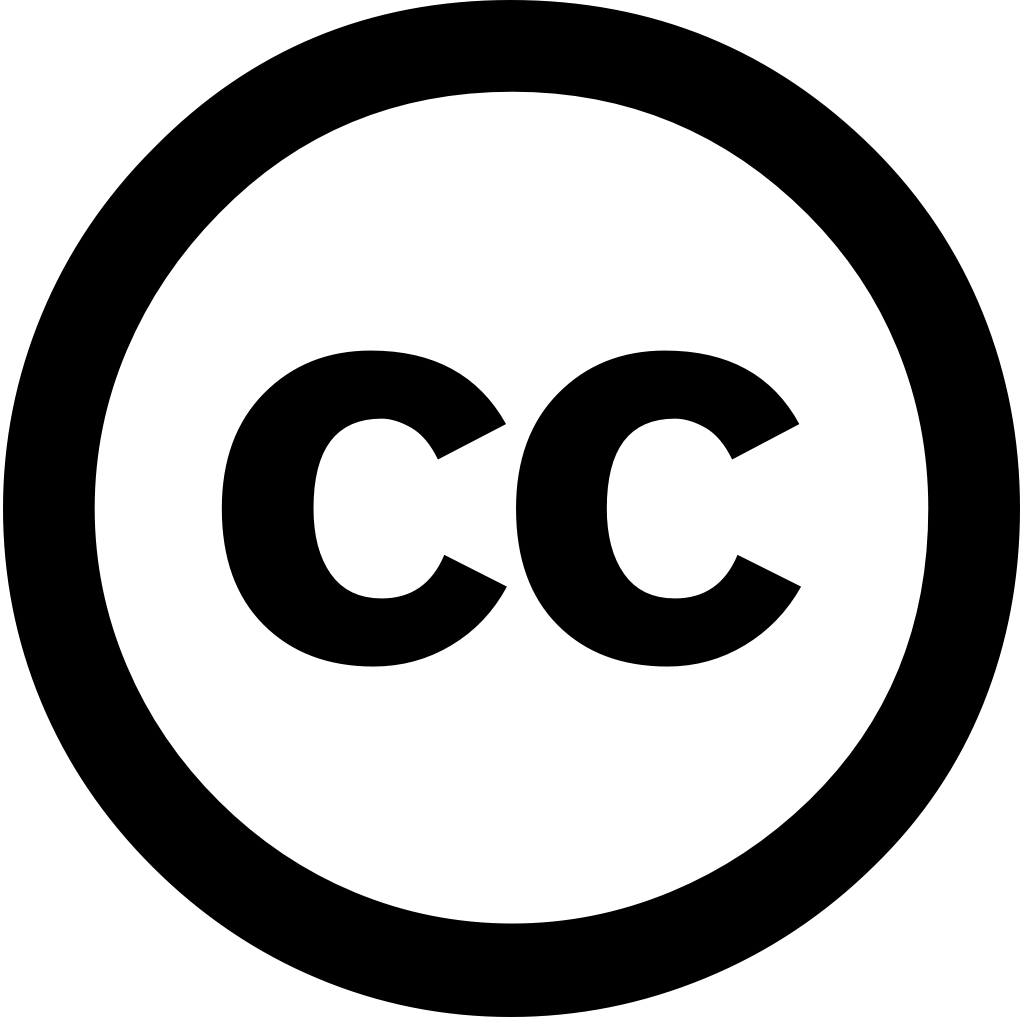
npj Computational Materials, Journal Year: 2025, Volume and Issue: 11(1)
Published: April 2, 2025
Language: Английский
npj Computational Materials, Journal Year: 2025, Volume and Issue: 11(1)
Published: April 2, 2025
Language: Английский
The Innovation, Journal Year: 2021, Volume and Issue: 2(4), P. 100179 - 100179
Published: Oct. 29, 2021
•"Can machines think?" The goal of artificial intelligence (AI) is to enable mimic human thoughts and behaviors, including learning, reasoning, predicting, so on.•"Can AI do fundamental research?" coupled with machine learning techniques impacting a wide range sciences, mathematics, medical science, physics, etc.•"How does accelerate New research applications are emerging rapidly the support by infrastructure, data storage, computing power, algorithms, frameworks. Artificial promising (ML) well known from computer science broadly affecting many aspects various fields technology, industry, even our day-to-day life. ML have been developed analyze high-throughput view obtaining useful insights, categorizing, making evidence-based decisions in novel ways, which will promote growth fuel sustainable booming AI. This paper undertakes comprehensive survey on development application different information materials geoscience, life chemistry. challenges that each discipline meets, potentials handle these challenges, discussed detail. Moreover, we shed light new trends entailing integration into scientific discipline. aim this provide broad guideline sciences potential infusion AI, help motivate researchers deeply understand state-of-the-art AI-based thereby continuous sciences.
