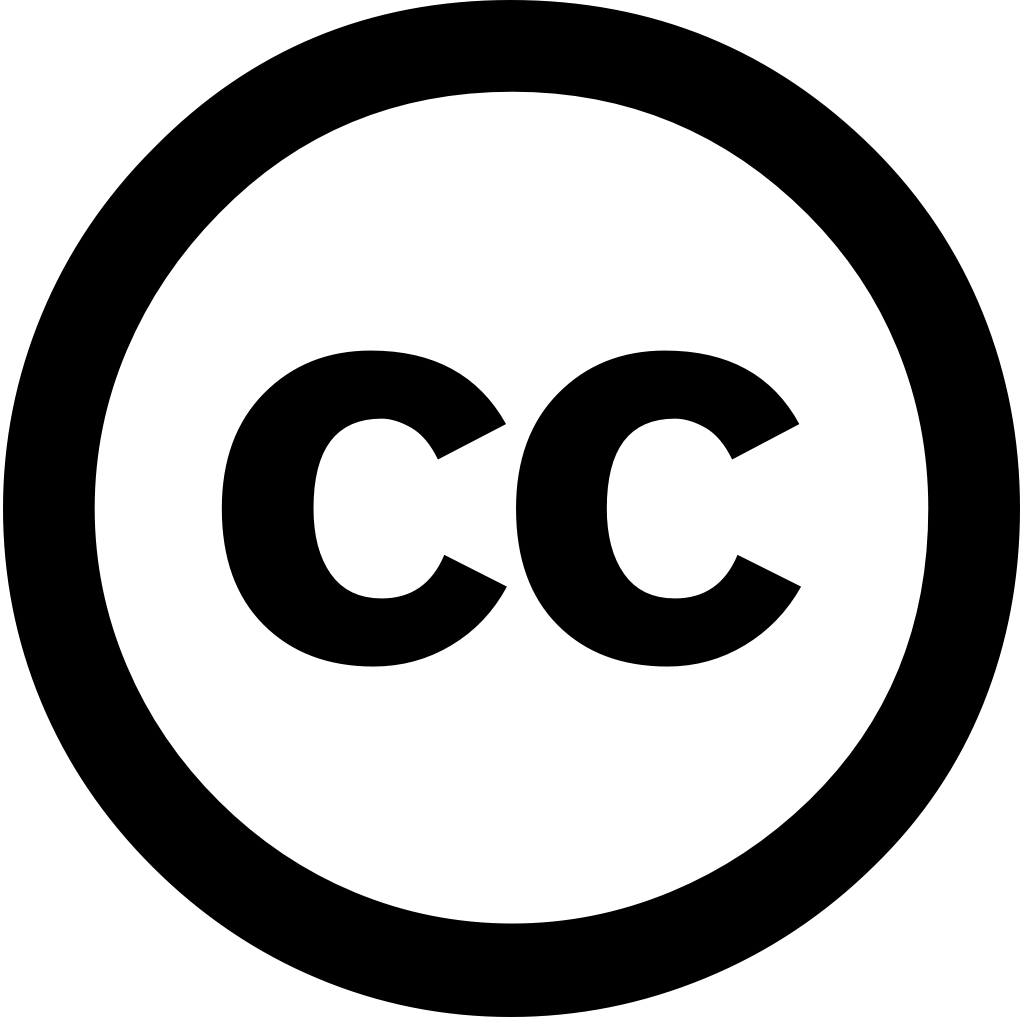
ENVIRONMENTAL SYSTEMS RESEARCH, Journal Year: 2024, Volume and Issue: 13(1)
Published: Sept. 3, 2024
The continued threat from climate change and human impacts on water resources demands high-resolution continuous hydrological data accessibility for predicting trends availability. This study proposes a novel threefold downscaling method based machine learning (ML) which integrates: normalization; interaction of hydrometeorological variables; the application time series split cross-validation that produces high spatial resolution groundwater storage anomaly (GWSA) dataset Gravity Recovery Climate Experiment (GRACE) its successor mission, GRACE Follow-On (GRACE-FO). In study, relationship between terrestrial (TWSA) other land surface variables (e.g., vegetation coverage, temperature, precipitation, in situ level data) is leveraged to downscale GWSA. predicted downscaled GWSA datasets were tested using monthly observations, results showed model satisfactorily reproduced temporal variations area, with Nash-Sutcliffe efficiency (NSE) correlation coefficient values 0.8674 (random forest) 0.7909 (XGBoost), respectively. Evapotranspiration was most influential predictor variable random forest model, whereas it rainfall XGBoost model. particular, excelled aligning closely observed patterns, as evidenced by positive correlations lower error metrics (Mean Absolute Error (MAE) 54.78 mm; R-squared (R²) 0.8674). 5 km (based decreasing trend associated variability pattern. An increase drought severity during El Niño lengthened full recovery historical trends. Furthermore, lag occurrence precipitation recharge likely controlled intensity characteristics aquifer. Projected increases could further times response droughts changing climate, resetting new tipping condition. Therefore, adaptation strategies must recognise less will be available supplement supply droughts.
Language: Английский