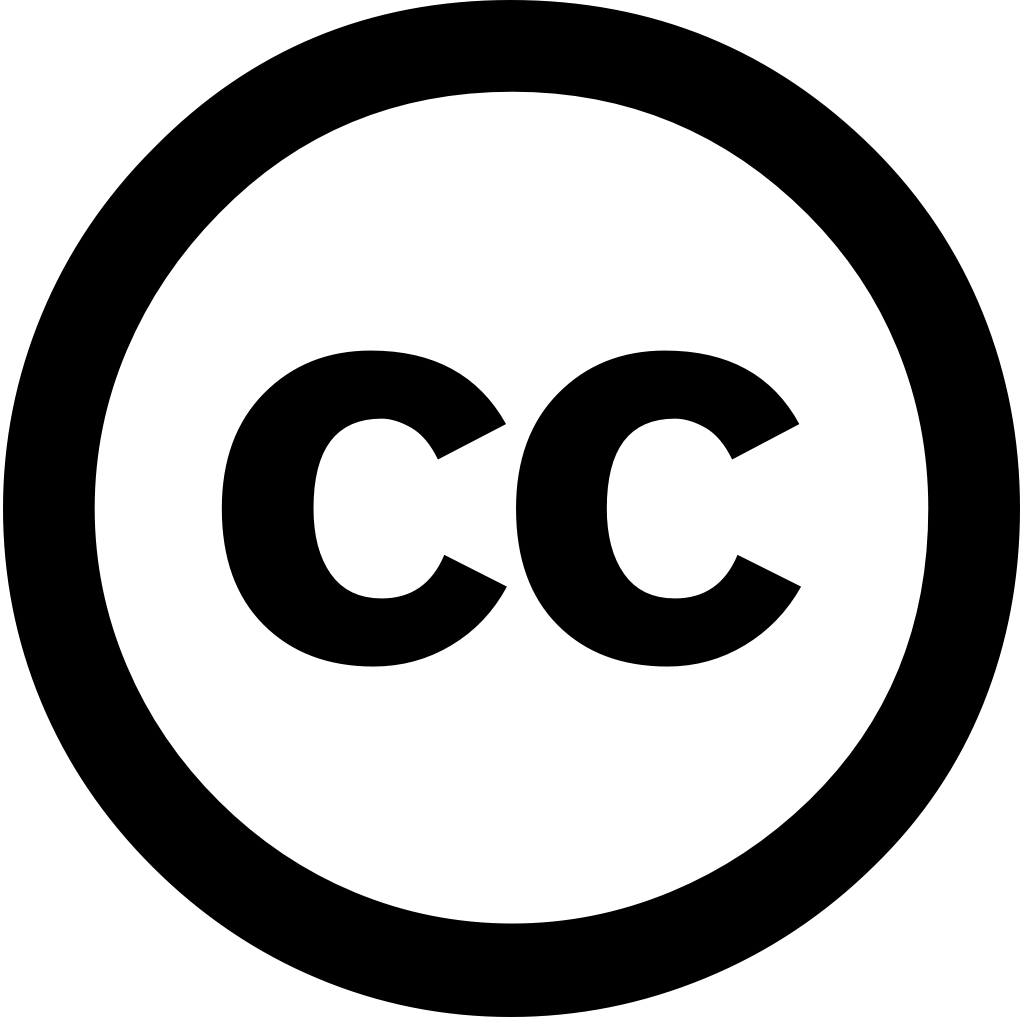
Coordination Chemistry Reviews, Journal Year: 2025, Volume and Issue: 539, P. 216760 - 216760
Published: May 6, 2025
Language: Английский
Coordination Chemistry Reviews, Journal Year: 2025, Volume and Issue: 539, P. 216760 - 216760
Published: May 6, 2025
Language: Английский
Coordination Chemistry Reviews, Journal Year: 2023, Volume and Issue: 484, P. 215112 - 215112
Published: March 21, 2023
The reticular chemistry of metal–organic frameworks (MOFs) allows for the generation an almost boundless number materials some which can be a substitute traditionally used porous in various fields including gas storage and separation, catalysis, drug delivery. MOFs their potential applications are growing so quickly that, when novel synthesized, testing them all possible is not practical. High-throughput computational screening approaches based on molecular simulations have been widely to investigate identify optimal specific application. Despite resources, given enormous MOF material space, identification promising requires more efficient terms time effort. Leveraging data-driven science techniques offer key benefits such as accelerated design discovery pathways via establishment machine learning (ML) models interpretation complex structure-performance relationships that reach beyond expert intuition. In this review, we present scientific breakthroughs propelled modeling discuss state-of-the-art extending from ML algorithms. Finally, provide our perspective opportunities challenges future big discovery.