Language: Английский
Citations
943Nature Communications, Journal Year: 2022, Volume and Issue: 13(1)
Published: Feb. 21, 2022
The rational design of molecules with desired properties is a long-standing challenge in chemistry. Generative neural networks have emerged as powerful approach to sample novel from learned distribution. Here, we propose conditional generative network for 3d molecular structures specified chemical and structural properties. This agnostic bonding enables targeted sampling distributions, even domains where reference calculations are sparse. We demonstrate the utility our method inverse by generating motifs or composition, discovering particularly stable molecules, jointly targeting multiple electronic beyond training regime.
Language: Английский
Citations
127The Journal of Chemical Physics, Journal Year: 2023, Volume and Issue: 158(12)
Published: March 2, 2023
Machine learning (ML) approaches enable large-scale atomistic simulations with near-quantum-mechanical accuracy. With the growing availability of these methods, there arises a need for careful validation, particularly physically agnostic models-that is, potentials that extract nature atomic interactions from reference data. Here, we review basic principles behind ML and their validation atomic-scale material modeling. We discuss best practice in defining error metrics based on numerical performance, as well guided validation. give specific recommendations hope will be useful wider community, including those researchers who intend to use materials "off shelf."
Language: Английский
Citations
68Nature Computational Science, Journal Year: 2023, Volume and Issue: 3(3), P. 198 - 209
Published: March 31, 2023
Language: Английский
Citations
48The Journal of Chemical Physics, Journal Year: 2023, Volume and Issue: 158(14)
Published: March 21, 2023
SchNetPack is a versatile neural network toolbox that addresses both the requirements of method development and application atomistic machine learning. Version 2.0 comes with an improved data pipeline, modules for equivariant networks, PyTorch implementation molecular dynamics. An optional integration Lightning Hydra configuration framework powers flexible command-line interface. This makes easily extendable custom code ready complex training tasks, such as generation 3D structures.
Language: Английский
Citations
45Nature Reviews Materials, Journal Year: 2023, Volume and Issue: 8(5), P. 309 - 313
Published: March 13, 2023
Language: Английский
Citations
43Energy storage materials, Journal Year: 2023, Volume and Issue: 59, P. 102785 - 102785
Published: April 23, 2023
Language: Английский
Citations
43Advanced Functional Materials, Journal Year: 2024, Volume and Issue: unknown
Published: April 8, 2024
Abstract Electrocatalytic nitrate reduction reaction (NO 3 RR) driven by renewable energy is a promising technology for the removal of nitrate‐containing wastewater. However, sluggish kinetics resulted from complex proton‐coupled electron transfer and various intermediates remain key barriers large‐scale application NO RR. Herein, tactic reported to raise rate RR increase selectivity N 2 using bimetal catalyst: Co inclined act on steps needed in process, rate‐determining step (RDS: *NO , asterisk means intermediates) subsequent *N hydrogenation as well Fe exhibits efficient activity selectivity‐ determining (SDS: then ) via relay catalysis mechanism. A efficiency 78.5% an ultra‐long cycle stability 60 cycles (12 h per cycle) are achieved FeCo alloy confined with nitrogen‐doped porous carbon nanofibers (FeCo‐NPCNFs). DFT calculations unveil that introduction active site not only regulates d‐band center alloy, optimizes adsorption intermediates, but also has strong capacity supply hydrogen species. Clearly, this study elucidates effects bimetallic performance electrocatalytic offers avenues designing Fe‐based catalysts realize nitrogen‐neutral cycle.
Language: Английский
Citations
43Artificial Intelligence Chemistry, Journal Year: 2024, Volume and Issue: 2(1), P. 100049 - 100049
Published: Jan. 19, 2024
Artificial intelligence (AI) is driving a revolution in chemistry, reshaping the landscape of molecular design. This review explores AI's pivotal roles field organic synthesis applications. AI accurately predicts reaction outcomes, controls chemical selectivity, simplifies planning, accelerates catalyst discovery, and fuels material innovation so on. It seamlessly integrates data-driven algorithms with intuition to redefine As chemistry advances, it promises accelerated research, sustainability, innovative solutions chemistry's pressing challenges. The fusion poised shape field's future profoundly, offering new horizons precision efficiency. encapsulates transformation marking moment where data converge revolutionize world molecules.
Language: Английский
Citations
25Journal of Chemical Information and Modeling, Journal Year: 2024, Volume and Issue: 64(3), P. 799 - 811
Published: Jan. 18, 2024
The pursuit of designing smart and functional materials is paramount importance across various domains, such as material science, engineering, chemical technology, electronics, biomedicine, energy, numerous others. Consequently, researchers are actively involved in the development innovative models strategies for design. Recent advancements analytical tools, experimentation, computer technology additionally enhance design possibilities. Notably, data-driven techniques like artificial intelligence machine learning have achieved substantial progress exploring applications within science. One approach, ChatGPT, a large language model, holds transformative potential addressing complex queries. In this article, we explore ChatGPT's understanding science by assigning some simple tasks subareas computational findings indicate that while ChatGPT may make minor errors accomplishing general tasks, it demonstrates capability to learn adapt through human interactions. However, issues output consistency, probable hidden errors, ethical consequences should be addressed.
Language: Английский
Citations
19