The application of Simulated Annealing Algorithm, Firefly Algorithm, Invasive Weed Optimization, and Shuffled Frog Leaping Algorithm for prediction of Water Quality Index
Abstract
Groundwater
is
a
vital
resource
for
drinking
water,
agriculture,
and
industry
worldwide.
Effective
groundwater
quality
management
crucial
safeguarding
public
health
ensuring
ecological
sustainability.
Hydrogeochemical
data
modeling
widely
utilized
to
predict
using
various
approaches.
The
method
proposed
in
this
study
leverages
an
intelligent
model
combined
with
chemical
compositions.
Sampling
was
conducted
from
175
agricultural
wells
the
Arak
Plain.
By
utilizing
hydrogeochemical
performing
correlation
sensitivity
analyses,
key
compositions
were
identified:
Ca²⁺,
Cl⁻,
EC,
HCO₃⁻,
K⁺,
Mg²⁺,
Na⁺,
pH,
SO₄²⁻,
TDS,
NO₃⁻.The
predicted
Water
Quality
Index
(WQI)
values
composition
artificial
neural
network
(ANN)
model.
of
served
as
model’s
input,
while
WQI
treated
output.
To
enhance
ANN's
accuracy,
several
optimization
algorithms
used,
including:
Simulated
Annealing
Algorithm
(SAA),
Firefly
(FA),
Invasive
Weed
Optimization
(IWO),
Shuffled
Frog
Leaping
(SFLA).The
comparison
results
indicated
that
ANN-SAA
outperformed
other
models.
R²
MSE
predicting
training
data:
=
0.8275,
0.0303
test
0.7357,
0.0371.These
demonstrate
provides
reliable
accurate
index
values,
offering
valuable
tool
assessment
management.
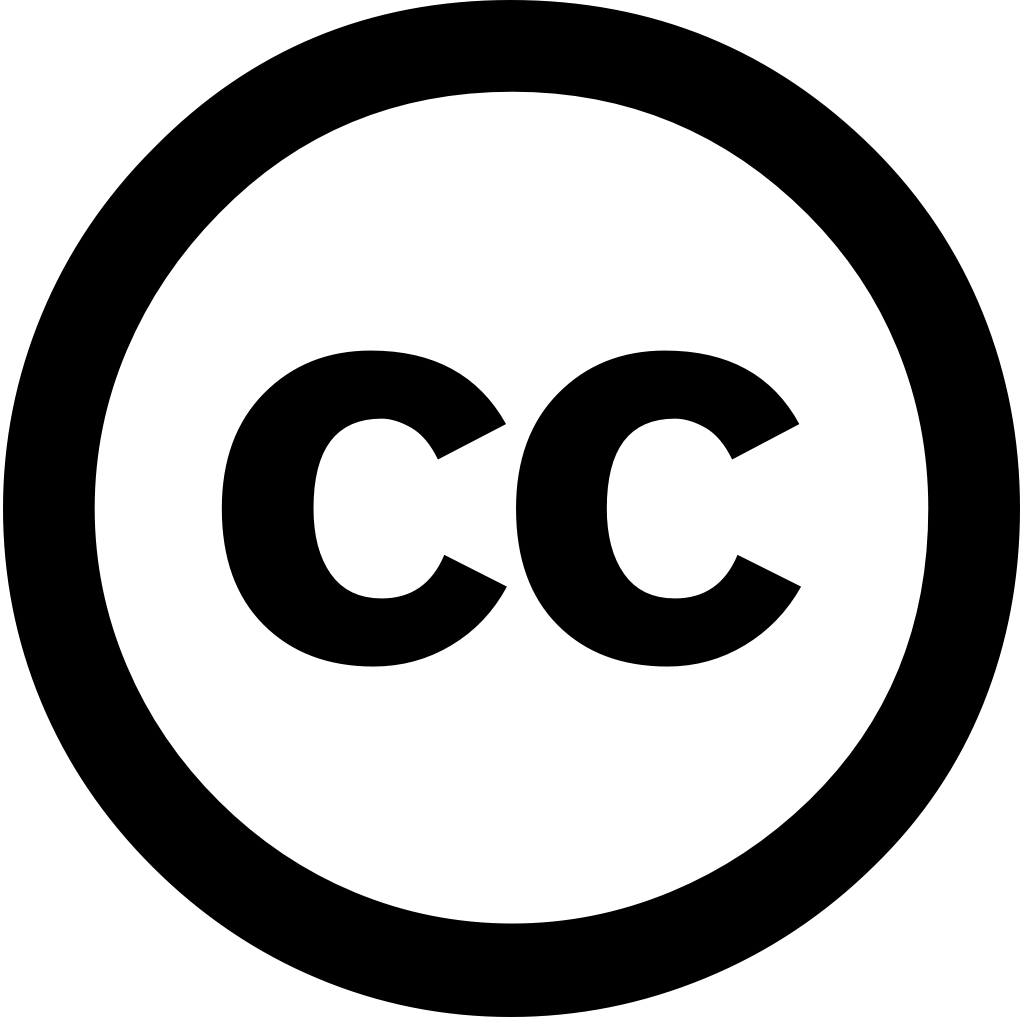
Research Square (Research Square), Journal Year: 2025, Volume and Issue: unknown
Published: Feb. 10, 2025
Language: Английский