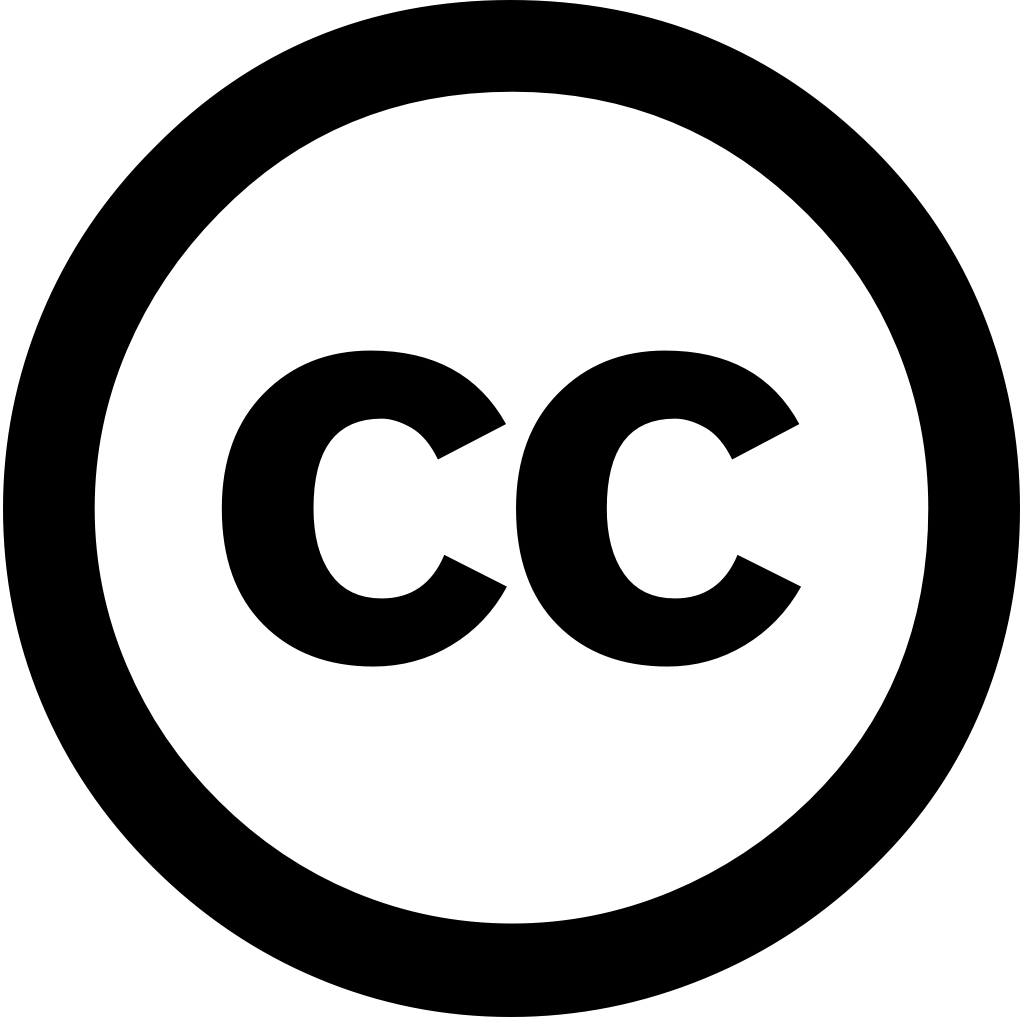
Frontiers in Endocrinology, Journal Year: 2025, Volume and Issue: 16
Published: Jan. 14, 2025
1. IntroductionThe field of clinical endocrinology, as well healthcare in general, is facing a transformative change by new technologies, especially artificial intelligence (AI). AI holds the promise to dramatically improve way we screen, diagnose, treat, monitor, and coach patients (1, 2). Not only will tools make flow endocrine decision-making faster more reliable, use opens personalized treatment plans tailored individual patient characteristics (3, 4). within computer science that encompasses machine learning (ML). ML uses mathematical algorithms designed predictions or classifications. These models are typically trained on known, labeled datasets iteratively enhanced gain capability accurate unseen data (5). Deep (DL), subset ML, complex mimic human central nervous system. DL entails neural networks (ANNs). ANNs consist interconnected layers pass information optimize minimizing error (6). Once trained, can process vast perform tasks such predictions, classifications, even advanced applications like large language (LLMs), vision, multimedia generation from text inputs (7-9). We anticipating an unprecedented disruption endocrinology AI. Nevertheless, most clinicians lack proper understanding potential one hand, shortcomings caveats other hand. A balanced comprehension underpinnings imperative maximize its benefits. Hence, providers must familiarize themselves with this technology but also understand limitations. Table 1 gives overview differences between AI-based conventional methods endocrinology.The aim paper give future direction domain diabetes. 2. Improved Risk AssessmentThe importance timely risk assessment well-established, significantly enhance both speed efficiency. For instance, Wändell colleagues created tool evaluate having de novo diabetes using stochastic gradient boosting model. Area under curve (AUC) was 0.773 0.825, indicating good discriminatory power (10). The important factors were identified being arterial hypertension obesity. model, adults over 30 years old Stokholm, Sweden included. No given relation ethnicity. Yousef co-workers used interpretable model for prediction undiagnosed type 2 mellitus. subjects study rural screening clinic Albury, Australia. Ethnicity not recorded. They two different Isolated Forest (iForest) algorithms. first basis BMI (body mass index), blood glucose level, triglycerides. second iForest same parameters, supplemented biomarkers oxidative stress (8-isoprostane, 8-hydroxydeoxyguanosine, oxidized glutathione), inflammation (interleukin-6, interleukin-10, interleukin-1β, insulin-like growth factor-1), mitochondrial dysfunction (humanin, MOTS-c, P66Shc). latter outperformed former one; F1-score increased 0.61 0.81 (11). In another study, Nabrdalik et al. stratification MASLD (metabolic dysfunction-associated steatotic liver disease) Patients recruited diabetology ward hospital Zabrze, Poland. initially 80 parameters. To determine discriminative predictors, feature selection conducted chi-squared test. stability rendered variables assured repeating Monte-Carlo simulation 1,000 times. independent employed multiple logistic regression order predict occurrence (12). has been hypoglycemia Cichosz developed binary classification XGBoost (extreme boost) algorithm aim, CGM (continuous monitoring) 206 United States. More than 90% white, non-Hispanic. Their median age 68 years. validated cohorts. total 61,470 weeks included analysis. demonstrated strong performance, ROC-AUCs (area receiver operating characteristic curve) ranging 0.90 across validation cohorts (13). shows osteoporosis assessment. Hong argue could be very beneficial prone osteoporotic fractures. An individualized approach management believed reach help cutting-edge (14). This potentially reduce morbidity mortality, costs alleviate workload providers. Assessment thyroid nodules challenging at Distinguishing benign malignancy paramount care. Wildman-Tobriner therefore system ultrasound images nodules, imaging reporting (AI TI-RADS). 378 320 study. Subjects' collected electronic health records Duke University Medical Center, Durham, NC, demographics mentioned, besides sex. All underwent fine needle aspiration cytology. Results TI-RADS comparable ACR (American College Radiology Thyroid Imaging Reporting Data Systems) (15). AI-driven expected diagnostic performance near future. Still challenges remain, inconsistent ratings physicians, uncertainty cytopathological diagnosis difficulty discriminating follicular lesions (16). hold promising As availability increases, comprehensive emerge. 