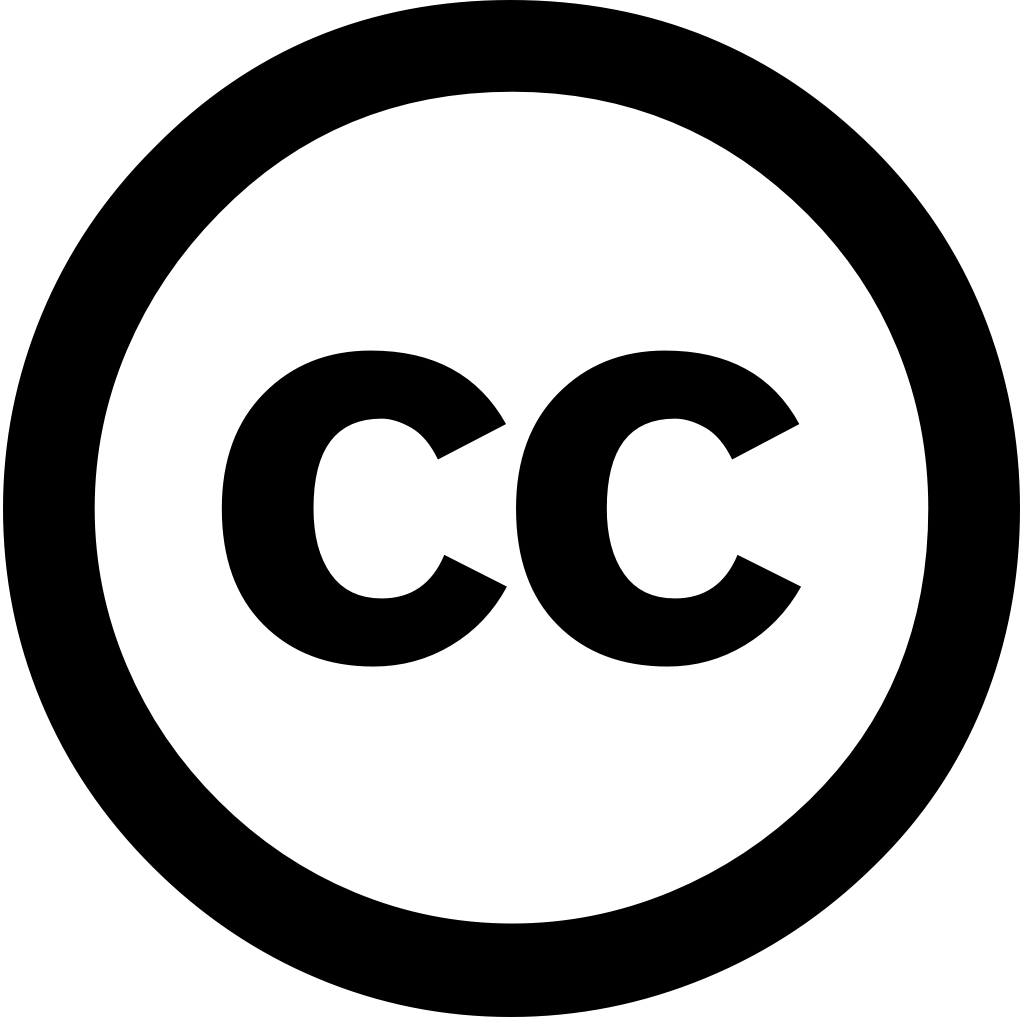
Journal of Chemical Theory and Computation, Journal Year: 2025, Volume and Issue: unknown
Published: April 24, 2025
Machine learning interatomic potentials (MLIPs) offer a promising alternative to traditional force fields and ab initio methods for simulating complex materials such as oxide glasses. In this work, we present the first evaluation of pretrained MACE (Multi-ACE) model [D.P. Kovács et al., J. Chem. Phys. 159(2023), 044118] silicate glasses, using sodium silicates test case. We compare its performance with DeePMD-based MLIP specifically trained on compositions [M. Bertani Theory Comput. 20(2024), 1358-1370] assess their accuracy in reproducing structural dynamical properties. Additionally, investigate role dispersion interactions by incorporating D3(BJ) correction both models. Our results show that while accurately reproduces neutron structure factors, pair distribution functions, Si[Qn] speciation, it performs slightly worst elastic properties calculations. However, is suitable simulations The inclusion significantly improves reproduction density MLIPs, highlighting critical glass modeling. These findings provide insight into transferability general MLIPs disordered systems emphasize need dispersion-aware training data sets developing accurate
Language: Английский