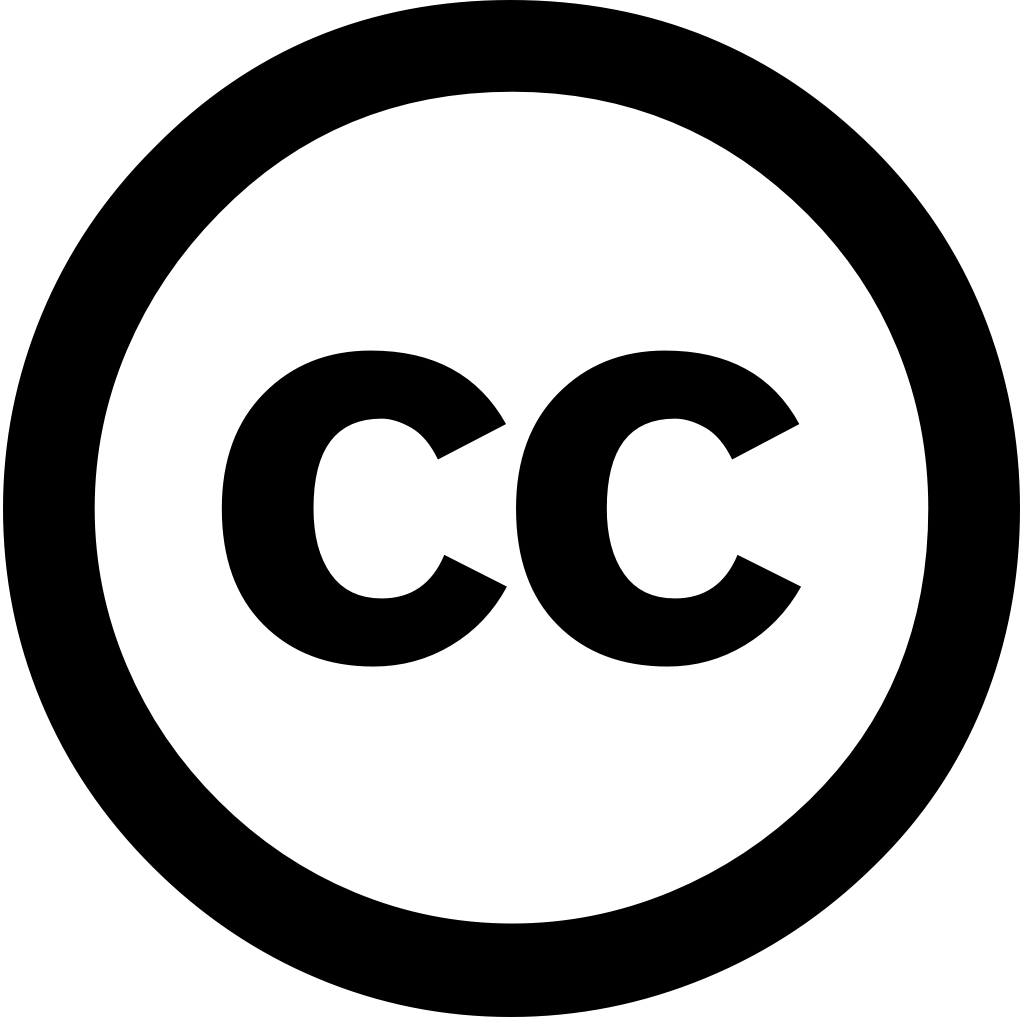
Scientific Reports, Journal Year: 2024, Volume and Issue: 14(1)
Published: Sept. 6, 2024
Language: Английский
Scientific Reports, Journal Year: 2024, Volume and Issue: 14(1)
Published: Sept. 6, 2024
Language: Английский
npj Digital Medicine, Journal Year: 2024, Volume and Issue: 7(1)
Published: Feb. 12, 2024
Digital measures of health status captured during daily life could greatly augment current in-clinic assessments for rheumatoid arthritis (RA), to enable better assessment disease progression and impact. This work presents results from weaRAble-PRO, a 14-day observational study, which aimed investigate how digital technologies (DHT), such as smartphones wearables, patient reported outcomes (PRO) determine RA severity in study 30 moderate-to-severe patients, compared matched healthy controls (HC). Sensor-based status, mobility, dexterity, fatigue, other specific symptoms were extracted iPhone guided tests (GT), well actigraphy heart rate sensor data, was passively recorded patients' Apple smartwatch continuously over the duration. We subsequently developed machine learning (ML) framework distinguish estimate severity. It found that wearable sensor-outcomes robustly distinguished HC participants (F1, 0.807). Furthermore, by day 7 (half-way), sufficient volume data had been collected reliably capture characteristics participants. In addition, we observed detection levels be improved augmenting standard with sensor-based features 0.833) comparison using PRO alone 0.759), combination modalities reliability measure continuous severity, determined clinician-assessed RAPID-3 score at baseline (r
Language: Английский
Citations
23npj Digital Medicine, Journal Year: 2024, Volume and Issue: 7(1)
Published: May 20, 2024
Abstract Sleep is essential to life. Accurate measurement and classification of sleep/wake sleep stages important in clinical studies for disorder diagnoses the interpretation data from consumer devices monitoring physical mental well-being. Existing non-polysomnography techniques mainly rely on heuristic methods developed relatively small cohorts. Thus, we aimed establish accuracy wrist-worn accelerometers stage subsequently describe association between duration efficiency (proportion total time asleep when bed) with mortality outcomes. We a self-supervised deep neural network using concurrent laboratory-based polysomnography accelerometry. After exclusion, 1113 participant nights were used training. The difference model classifications external validation was 48.2 min (95% limits agreement (LoA): −50.3 146.8 min) duration, −17.1 REM LoA: −56.7 91.0 31.1 −67.3 129.5 NREM duration. classifier deployed UK Biobank ~100,000 participants study all-cause mortality. Among 66,262 participants, 1644 events observed. Short sleepers (<6 h) had higher risk compared normal 6–7.9 h, regardless whether they low (Hazard ratios (HRs): 1.36; 95% confidence intervals (CIs): 1.18 1.58) or high (HRs: 1.29; CIs: 1.04–1.61). Deep-learning-based has fair moderate polysomnography. Our findings suggest that having short overnight confers irrespective continuity.
