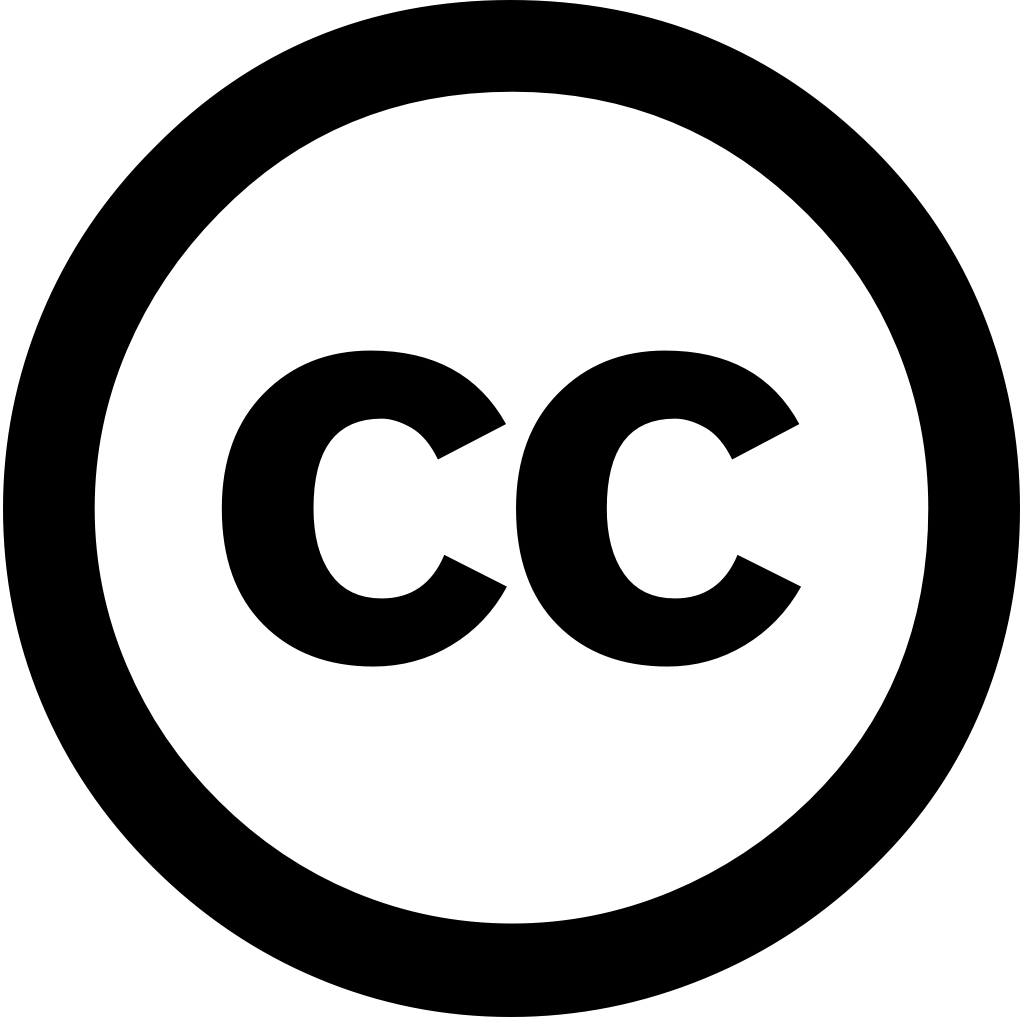
bioRxiv (Cold Spring Harbor Laboratory), Journal Year: 2025, Volume and Issue: unknown
Published: April 16, 2025
Abstract Breast carcinoma remains the most commonly diagnosed malignancy and a leading cause of cancer-related mortality among women worldwide. While mammography is gold standard for early detection, challenges such as high breast density often obscure malignancies, reducing diagnostic sensitivity. Conventional parenchymal texture analysis methods have limitations due to struggles with spatial interpretation noise This study investigates efficacy fractal-based global features distinguishing between malignant normal mammograms assessing their potential molecular subtype differentiation. Digital were analyzed using standardized preprocessing techniques, fractal measures computed capture complexity connectivity properties within tissue structures. Fractal dimension, multifractality strength, succolarity reservoir found effectively characterize specific mammographic texture; however, incorporation into machine learning models yielded moderate discriminatory performance categories. We introduced novel parameter accounting tissues’ latent connectivity. In addition, while exhibits differentiating Luminal B from other subtypes, its overall discriminative power limited. proof-of-concept underscores non-invasive biomarker in diagnosis.
Language: Английский