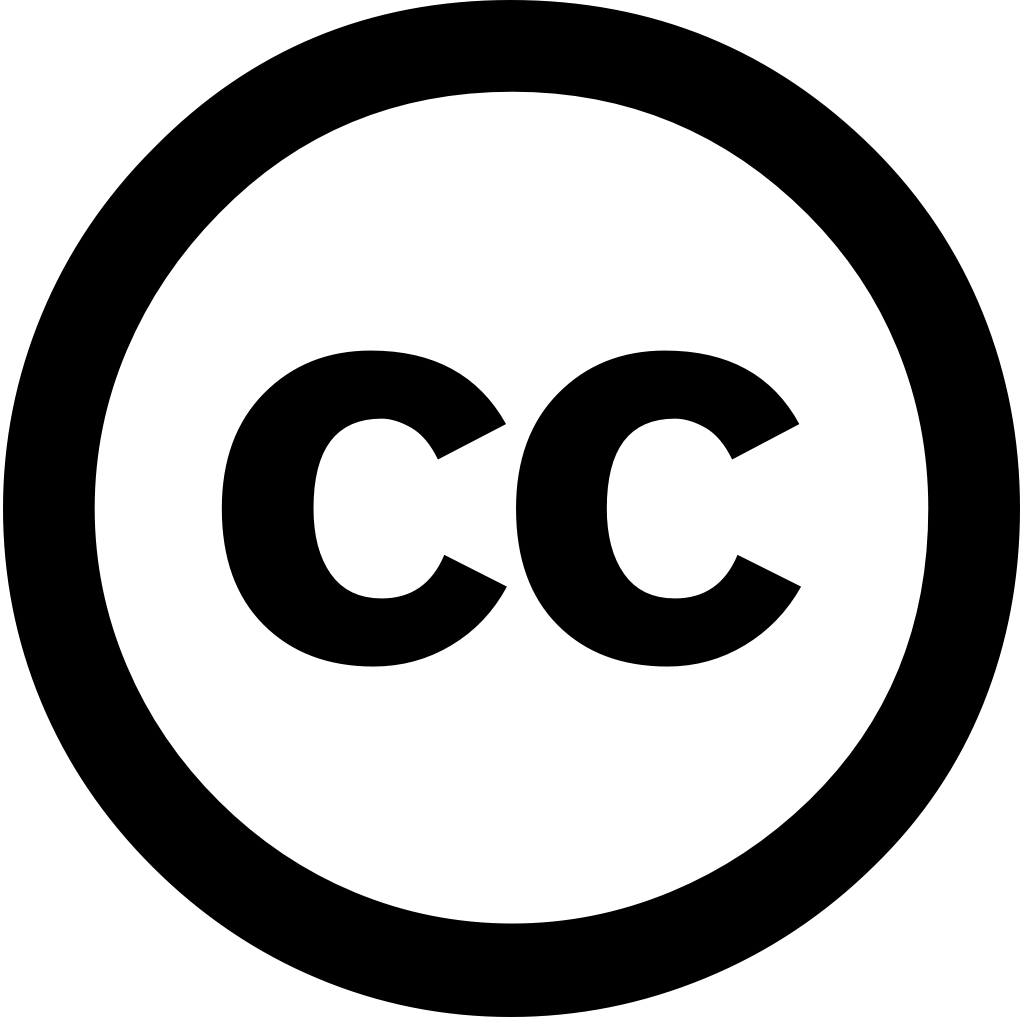
APL Machine Learning, Journal Year: 2025, Volume and Issue: 3(2)
Published: April 7, 2025
Next-generation artificial intelligence (AI) hardware based on memristive devices offers a promising approach to reducing the increasingly large energy consumption of AI applications. However, programming achieve desired synaptic weight configuration remains challenging because it requires accurate and energy-efficient algorithms for selecting optimal weight-update pulses. Here, we present computationally efficient model predicting update guiding device programming. The behavior bilayer HfO2/TiO2 is characterized over range pulse parameters provide experimental data model. Three different neural network (ANN) configurations are trained evaluated regarding amount training required predictions computational costs. Finally, apply an antipulse process demonstrate its performance. results show that inexpensive possible with comparatively few datasets small ANNs. normalized processes predicted accuracies comparable larger architectures but require only 896 floating point operations 8.33 nJ per inference. This makes candidate integration into AI-driven controllers as precise solution
Language: Английский