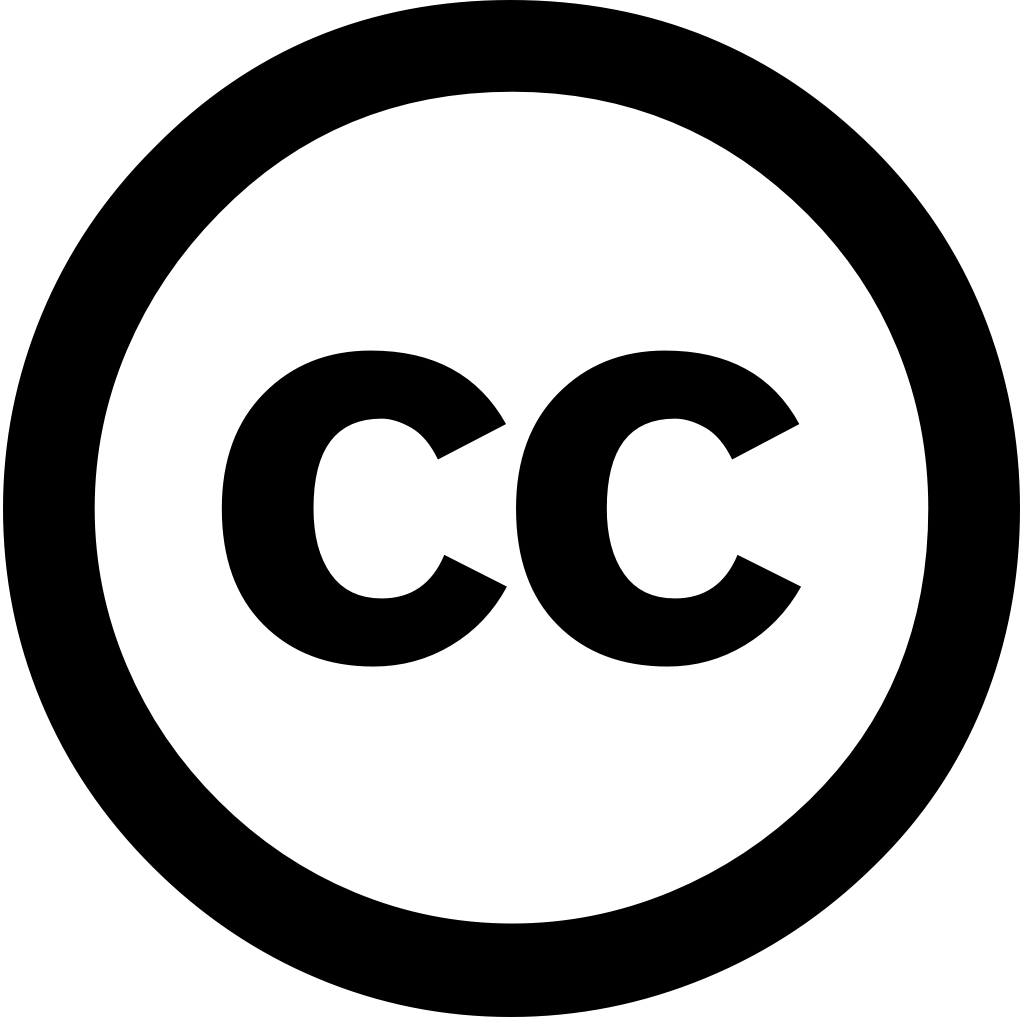
Scientific Reports, Journal Year: 2024, Volume and Issue: 14(1)
Published: Oct. 28, 2024
Language: Английский
Scientific Reports, Journal Year: 2024, Volume and Issue: 14(1)
Published: Oct. 28, 2024
Language: Английский
Scientific Reports, Journal Year: 2025, Volume and Issue: 15(1)
Published: Jan. 2, 2025
Language: Английский
Citations
2Scientific Reports, Journal Year: 2024, Volume and Issue: 14(1)
Published: Oct. 9, 2024
Accurate runoff forecasting is of great significance for water resource allocation flood control and disaster reduction. However, due to the inherent strong randomness sequences, this task faces significant challenges. To address challenge, study proposes a new SMGformer forecast model. The model integrates Seasonal Trend decomposition using Loess (STL), Informer's Encoder layer, Bidirectional Gated Recurrent Unit (BiGRU), Multi-head self-attention (MHSA). Firstly, in response nonlinear non-stationary characteristics sequence, STL used extract sequence's trend, period, residual terms, multi-feature set based on 'sequence-sequence' constructed as input model, providing foundation subsequent models capture evolution runoff. key features are then captured layer. Next, BiGRU layer learn temporal information these features. further optimize output MHSA mechanism introduced emphasize impact important information. Finally, accurate achieved by transforming through Fully connected verify effectiveness proposed monthly data from two hydrological stations China selected, eight compare performance results show that compared with Informer 1th step MAE decreases 42.2% 36.6%, respectively; RMSE 37.9% 43.6% NSE increases 0.936 0.975 0.487 0.837, respectively. In addition, KGE at 3th 0.960 0.805, both which can maintain above 0.8. Therefore, accurately sequence extend effective period
Language: Английский
Citations
9Journal of Hydrology, Journal Year: 2025, Volume and Issue: unknown, P. 132918 - 132918
Published: Feb. 1, 2025
Language: Английский
Citations
1Expert Systems with Applications, Journal Year: 2025, Volume and Issue: unknown, P. 127228 - 127228
Published: March 1, 2025
Language: Английский
Citations
1Sustainability, Journal Year: 2024, Volume and Issue: 16(19), P. 8699 - 8699
Published: Oct. 9, 2024
The establishment of an accurate and reliable predictive model is essential for water resources planning management. Standalone models, such as physics-based hydrological models or data-driven have their specific applications, strengths, limitations. In this study, a hybrid (namely SWAT-Transformer) was developed by coupling the Soil Water Assessment Tool (SWAT) with Transformer to enhance monthly streamflow prediction accuracy. SWAT first constructed calibrated, then its outputs are used part inputs Transformer. By correcting errors using Transformer, two effectively coupled. Monthly runoff data at Yan’an Ganguyi stations on Yan River, first-order tributary Yellow River Basin, were evaluate proposed model’s performance. results indicated that performed well in predicting high flows but poorly low flows. contrast, able capture low-flow period information more accurately outperformed overall. SWAT-Transformer could correct predictions overcome limitations single model. integrating SWAT’s detailed physical process portrayal Transformer’s powerful time-series analysis, coupled significantly improved offer optimal resource management, which crucial sustainable economic societal development.