Language: Английский
Citations
9medRxiv (Cold Spring Harbor Laboratory), Journal Year: 2023, Volume and Issue: unknown
Published: Feb. 22, 2023
Step count is an intuitive measure of physical activity frequently quantified in a range health-related studies; however, accurate quantification step can be difficult the free-living environment, with counting error routinely above 20% both consumer and research-grade wrist-worn devices. This study aims to describe development validation derived from accelerometer assess its association cardiovascular all-cause mortality large prospective cohort study.
Language: Английский
Citations
12The Lancet Rheumatology, Journal Year: 2024, Volume and Issue: unknown
Published: July 1, 2024
Language: Английский
Citations
4Expert Systems with Applications, Journal Year: 2025, Volume and Issue: unknown, P. 126638 - 126638
Published: Jan. 1, 2025
Language: Английский
Citations
0Lecture notes in computer science, Journal Year: 2025, Volume and Issue: unknown, P. 82 - 94
Published: Jan. 1, 2025
Language: Английский
Citations
0Lecture notes in computer science, Journal Year: 2025, Volume and Issue: unknown, P. 66 - 81
Published: Jan. 1, 2025
Language: Английский
Citations
0BMC Biomedical Engineering, Journal Year: 2025, Volume and Issue: 7(1)
Published: Jan. 31, 2025
Abstract Aim The aim of this study is to apply a novel hybrid framework incorporating Vision Transformer (ViT) and bidirectional long short-term memory (Bi-LSTM) model for classifying physical activity intensity (PAI) in adults using gravity-based acceleration. Additionally, it further investigates how PAI temporal window (TW) impacts the model’ s accuracy. Method This research used Capture-24 dataset, consisting raw accelerometer data from 151 participants aged 18 91. Gravity-based acceleration was utilised generate images encoding various PAIs. These were subsequently analysed ViT-BiLSTM model, with results presented confusion matrices compared baseline models. model’s robustness evaluated through stability testing examination accuracy loss curves. Result excelled classification task, achieving an overall 98.5% ± 1.48% across five TWs-98.7% 1s, 98.1% 5s, 98.2% 10s, 99% 15s, 98.65% 30s TW. consistently exhibited superior predicting sedentary (98.9% 1%) light (98.2% 2%) moderate-to-vigorous 3%). ANOVA showed no significant variation PAIs (F = 2.18, p 0.13) TW 0.52, 0.72). Accuracy curves show improves its performance epochs, demonstrating excellent robustness. Conclusion demonstrates efficacy acceleration, remaining consistent diverse TWs intensities. However, could result slight variations performance. Future should concern investigate impact on thresholds, which may influence reliability.
