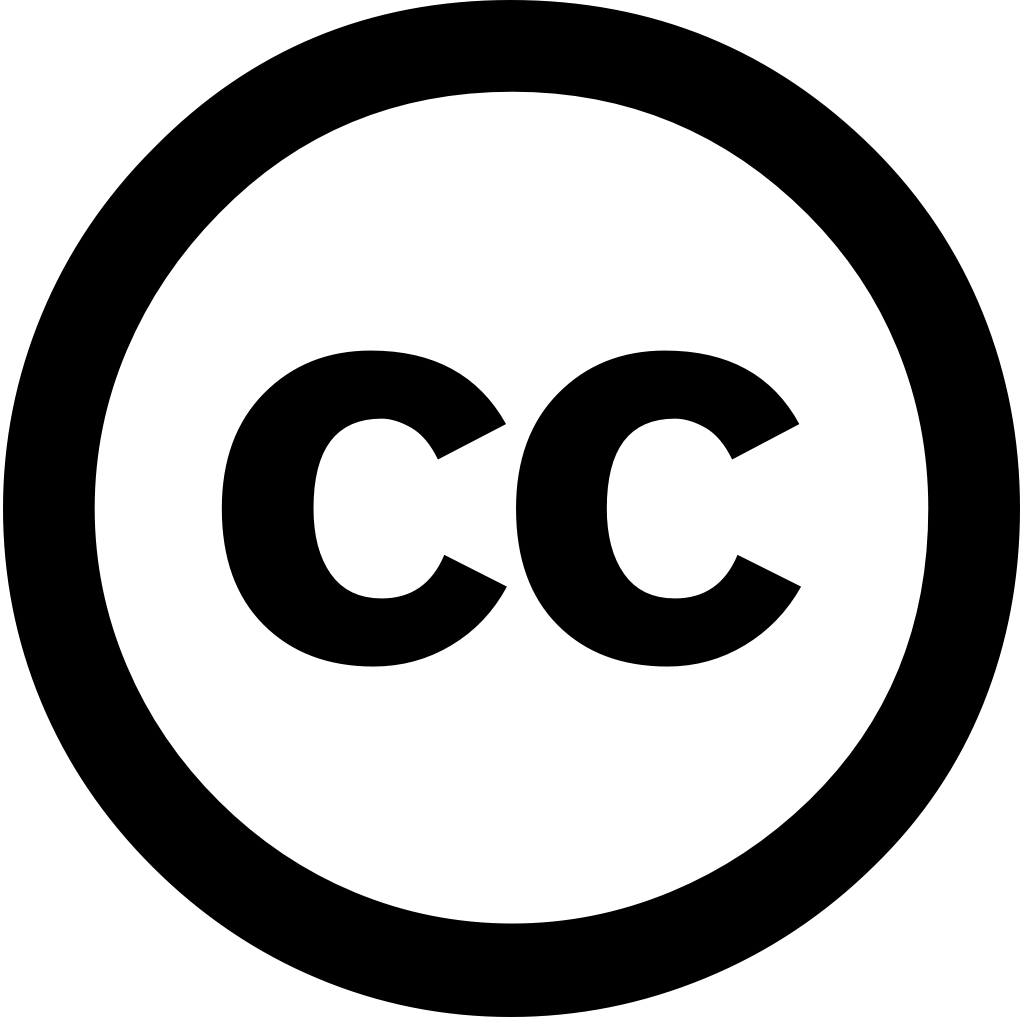
Computer Physics Communications, Journal Year: 2024, Volume and Issue: unknown, P. 109446 - 109446
Published: Nov. 1, 2024
Language: Английский
Computer Physics Communications, Journal Year: 2024, Volume and Issue: unknown, P. 109446 - 109446
Published: Nov. 1, 2024
Language: Английский
Chemical Reviews, Journal Year: 2025, Volume and Issue: unknown
Published: March 10, 2025
In this field guide, we outline empirical and theory-based approaches to characterize the fundamental properties of liquid multivalent-ion battery electrolytes, including (i) structure chemistry, (ii) transport, (iii) electrochemical properties. When detailed molecular-scale understanding multivalent electrolyte behavior is insufficient use examples from well-studied lithium-ion electrolytes. recognition that coupling techniques highly effective, but often nontrivial, also highlight recent characterization efforts uncover a more comprehensive nuanced underlying structures, processes, reactions drive performance system-level behavior. We hope insights these discussions will guide design future studies, accelerate development next-generation batteries through modeling with experiments, help avoid pitfalls ensure reproducibility results.
Language: Английский
Citations
1Computer Physics Communications, Journal Year: 2025, Volume and Issue: unknown, P. 109512 - 109512
Published: Jan. 1, 2025
Language: Английский
Citations
0The Journal of Physical Chemistry Letters, Journal Year: 2025, Volume and Issue: unknown, P. 4626 - 4634
Published: May 1, 2025
Predicting the binding affinity between small molecules and target macromolecules while combining both speed accuracy is a cornerstone of modern computational drug discovery, which critical for accelerating therapeutic development. Despite recent progress in molecular dynamics (MD) simulations, such as advanced polarizable force fields enhanced sampling techniques, estimating absolute free energies (ABFEs) remains computationally challenging. To overcome these difficulties, we introduce highly efficient hybrid methodology that couples Lambda-adaptive biasing (Lambda-ABF) scheme with on-the-fly probability (OPES). This approach achieves up to 9-fold improvement efficiency compared original Lambda-ABF when used conjunction AMOEBA field, yielding converged results at fraction cost standard techniques.
Language: Английский
Citations
0Chemical Physics Reviews, Journal Year: 2025, Volume and Issue: 6(1)
Published: March 1, 2025
Surfaces and interfaces play key roles in chemical material science. Understanding physical processes at complex surfaces is a challenging task. Machine learning provides powerful tool to help analyze accelerate simulations. This comprehensive review affords an overview of the applications machine study systems materials. We categorize into following broad categories: solid–solid interface, solid–liquid liquid–liquid surface solid, liquid, three-phase interfaces. High-throughput screening, combined first-principles calculations, force field accelerated molecular dynamics simulations are used rational design such as all-solid-state batteries, solar cells, heterogeneous catalysis. detailed information on for
Language: Английский
Citations
0Computer Physics Communications, Journal Year: 2024, Volume and Issue: unknown, P. 109446 - 109446
Published: Nov. 1, 2024
Language: Английский
Citations
2