Language: Английский
Citations
4Journal on Computing and Cultural Heritage, Journal Year: 2025, Volume and Issue: unknown
Published: Jan. 31, 2025
Jiayuguan, the first majestic pass at western end of Ming Dynasty's Great Wall, is an important world cultural heritage site with high historical, scientific, social, and artistic value. It primarily constructed rammed earth has existed in form relics for over 600 years. Due to prolonged exposure natural environmental factors such as rainfall, wind-borne sand, temperature variations, typical crack diseases have developed significantly, posing a threat overall stability earthen site. To ensure long-term safety sites, we explore primary influencing progression damages these sites using monitoring techniques. Additionally, employing data mining techniques analyze development trends predictive warnings will prove be challenging task. In this context, paper collects on occurrence environment Jiayuguan site, uses wavelet correlation analysis method Boruta feature screening find main that affect diseases. Then, combining factor variables, it deep learning LSTM, GRU, Transformer transfer Transformer-LSTM, GRU-Transformer, A-SBiLSTM predict trend north wall beacon tower respectively. can seen (i) model RMSE=0.0154, R 2 =0.973; (ii) Transformer-LSTM RMES=0.0290, =0.937; (iii) are closer actual SD value than other models. The research results show models accuracy, good stability, optimal prediction effects. They suitable predicting These application potential related fields provide references subsequent research.
Language: Английский
Citations
0Water, Journal Year: 2025, Volume and Issue: 17(6), P. 907 - 907
Published: March 20, 2025
Accurate forecasting of river flows is essential for effective water resource management, flood risk reduction and environmental protection. The ongoing effects climate change, in particular the shift precipitation patterns increasing frequency extreme weather events, necessitate development advanced models. This study investigates application long short-term memory (LSTM) neural networks predicting runoff Velika Morava catchment Serbia, representing a pioneering LSTM this region. uses daily runoff, temperature data from 1961 to 2020, interpolated using inverse distance weighting method. model, which was optimized trial-and-error approach, showed high prediction accuracy. For station, model mean square error (MSE) 2936.55 an R2 0.85 test phase. findings highlight effectiveness capturing nonlinear hydrological dynamics, temporal dependencies regional variations. underlines potential models improve management strategies Western Balkans.
Language: Английский
Citations
0Water Resources Management, Journal Year: 2025, Volume and Issue: unknown
Published: March 22, 2025
Language: Английский
Citations
0Journal of Hydrology, Journal Year: 2025, Volume and Issue: unknown, P. 133221 - 133221
Published: April 1, 2025
Language: Английский
Citations
0Results in Engineering, Journal Year: 2025, Volume and Issue: unknown, P. 104956 - 104956
Published: April 1, 2025
Language: Английский
Citations
0