Language: Английский
Citations
122Journal of the American Chemical Society, Journal Year: 2023, Volume and Issue: 145(5), P. 2958 - 2967
Published: Jan. 27, 2023
Metal-organic frameworks (MOFs) are materials with a high degree of porosity that can be used for many applications. However, the chemical space MOFs is enormous due to large variety possible combinations building blocks and topology. Discovering optimal specific applications requires an efficient accurate search over countless potential candidates. Previous high-throughput screening methods using computational simulations like DFT time-consuming. Such also require 3D atomic structures MOFs, which adds one extra step when evaluating hypothetical MOFs. In this work, we propose structure-agnostic deep learning method based on Transformer model, named as MOFormer, property predictions MOFormer takes text string representation MOF (MOFid) input, thus circumventing need obtaining structure accelerating process. By comparing other descriptors such Stoichiometric-120 revised autocorrelations, demonstrate achieve state-of-the-art prediction accuracy all benchmarks. Furthermore, introduce self-supervised framework pretrains via maximizing cross-correlation between its representations structure-based crystal graph convolutional neural network (CGCNN) >400k publicly available data. Benchmarks show pretraining improves both models various downstream tasks. revealed more data-efficient quantum-chemical than CGCNN training data limited. Overall, provides novel perspective learning.
Language: Английский
Citations
83International Journal of Hydrogen Energy, Journal Year: 2024, Volume and Issue: 67, P. 1270 - 1294
Published: March 27, 2024
The demand for clean and sustainable energy solutions is escalating as the global population grows economies develop. Fossil fuels, which currently dominate sector, contribute to greenhouse gas emissions environmental degradation. In response these challenges, hydrogen storage technologies have emerged a promising avenue achieving sustainability. This review provides an overview of recent advancements in materials technologies, emphasizing importance efficient maximizing hydrogen's potential. highlights physical methods such compressed (reaching pressures up 70 MPa) material-based approaches utilizing metal hydrides carbon-containing substances. It also explores design considerations, computational chemistry, high-throughput screening, machine-learning techniques employed developing materials. comprehensive analysis showcases potential addressing demands, reducing emissions, driving innovation.
Language: Английский
Citations
62Digital Discovery, Journal Year: 2023, Volume and Issue: 2(3), P. 618 - 633
Published: Jan. 1, 2023
Deep graph learning based on electronic structure can contribute to the accelerated discovery of transition metal complexes.
Language: Английский
Citations
25Materials Today Energy, Journal Year: 2023, Volume and Issue: 38, P. 101426 - 101426
Published: Sept. 23, 2023
Hydrogen (H2) is a promising energy carrier for achieving net zero carbon emissions. Metal organic frameworks (MOFs) and covalent (COFs) have emerged as strong alternatives to traditional porous materials highly efficient H2 storage purification applications. With the very rapid continuous increase in number variety of MOFs COFs, early studies this field focused on experimental testing few types randomly selected recently evolved into combining computational screening large material databases with machine learning (ML). In review, we highlighted recent trends merging molecular modeling ML COFs purification. After reviewing high-throughput aiming determine best candidates adsorption separation, discussed that use extracting hidden structure-performance relations from simulation results provide new guidelines inverse design novel MOFs. Finally, addressed current opportunities challenges fusing data science speed development innovative adsorbent membrane respectively.