3. Better Faster DiagnosisThe prove great benefit diagnostics. diagnosis, endocrinologists rely presentation, history, lab results technical examinations, medical imaging. Usually quite straightforward. However, occasionally doctors confronted cases where might increase accuracy. Chia co-workers, example, diagnose retinopathy (DR) indigenous Australian patients. Aboriginal Community Controlled Health Service located metropolitan area Perth, Western retina specialist terms sensitivity; specificity (17). Joseph performed systematic review which 34 studies carried out Asia (57%), Europe (20%), North America (12%), Australia (7%), Africa (2%) South (2%). findings indicate fact acceptable DR. Fundus compared graders. software conjunction fundus camera indeed facilitate work ophthalmologists accuracy (18). Wu linear random forest (RF) laboratory 479 patients, mellitus, neuropathy lower limb disease Tongji Hospital, Shanghai, China. proved diagnosing comparison models. Coversely, RF revealed suitable detecting peripheral vascular (19). some crucial outcome. Here automatic interpretation provoke alarm, so physician swiftly attend patient. Tirado-Aguilar underscored gestational avoid adverse neonatal maternal outcomes (20). become normal daily practice stethoscope today. bound see staggering progression come. 4. Personalized treatmentsOne opportunities possibility forge distinctions. medicine thus coming reach. decades come, leave one-size-fits-all shift towards optimized therapies highest efficiency while limiting effects. Long models, 9 responders versus non-responders metformin Beijing Friendship Capital University, Beijing, F1 scores XGBoost, KNN (K-Nearest Neighbors) , NB (Naive Bayes), SVM (Support Vector Machine) 0.830, 0.517, 0.898, 0.864 0.475, respectively (21). strategy expanded drugs compose best possible each Popova performing trial app women mellitus control their glycemic levels. outpatient department Perinatal Center Almazov National Research antenatal clinics, all Saint Petersburg, Russia. Prognoses level hour postprandial every time they input meal (22). anticipate adjust current manner hyperglycemia. Closed loop pancreas systems exciting greatly quality life Several already (23). helpful pathologies assisting test prescriptions, guide them interpretation, (24). mainstream once AI-tools sufficiently accepted. 5. Monitoring distanceThe too overloaded, going problem worse future; AI-enhanced distance monitoring mitigate problem. part solution may lie wearable devices sensors monitor without need direct oversight professional. Promphet introduced sensing device smartphone levels applying regressor Subject mentioned empower take doctor (25). diverse Juyal detect subtle patterns real (26). Besides kind sophisticated increasing wearables nowadays. provide interesting development forthcoming distinguishing immediate attention, those withstand delay. However technologies are, problems remain solved. Privacy concerns necessitate high encryption. And cost subject discussion. It conceivable, however, savings would offset investment long run. 6. Ethical considerations limitations AIDespite enormous there hinder widespread adoption technology. limitation dependent they're on. Missing data, incorrectly errors, mistakes rise inaccurate (27). constraint lies generalizability problematic certain ethnic groups, geographical locations, social strata, gender, category, apply aligned training operates (28). third hurdle privacy regarding sensitive (29). Certain regulations have adhered to. complicate inhibit application situations. last impediment relates issue liability. remains unclear whether responsible outcomes, if company providing final responsibility (30). There still ethical questions answered before fully embraced endocrinology. 7. ConclusionsIn conclusion, revolutionize enhancing offering treatments, allowing remote monitoring. transformation realized, professionals proactively embrace AI, benefits Without adequate preparation comprehension, miss pivotal opportunity care through groundbreaking essential engage responsibly, ensuring equipped navigate promises practical challenges.
Language: Английский