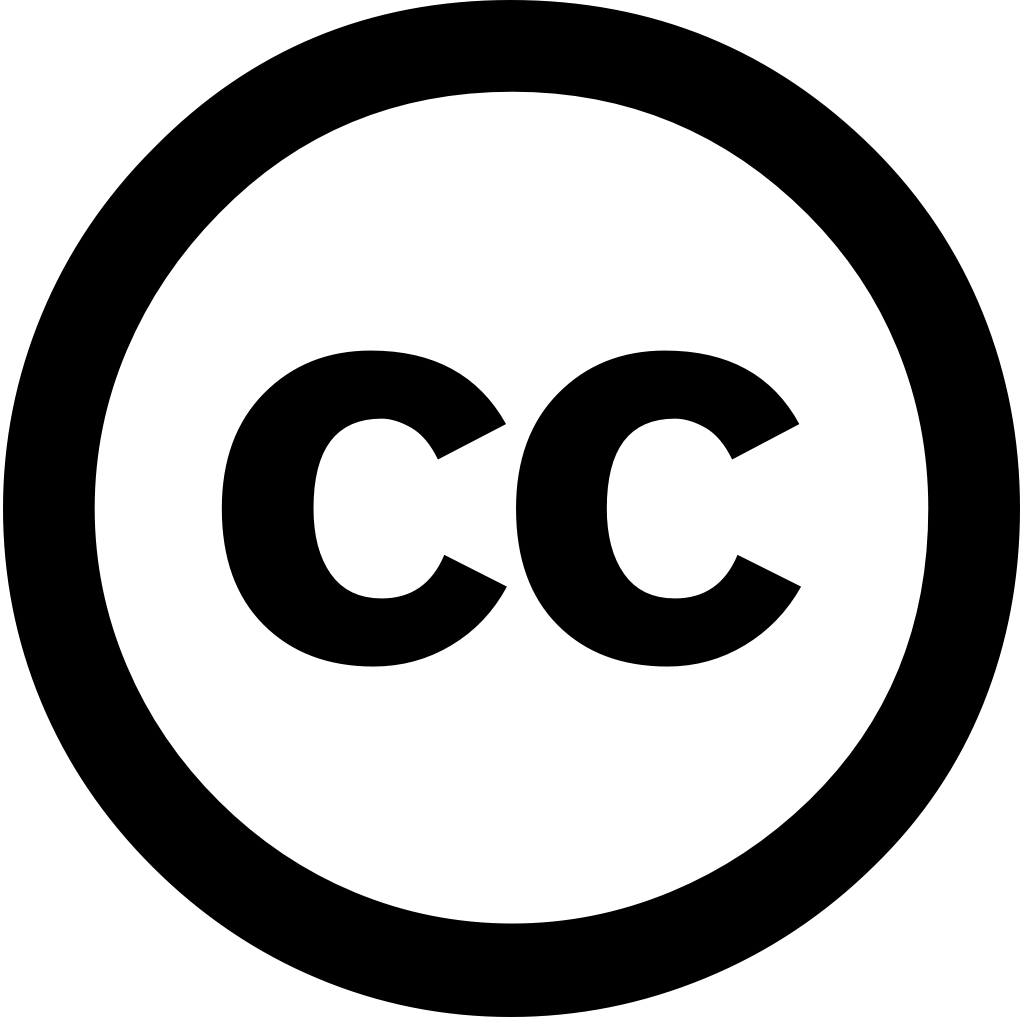
npj Computational Materials, Journal Year: 2024, Volume and Issue: 10(1)
Published: Dec. 19, 2024
Language: Английский
npj Computational Materials, Journal Year: 2024, Volume and Issue: 10(1)
Published: Dec. 19, 2024
Language: Английский
Proceedings of the National Academy of Sciences, Journal Year: 2024, Volume and Issue: 121(23)
Published: May 29, 2024
While RNA appears as a good candidate for the first autocatalytic systems preceding emergence of modern life, synthesis oligonucleotides without enzymes remains challenging. Because uncatalyzed reaction is extremely slow, experimental studies bring limited and indirect information on mechanism, nature which debated. Here, we develop neural network potentials (NNPs) to study phosphoester bond formation in water. NNPs are becoming routinely applied nonreactive or simple reactions, demonstrate how they can systematically be trained explore phase space complex reactions involving several proton transfers exchanges heavy atoms. We then propagate at moderate computational cost hundreds nanoseconds variety enhanced sampling simulations with quantum accuracy explicit solvent conditions. The thermodynamically preferred pathway concerted, dissociative transient metaphosphate transition state direct participation water molecules that facilitate exchange protons through nonbridging phosphate oxygens. Associative-dissociative pathways, characterized by much tighter pentacoordinated phosphate, higher free energy. Our also suggest diprotonated whose reactivity never directly assessed experiments, significantly less reactive than monoprotonated species, suggesting it probably species normal pH These observations rationalize unexplained results temperature dependence rate, pave way design more efficient abiotic catalysts activating groups.
Language: Английский
Citations
11ACS Catalysis, Journal Year: 2025, Volume and Issue: unknown, P. 2607 - 2619
Published: Jan. 28, 2025
Language: Английский
Citations
1Journal of Chemical Theory and Computation, Journal Year: 2025, Volume and Issue: unknown
Published: Jan. 14, 2025
Training accurate machine learning potentials requires electronic structure data comprehensively covering the configurational space of system interest. As construction this is computationally demanding, many schemes for identifying most important structures have been proposed. Here, we compare performance high-dimensional neural network (HDNNPs) quantum liquid water at ambient conditions trained to sets constructed using random sampling as well various flavors active based on query by committee. Contrary common understanding learning, find that a given set size, leads smaller test errors not included in training process. In our analysis, show can be related small energy offsets caused bias added which overcome instead correlations an error measure invariant such shifts. Still, all HDNNPs yield very similar and structural properties water, demonstrates robustness procedure with respect algorithm even when few 200 structures. However, preliminary potentials, reasonable initial avoid unnecessary extension covered configuration less relevant regions.
Language: Английский
Citations
0Physical Chemistry Chemical Physics, Journal Year: 2025, Volume and Issue: unknown
Published: Jan. 1, 2025
Stability constants of simple reactions involving addition the NO 3 − ion to hydrated metal complexes, [M(H 2 O) x ] n + are calculated with a computational workflow developed using cloud computing resources.
Language: Английский
Citations
0Journal of Chemical Theory and Computation, Journal Year: 2025, Volume and Issue: unknown
Published: March 2, 2025
Machine learning (ML) methods have emerged as an efficient surrogate for high-level electronic structure theory, offering precision and computational efficiency. However, the vast conformational chemical space remains challenging when constructing a general force field. Training data sets typically cover only limited region of this space, resulting in poor extrapolation performance. Traditional strategies must address problem by training models from scratch using old new sets. In addition, model transferability is crucial field construction. Existing ML fields, designed closed systems with no external environmental potential, exhibit to complex condensed phase such enzymatic reactions, inferior performance high memory costs. Our ML/MM model, based on Taylor expansion electrostatic operator, showed between reactions several simple solvents. This work extends strategy explore more heterogeneous environments. we also apply continual enable autonomous on-the-fly continuous stream data. By combining these two methods, can efficiently construct that be applied various media.
Language: Английский
Citations
0Computer Physics Communications, Journal Year: 2024, Volume and Issue: unknown, P. 109446 - 109446
Published: Nov. 1, 2024
Language: Английский
Citations
2npj Computational Materials, Journal Year: 2024, Volume and Issue: 10(1)
Published: Dec. 19, 2024
Language: Английский
Citations
1