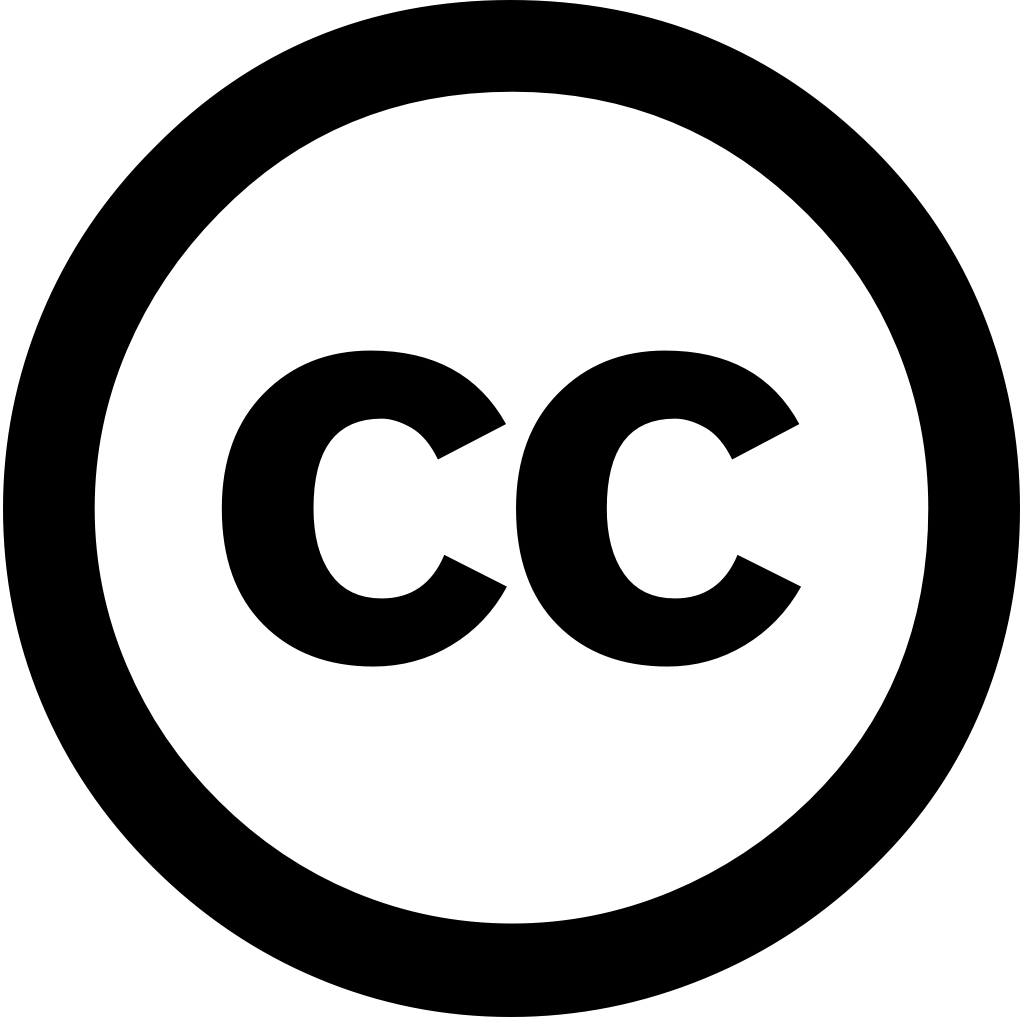
Medicina, Journal Year: 2025, Volume and Issue: 61(5), P. 809 - 809
Published: April 26, 2025
Background and Objectives: Breast cancer is a leading global health challenge, where early detection essential for improving survival outcomes. Two-dimensional (2D) mammography the established standard breast screening; however, its diagnostic accuracy limited by factors such as density inter-reader variability. Recent advances in artificial intelligence (AI) have shown promise enhancing radiological interpretation. This study aimed to assess utility of AI lesion classification 2D mammography. Materials Methods: A retrospective analysis was performed on dataset 578 mammographic images obtained from single radiology center. The consisted 36% pathologic 64% normal cases, partitioned into training (403 images), validation (87 test (88 images) sets. Image preprocessing involved grayscale conversion, contrast-limited adaptive histogram equalization (CLAHE), noise reduction, sharpening. convolutional neural network (CNN) model developed using transfer learning with ResNet50. Model performance evaluated sensitivity, specificity, accuracy, area under receiver operating characteristic (AUC-ROC) curve. Results: achieved an overall 88.5% AUC-ROC 0.93, demonstrating strong discriminative capability between cases. Notably, exhibited high specificity 92.7%, contributing reduction false positives improved screening efficiency. Conclusions: AI-assisted holds potential enhance reducing false-positive findings. Although further optimization required minimize negatives. Future efforts should aim improve incorporate multimodal imaging techniques, validate results across larger, multicenter prospective cohorts ensure effective integration clinical workflows.
Language: Английский