Language: Английский
Citations
24Journal of the American Chemical Society, Journal Year: 2024, Volume and Issue: 146(9), P. 6134 - 6144
Published: Feb. 26, 2024
In recent data-driven approaches to material discovery, scenarios where target quantities are expensive compute and measure often overlooked. such cases, it becomes imperative construct a training set that includes the most diverse, representative, informative samples. Here, novel regression tree-based active learning algorithm is employed for purpose. It applied predict band gap adsorption properties of metal-organic frameworks (MOFs), class materials results from virtually infinite combinations their building units. Simpler low dimensional descriptors, as those based on stoichiometric geometric properties, used feature space this model owing ability better represent MOFs in data regime. The partitions given by tree constructed labeled part select new samples be added set, thereby limiting its size while maximizing prediction quality. Tests QMOF, hMOF, dMOF sets reveal our method constructs small learn models more efficiently than existing approaches, with lower variance. Specifically, approach highly beneficial when labels unevenly distributed descriptor label distribution imbalanced, which case real world data. regions defined help revealing patterns data, offering unique tool analyze complex structure-property relationships accelerate discovery.
Language: Английский
Citations
13Advanced Materials, Journal Year: 2025, Volume and Issue: unknown
Published: Jan. 7, 2025
Even if MOFs are recently developed for large-scale applications, the road to applications of is long and rocky. This requires overcome challenges associated with phase discovery, synthesis optimization, basic advanced characterization, computational studies. Lab-scale results need be transferred processes, which often not trivial, life-cycle analyses techno-economic performed realistically assess their potential industrial relevance. Based on experience in field stable, functional combining synthesis, modeling, this mini-review gives recommendations especially non-specialists, example, from chemical engineers medical doctors, accelerate facilitate knowledge transfer will ultimately lead application MOFs. The include reporting characterization data as well standardization detailed information required mining machine learning techniques, increasingly used discovery new materials analysis. Once a suitable MOF identified its key properties determined, translational studies shall finally carried out collaboration end-users validate performance under real conditions allow understanding processes involved.
Language: Английский
Citations
1Catalysts, Journal Year: 2023, Volume and Issue: 13(1), P. 154 - 154
Published: Jan. 9, 2023
Nowadays, heterogeneous photocatalysis for water treatment and hydrogen production are topics gaining interest scientists developers from different areas, such as environmental technology material science. Most of the efforts resources devoted to development new photocatalyst materials, while modeling reaction systems allowing upscaling process pilot or industrial scale scarce. In this work, we present what is known on purify produce green H2. The types reactors successfully used in plants presented study cases. challenges H2 explored perspectives (a) adaptation photoreactors, (b) competitiveness process, (c) safety. Throughout text, Green Chemistry Engineering Principles described discussed how they currently being applied along with that ahead. Lastly, role automation high-throughput methods following discussed.
Language: Английский
Citations
17Langmuir, Journal Year: 2023, Volume and Issue: 39(45), P. 15849 - 15863
Published: Nov. 3, 2023
Metal–organic frameworks (MOFs) have attracted tremendous interest because of their tunable structures, functionalities, and physiochemical properties. The nearly infinite combinations metal nodes organic linkers led to the synthesis over 100,000 experimental MOFs construction millions hypothetical counterparts. It is intractable identify best candidates in immense chemical space for applications via conventional trial-to-error experiments or brute-force simulations. Over past several years, machine learning (ML) has substantially transformed way MOF discovery, design, synthesis. Driven by abundant data from simulations, ML can not only efficiently accurately predict properties but also quantitatively derive structure–property relationships rational design screening. In this Perspective, we summarize recent achievements leveraging aspects acquisition, featurization, model training, applications. Then, current challenges new opportunities are discussed future exploration accelerate development vibrant field.
Language: Английский
Citations
17Advanced Science, Journal Year: 2022, Volume and Issue: 9(36)
Published: Oct. 26, 2022
Abstract Addressing climate change challenges by reducing greenhouse gas levels requires innovative adsorbent materials for clean energy applications. Recent progress in machine learning has stimulated technological breakthroughs the discovery, design, and deployment of with potential high‐performance low‐cost This review summarizes basic methods—data collection, featurization, model generation, evaluation—and reviews their use development robust materials. Key case studies are provided where these methods used to accelerate design optimize synthesis conditions, understand complex feature–property relationships. The provides a concise resource researchers wishing rapidly develop effective positive impact on environment.
Language: Английский
Citations
26