Language: Английский
Citations
0IEEE Transactions on Neural Systems and Rehabilitation Engineering, Journal Year: 2025, Volume and Issue: 33, P. 770 - 779
Published: Jan. 1, 2025
Patients with femoral fractures are typically advised to undergo partial weight-bearing (PWB) gait training during the postoperative rehabilitation period facilitate bone healing and restore lower limb function. Various current portable biofeedback devices monitor ground reaction force (GRF) assess loading of patients PWB walking. However, due influence muscle forces complexity load transmission in limbs, GRF may not accurately reflect internal femur In this study, we developed an innovative device that incorporates inertial measurement units pressure-sensitive insoles. Utilizing data collected from 12 participants, a physics-informed temporal convolutional network (PITCN) method was proposed estimate loading. The performance PITCN compared two other machine learning approaches baseline method, demonstrating superior predictive capabilities. study also revealed that, irrespective level walking, peak consistently exceeded GRF. Moreover, timing values for these within each cycle always coincide. These findings further emphasize necessity monitoring providing feedback on actual loading, rather than solely relying GRF, fractures. system is non-invasive, reliable, provides audio feedback. It shows potential as viable solution daily life, contributing enhancement patients' outcomes.
Language: Английский
Citations
0Scientific Reports, Journal Year: 2025, Volume and Issue: 15(1)
Published: Feb. 27, 2025
Abstract Human Activity Recognition (HAR) plays a critical role in fields such as healthcare, sports, and human-computer interaction. However, achieving high accuracy robustness remains challenge, particularly when dealing with noisy sensor data from accelerometers gyroscopes. This paper introduces HARCNN, novel approach leveraging Convolutional Neural Networks (CNNs) to extract hierarchical spatial temporal features raw data, enhancing activity recognition performance. The HARCNN model is designed 10 convolutional blocks, referred “ConvBlk.” Each block integrates layer, ReLU activation function, batch normalization layer. outputs specific blocks “ConvBlk_3 ConvBlk_4,” “ConvBlk_6 ConvBlk_7,” “ConvBlk_9 ConvBlk_10” are fused using depth concatenation approach. concatenated subsequently passed through 2 × max-pooling layer stride of for further processing. proposed framework evaluated accuracy, precision, sensitivity, f-score key metrics, reflecting the model’s ability correctly classify differentiate between human activities. performance compared traditional pre-trained other state-of-the-art techniques. By advanced feature extraction optimized learning strategies, demonstrates its efficacy 97.87%, 99.12%, 96.58%, 98.51% various activities datasets; UCI-HAR, KU-HAR, WISDM, HMDB51, respectively. comparison underscores robustness, highlighting improvements minimizing false positives negatives, which crucial real-world applications where reliable predictions essential. experiments were conducted window sizes (50ms, 100ms, 200ms, 500ms, 1s, 2s). results indicate that method achieves reliability across these different sizes, adapt varying granularities without significant loss method’s effectiveness making it well-suited deployment diverse HAR scenarios. Notably, best obtained size 200ms.
Language: Английский
Citations
0