN. Analysis RMSD, RMSF, RG, SASA, H‐bond, PCA, FEL, MM‐PBSA indicated stable complex behavior time. Our findings suggest that increasing levels be beneficial managing DKD, potentially isolating from fungal sources, synthesizing it dietary supplements, or enhancing endogenous synthesis within body.
Language: Английский
Citations
1The Korean Journal of Internal Medicine, Journal Year: 2024, Volume and Issue: 39(6), P. 898 - 905
Published: Oct. 22, 2024
Diabetic nephropathy (DN), a leading cause of chronic kidney disease and end-stage (ESKD), poses global health challenges given its increasing prevalence. DN increases the risk mortality cardiovascular events. Early identification appropriate management are crucial. However, current diagnostic methods rely on general traditional markers, highlighting need for DN-specific diagnostics. Metabolomics, study small molecules produced by metabolic activity, promises to identify specific biomarkers that distinguish from other diseases, decode underlying mechanisms, predict course. Profound changes in pathways apparent individuals with DN, alterations tricarboxylic acid cycle amino lipid metabolism, suggestive mitochondrial dysfunction. Metabolomics aids prediction progression; several metabolites serve as indicators renal functional decline ESKD. Integration such information omics data will further enhance our understanding paving way personalized treatment. In summary, metabolomics multi-omics offer valuable insights into promising prognostic tools.
Language: Английский
Citations
1Current Medical Research and Opinion, Journal Year: 2024, Volume and Issue: unknown, P. 1 - 31
Published: Oct. 30, 2024
The purpose of this study was to conduct a systematic investigation the potential artificial intelligence (AI) models in prediction, detection diagnostic biomarkers, and progression diabetic kidney disease (DKD). In addition, we compared performance non-logistic regression (LR) machine learning (ML) conventional LR prediction models.
Language: Английский
Citations
1Diabetologia, Journal Year: 2024, Volume and Issue: unknown
Published: Dec. 2, 2024
Language: Английский
Citations
1Expert Review of Proteomics, Journal Year: 2023, Volume and Issue: 20(12), P. 451 - 467
Published: Dec. 2, 2023
Introduction Diabetes Mellitus (DM) is a chronic heterogeneous metabolic disorder characterized by hyperglycemia due to the destruction of insulin-producing pancreatic β cells and/or insulin resistance. It now considered global epidemic disease associated with serious threats patient's life. Understanding pathways involved in pathogenesis and progression important would improve prevention management strategies. Metabolomics an emerging field research that offers valuable insights into perturbation diseases, including DM.
Language: Английский
Citations
3International Journal of Molecular Sciences, Journal Year: 2023, Volume and Issue: 24(11), P. 9412 - 9412
Published: May 28, 2023
Diabetic kidney disease (DKD) is a complication that strongly increases the risk of end-stage and cardiovascular events. The identification novel, highly sensitive, specific early biomarkers to identify DKD patients predict function decline pivotal aim translational medicine. In previous study, after high-throughput approach, we identified in 69 diabetic 5 serum mitochondrial RNAs (MT-ATP6, MT-ATP8, MT-COX3, MT-ND1, MT-RNR1) progressively downregulated with increasing eGFR stages. Here, analyzed protein concentrations three well-validated biomarkers: TNFRI, TNFRII, KIM-1. were gradually upregulated from G1 G2 G3 patients. All correlated creatinine, eGFR, BUN. Performing multilogistic analyses, found that, respect single biomarkers, combination between (I) TNFRI or KIM-1 each RNA transcript (II) TNFRII MT-ATP6, MT-COX-3, MT-ND1 determined an outstanding improvement diagnostic performance versus patient identification, reaching values most cases above 0.9 even equal 1. AUC was also evaluated normoalbuminuric microalbuminuric considered separately. This study proposes promising multikind marker panel associated impairment DKD.
Language: Английский
Citations
2