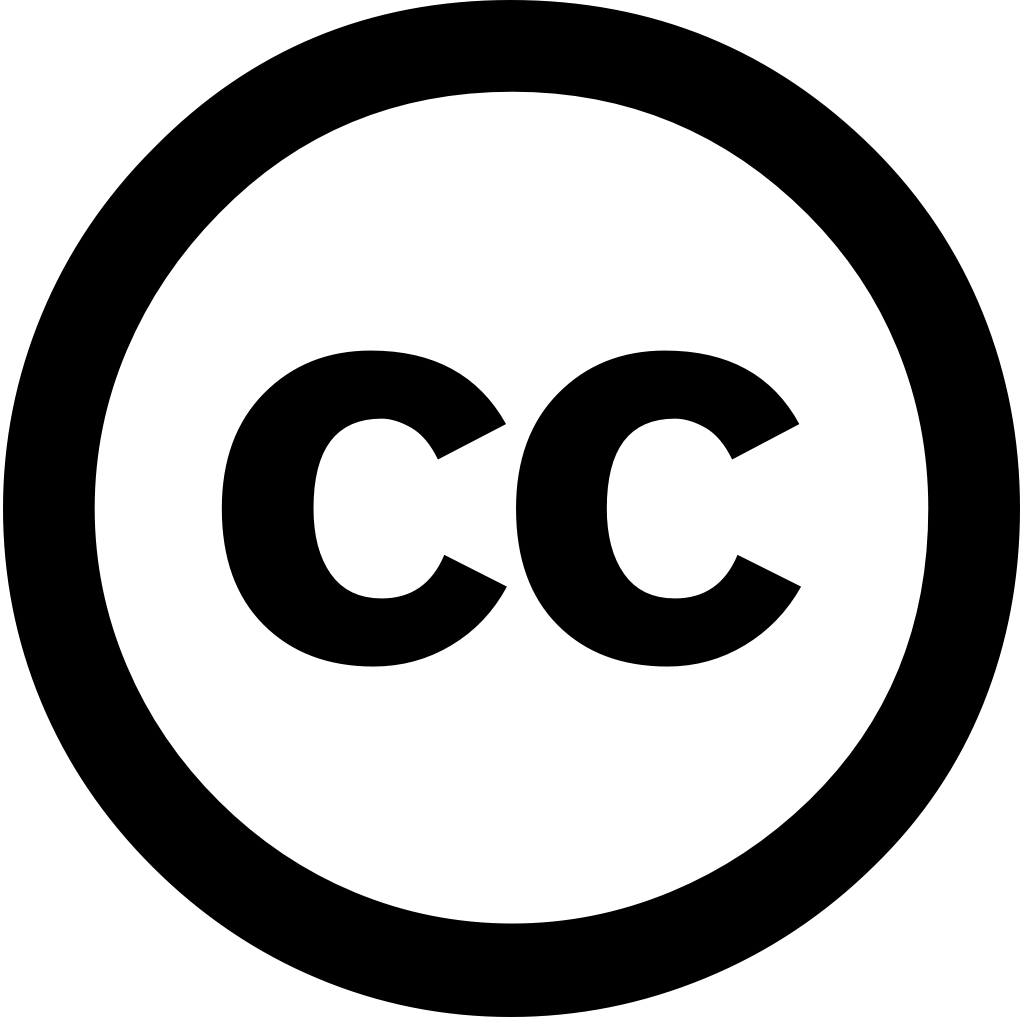
Scientific Reports, Journal Year: 2025, Volume and Issue: 15(1)
Published: March 24, 2025
This work aims to use powerful machine learning methods predict salicylic acid solubility in various solvents as function of pressure and temperature. Using a dataset consisting 217 data points 15 input features, the analysis was performed using variables including pressure, temperature, 13 different integral aspects. The considered for this study included: ethanol, water, methanol, ethyl acetate, PEG 300, 1,4-dioxane, 1-propanol, 1-butanol, 1-pentanol, 1-hexanol, 1-heptanol, acetonitrile, acetone. Temperature between 243.15 323.15 K, 90 101.32 kPa were used models. commenced with comprehensive pre-processing phase, which involved normalizing Min–Max Scaler. followed by removal outliers k-Nearest Neighbors Outlier Detection (KNNOD) technique. Several models, Convolutional Neural Networks (CNNs), Polynomial Regression (PR), Kernel Ridge (KRR), employed acid. Hyperband method utilized hyper-parameter optimization, ensuring optimal performance each model dynamically allocating computational resources. effectiveness these models evaluated metrics such R2 scores, MSE, MAE. results revealed that CNNs outperformed other high degree accuracy (R2 score 0.989, MSE 4.161203E−05, MAE 3.760119 E−03), while KRR achieved an 0.913873. underline robustness preprocessing methods, selection, tuning attainment accurate predictions, making useful contributions area prediction solvent environments.
Language: Английский