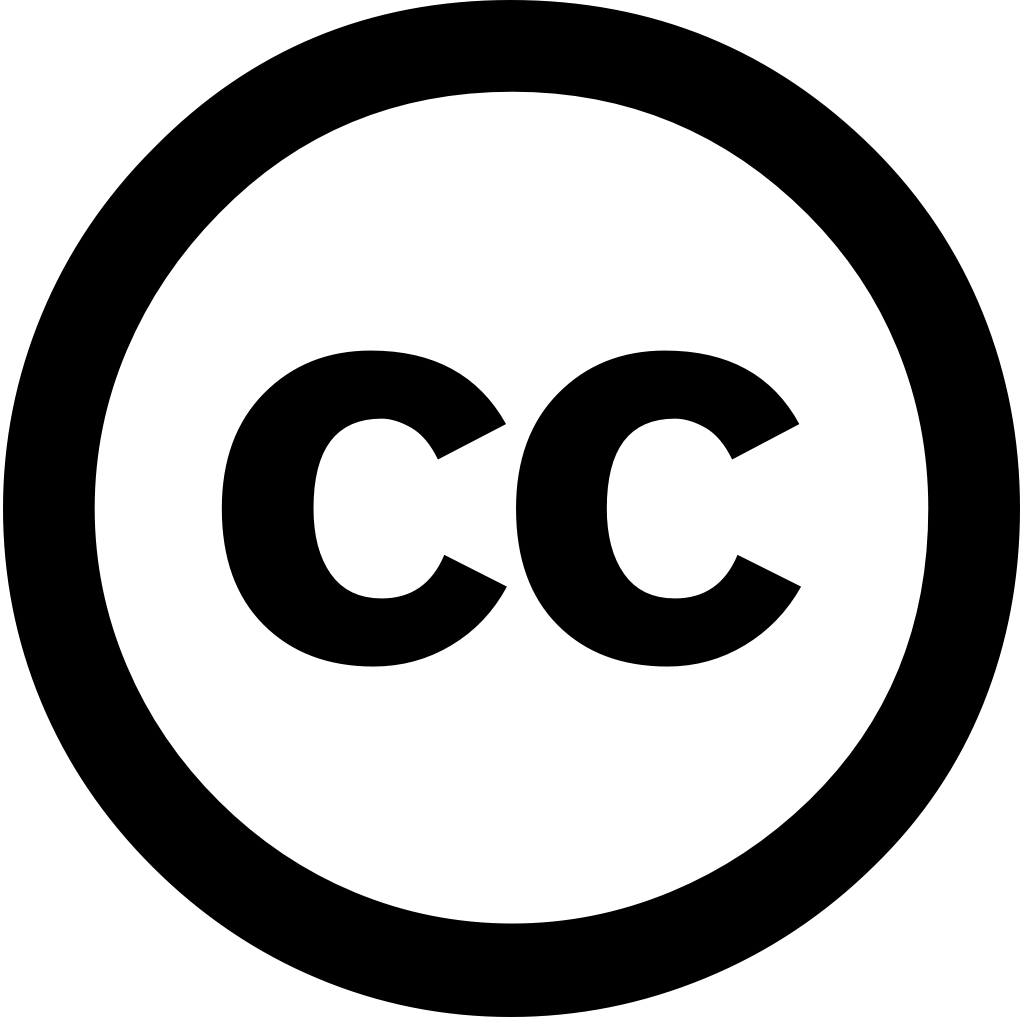
Diagnostics, Journal Year: 2025, Volume and Issue: 15(9), P. 1156 - 1156
Published: May 1, 2025
Background and Objectives: The accurate discrimination between patients with without cancer using their cell-free DNA (cfDNA) is crucial for early diagnosis. end-motifs of cfDNA serve as significant biomarkers, offering compelling prospects This study proposes EM-DeepSD, a signal decomposition deep learning framework based on end-motifs, which aimed at improving the accuracy diagnosis adapting to different sequencing modalities. Materials Methods: included 146 diagnosed 122 non-cancer controls. EM-DeepSD comprises three core modules. Initially, it utilizes module decompose reconstruct input end-motif profiles, thereby generating multiple regular subsequences that optimize subsequent modeling process. Subsequently, both machine are employed improve Furthermore, this paper compares performance existing benchmarked methods demonstrate its superiority. Based framework, we developed EM-DeepSSA model compared two across datasets. Results: In internal validation set, outperformed benchmark (area under curve (AUC), 0.920; adjusted p value < 0.05). Meanwhile, also exhibited best independent external testing sets were subjected 5-hydroxymethylcytosine (5hmCS) broad-range (BR-cfDNA-Seq), respectively (test set-1: AUC = 0.933; test set-2: 0.956; Conclusions: summary, present new can achieve high classification in applicable
Language: Английский