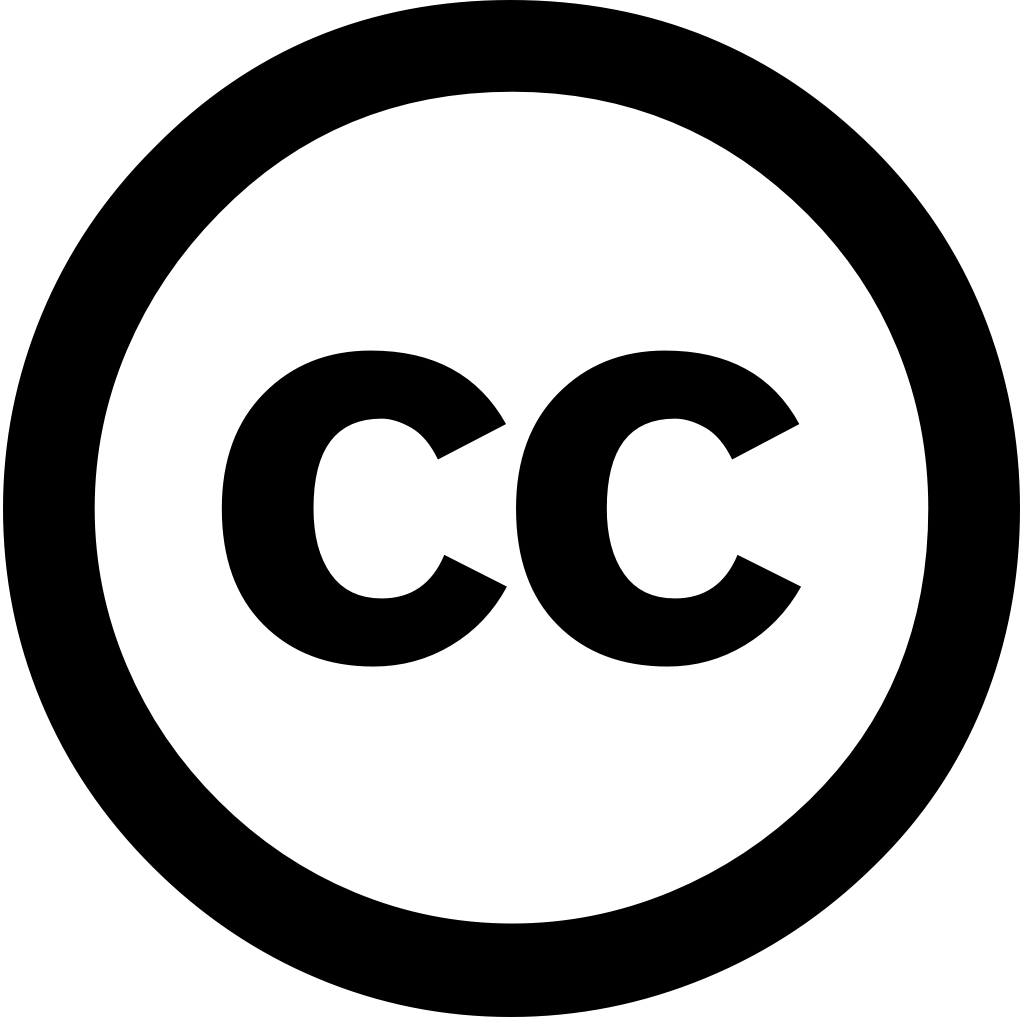
Journal of Technology in Behavioral Science, Journal Year: 2024, Volume and Issue: unknown
Published: Nov. 28, 2024
Abstract This systematic literature review explores the emerging field of remote-based deep learning predictive algorithms for depression, focusing on addressing limitations traditional diagnostic methods and examining current state research in this novel area. A search was conducted Embase, Medline, Web Science Core Collection, CINAHL, PsycINFO June 2023. To capture relevant studies, titles abstracts papers were reviewed against predefined inclusion exclusion criteria using four groups keywords prediction, validity, learning. Eligible studies systematically based Critical Appraisal Data Extraction Systematic Reviews Prediction Modelling Studies (CHARMS) checklist. The risk bias assessed Model Risk Bias Assessment (PROBAST) Tool methodological quality. synthesis data Synthesis Without Meta-Analysis (SWiM) framework. From 286 initially identified, 6 met all criteria, published between 2020 Performance metrics revealed potential models, with accuracy rates reaching as high 98.23%. Convolutional neural networks (CNNs) emerged predominant model, applicability across diverse sources such speech recordings, body motion data, facial images. However, issues related to prevalent, most lacking essential reporting details employing relatively small sample sizes. identified practical application these including limited demographic representation, absence external validation, a notable models capable anticipating onset depression. While focus primarily identifying existing depression any duration, there is need advancements that enable anticipation future depressive episodes. advance field, we recommend standardized practices, larger more datasets, development anticipate occurrences advance. These enhancements will contribute credibility real-world relevance models. hold promise revolutionizing they require refinement validation fulfil their clinical practice. underscores further area address improved mental health assessment intervention.
Language: Английский